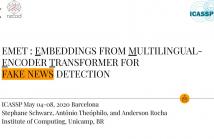
- Read more about EMET : EMBEDDINGS FROM MULTILINGUAL- ENCODER TRANSFORMER FOR FAKE NEWS DETECTION
- Log in to post comments
In the last few years, social media networks have changed human life experience and behavior as it has broken down communication barriers, allowing ordinary people to actively produce multimedia content on a massive scale. On this wise, the information dissemination in social media platforms becomes increasingly common. However, misinformation is propagated with the same facility and velocity as real news, though it can result in irreversible damage to an individual or society at large.
ICASSP.pdf

- Categories:
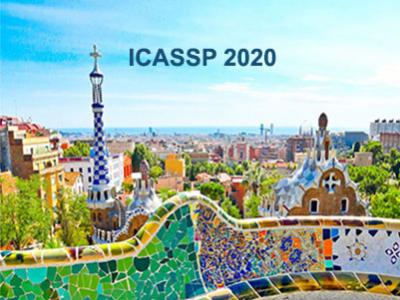
- Read more about Multi-Patch Aggregation Models for Resampling Detection
- 1 comment
- Log in to post comments
Images captured nowadays are of varying dimensions with smartphones and DSLR’s allowing users to choose from a list of available image resolutions. It is therefore imperative for forensic algorithms such as resampling detection to scale well for images of varying dimensions. However, in our experiments we observed that many state-of-the-art forensic algorithms are sensitive to image size and their performance quickly degenerates when operated on images of diverse dimensions despite re-training them using multiple image sizes.
- Categories:
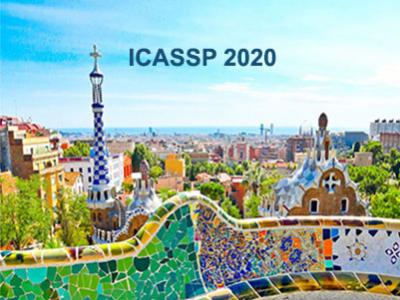
- Read more about Augmentation Data Synthesis via GANs: Boosting Latent Fingerprint Reconstruction
- Log in to post comments
Latent fingerprint reconstruction is a vital preprocessing step for its identification. This task is very challenging due to not only existing complicated degradation patterns but also its scarcity of paired training data. To address these challenges, we propose a novel generative adversarial network (GAN) based data augmentation scheme to improve such reconstruction.
ICASSP1263.pdf

- Categories:
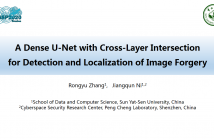
- Read more about A DENSE U-NET WITH CROSS LAYER INTERSECTION FOR DETECTION AND LOCALIZATION OF IMAGE FORGERY
- Log in to post comments
In this paper, we apply cross-layer intersection mechanism to dense u-net for image forgery detection and localization. We first train DenseNet for binary classification. Spatial rich model (SRM) filters are adopted for capturing residual signals in the detected images. Then we propose a new approach to preserve complete feature maps of fully connected layer and consider them as the spatial decision information for image segmentation.
- Categories:
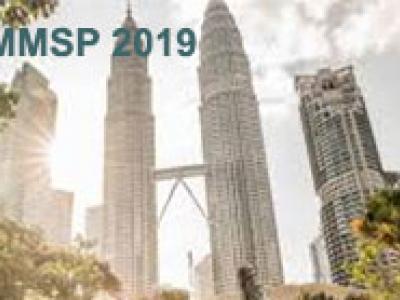
- Read more about Luminance-based Video Backdoor Attack Against Anti-spoofing Rebroadcast Detection
- 1 comment
- Log in to post comments
MMSP2019.pdf

- Categories:
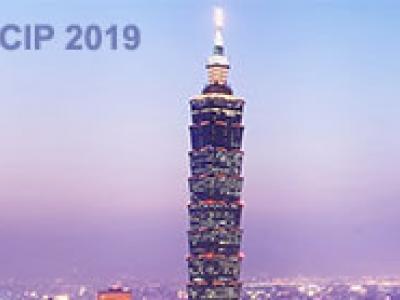
- Read more about A new Backdoor Attack in CNNs by training set corruption without label poisoning
- Log in to post comments
Backdoor attacks against CNNs represent a new threat against deep learning systems, due to the possibility of corrupting the training set so to induce an incorrect behaviour at test time. To avoid that the trainer recognises the presence of the corrupted samples, the corruption of the training set must be as stealthy as possible. Previous works have focused on the stealthiness of the perturbation injected into the training samples, however they all assume that the labels of the corrupted samples are also poisoned.
ICIP2019.pdf

- Categories:
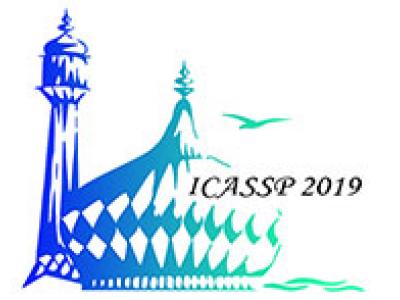
- Read more about Sentiment Aware Fake News Detection on Online Social Networks
- Log in to post comments
- Categories:
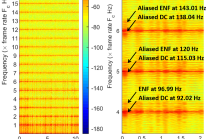
- Read more about ENF Signal Extraction for Rolling-shutter Videos Using Periodic Zero-padding
- Log in to post comments
Electric Network Frequency (ENF) analysis is a promising forensic technique for authenticating digital recordings and detecting tampering within the recordings. The validity of ENF analysis heavily relies on high-quality ENF signals extracted from multimedia recordings. In this paper, we propose an ENF signal extraction method for rolling shutter acquired videos using periodic zero-padding. Our analysis shows that the extracted ENF signals using the proposed method are not distorted and the component with the highest signal-to-noise ratio is located at the intrinsic frequency.
- Categories:
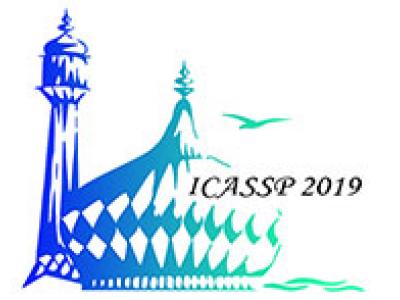
ENF (Electric Network Frequency) oscillates around a nominal value (50/60 Hz) due to imbalance between consumed and generated power. The intensity of a light source powered by mains electricity varies depending on the ENF fluctuations. These fluctuations can be extracted from videos recorded in the presence of mains-powered source illumination. This work investigates how the quality of the ENF signal estimated from video is affected by different light source illumination, compression ratios, and by social media encoding.
- Categories:
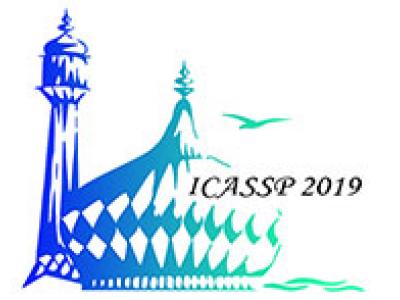
- Read more about Towards learned color representations for image splicing detection
- Log in to post comments
- Categories: