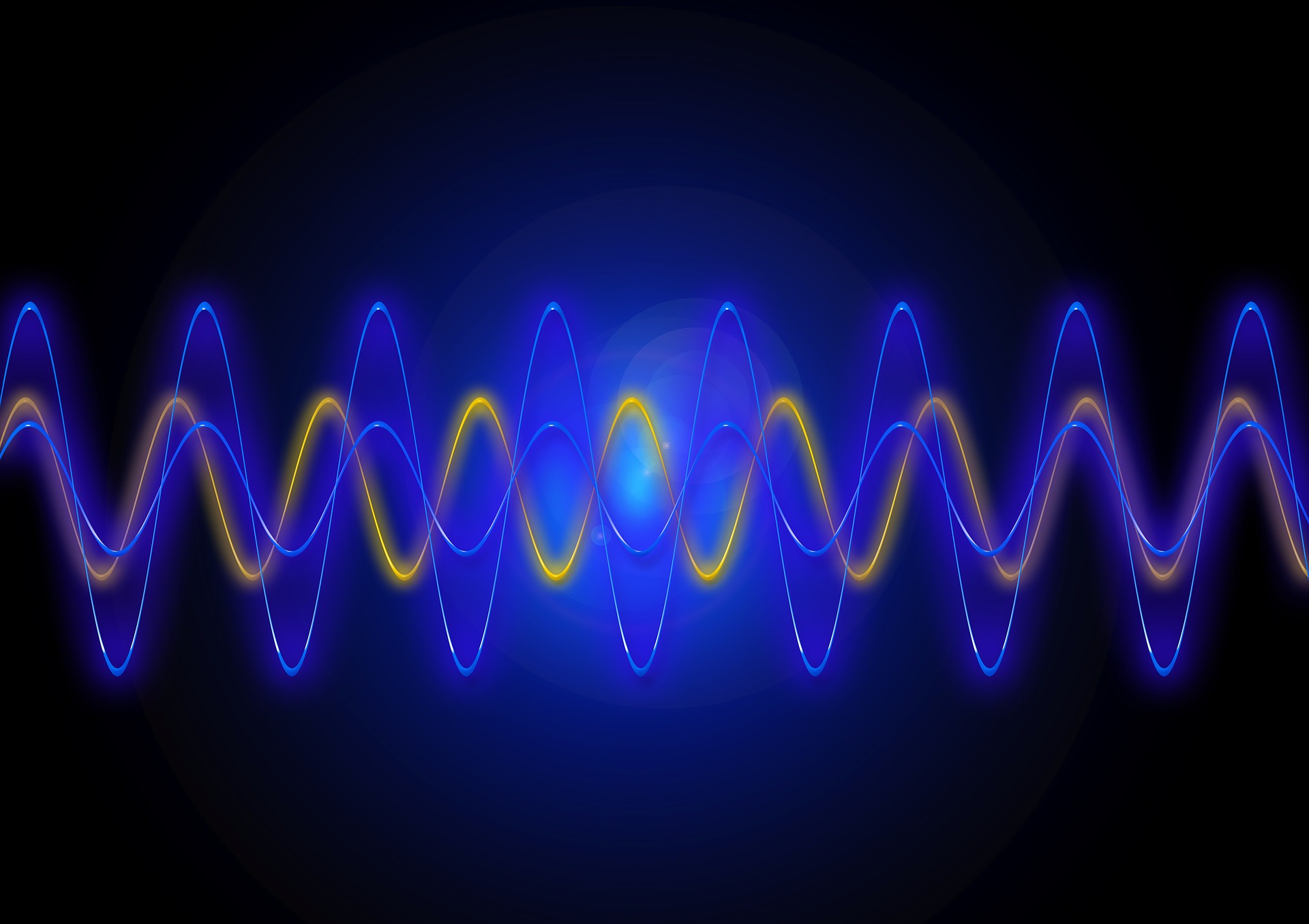
- Read more about DEEPFAKE DETECTION VIA SEPARABLE SELF-CONSISTENCY LEARNING SUPPLEMENTARY MATERIAL
- Log in to post comments
This document provides a supplementary material called "Deepfake Detection via Separable Self-Consistency Learning" submitted to the regular track of the ICIP 2024. The document contains the video-Level comprehensive results, frame-level results.
- Categories:
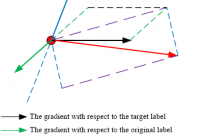
- Read more about Enhancing targeted transferability via suppressing high-confidence labels
- Log in to post comments
While extensive studies have pushed the limit of the transferability of untargeted attacks, transferable targeted attacks remain extremely challenging. This paper finds that the labels with high confidence in the source model are also likely to retain high confidence in the target model. This simple and intuitive observation inspires us to carefully deal with the high-confidence labels in generating targeted adversarial examples for better transferability.
- Categories:
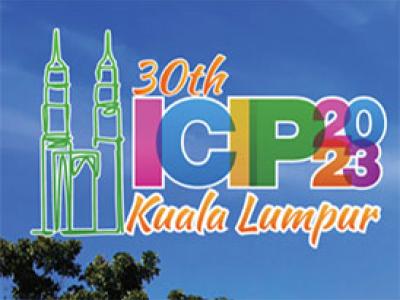
- Read more about ADVERSARIAL EXAMPLE DETECTION BAYESIAN GAME
- Log in to post comments
Despite the increasing attack ability and transferability of adversarial examples (AE), their security, i.e., how unlikely they can be detected, has been ignored more or less. Without the ability to circumvent popular detectors, the chance that an AE successfully fools a deep neural network is slim. This paper gives a game theory analysis of the interplay between an AE attacker and an AE detection investigator. Taking the perspective of a third party, we introduce a game theory model to evaluate the ultimate performance when both the attacker and the investigator are aware of each other.
- Categories:
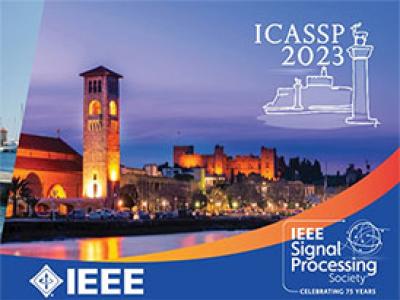
- Read more about EXPLOITING PRNU AND LINEAR PATTERNS IN FORENSIC CAMERA ATTRIBUTION UNDER COMPLEX LENS DISTORTION CORRECTION
- Log in to post comments
More complex and ever more common lens distortion correction post-processing is seriously hampering state-of-the-art camera attribution techniques. In this paper, we show that
ICASSP_OFF.pdf

- Categories:
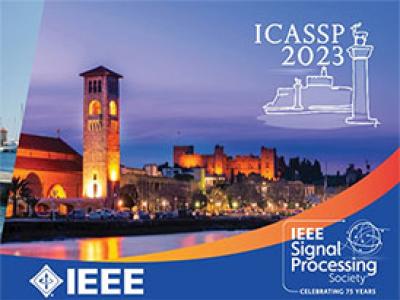
- Read more about ASSD: Synthetic Speech Detection in the AAC Compressed Domain
- Log in to post comments
Synthetic human speech signals have become very easy to generate given modern text-to-speech methods. When these signals are shared on social media they are often compressed using the Advanced Audio Coding (AAC) standard. Our goal is to study if a small set of coding metadata contained in the AAC compressed bit stream is sufficient to detect synthetic speech. This would avoid decompressing of the speech signals before analysis. We call our proposed method AAC Synthetic Speech Detection (ASSD).
- Categories:
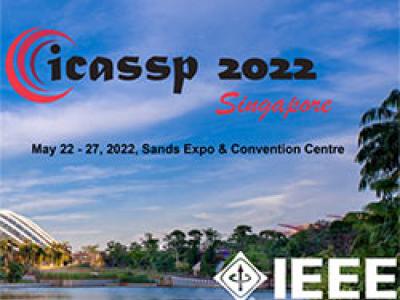
- Read more about DEEPFAKE SPEECH DETECTION THROUGH EMOTION RECOGNITION: A SEMANTIC APPROACH
- Log in to post comments
In recent years, audio and video deepfake technology has advanced relentlessly, severely impacting people's reputation and reliability.
Several factors have facilitated the growing deepfake threat.
On the one hand, the hyper-connected society of social and mass media enables the spread of multimedia content worldwide in real-time, facilitating the dissemination of counterfeit material.
- Categories:
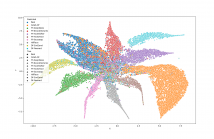
- Read more about Custom attribution loss for improving generalization and interpretability of deepfake detection
- Log in to post comments
The simplicity and accessibility of tools for generating deepfakes pose a significant technical challenge for their detection and filtering. Many of the recently proposed methods for deeptake detection focus on a `blackbox' approach and therefore suffer from the lack of any additional information about the nature of fake videos beyond the fake or not fake labels. In this paper, we approach deepfake detection by solving the related problem of attribution, where the goal is to distinguish each separate type of a deepfake attack.
- Categories:
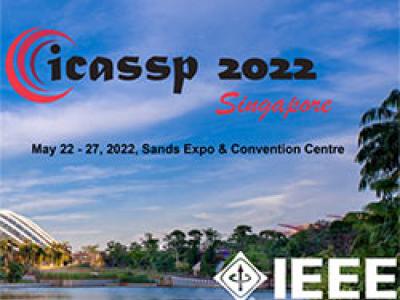
- Read more about A ROBUST DEEP AUDIO SPLICING DETECTION METHOD VIA SINGULARITY DETECTION FEATURE
- Log in to post comments
There are many methods for detecting forged audio produced by conversion and synthesis. However, as a simpler method of forgery, splicing has not attracted widespread attention.
Based on the characteristic that the tampering operation will cause singularities at high-frequency components, we propose a high-frequency singularity detection feature obtained
- Categories:
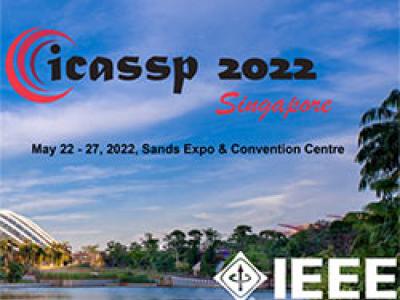
- Read more about A ROBUST DEEP AUDIO SPLICING DETECTION METHOD VIA SINGULARITY DETECTION FEATURE
- Log in to post comments
There are many methods for detecting forged audio produced by conversion and synthesis. However, as a simpler method of forgery, splicing has not attracted widespread attention.
Based on the characteristic that the tampering operation will cause singularities at high-frequency components, we propose a high-frequency singularity detection feature obtained
- Categories:
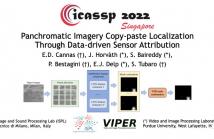
- Read more about Panchromatic imagery copy-paste localization through data-driven sensor attribution
- Log in to post comments
Overhead images can be obtained using different acquisition and processing techniques, and they are becoming more and more popular. As with common photographs, they can be forged and manipulated by malicious users. However, not all image forensics methods tailored to normal photos can be successfully applied out of the box to overhead images. In this paper we consider the problem of localizing copy-paste forgeries on panchromatic images acquired with different satellites.
- Categories: