- Read more about THE WITS INTELLIGENT TEACHING SYSTEM: DETECTING STUDENT ENGAGEMENT DURING LECTURES USING CONVOLUTIONAL NEURAL NETWORKS
- Log in to post comments
- Categories:
- Read more about DenseNet for Dense Flow
- Log in to post comments
Classical approaches for estimating optical flow have achieved rapid progress in the last decade. However, most of them are too slow to be applied in real-time video analysis. Due to the great success of deep learning, recent work has focused on using CNNs to solve such dense prediction problems. In this paper, we investigate a new deep architecture, Densely Connected Convolutional Networks (DenseNet), to learn optical flow. This specific architecture is ideal for the problem at hand as it provides shortcut connections throughout the network, which leads to implicit deep supervision.
- Categories:
- Read more about TOWARDS THINNER CONVOLUTIONAL NEURAL NETWORKS THROUGH GRADUALLY GLOBAL PRUNING
- Log in to post comments
Deep network pruning is an effective method to reduce the storage and computation cost of deep neural networks when applying them to resource-limited devices. Among many pruning granularities, neuron level pruning will remove redundant neurons and filters in the model and result in thinner networks. In this paper, we propose a gradually global pruning scheme for neuron level pruning. In each pruning step,
- Categories:
In this paper, we propose a new approach for searching action proposals in unconstrained videos. Our method first produces snippet action proposals by combining state-of-the-art YOLO detector (Static YOLO) and our regression based RNN detector (Recurrent YOLO). Then, these short action proposals are integrated to form final action proposals by solving two-pass dynamic programming which maximizes actioness score and temporal smoothness concurrently.
- Categories:
- Read more about Foveated Neural Network: Gaze Prediction On Egocentric Videos
- Log in to post comments
A novel deep convolution neural network, named as Foveated Neural Network (FNN), is proposed to predict gaze on current frames in egocentric videos. The retina-like visual inputs from the region of interest on the previous frame get analysed and encoded. The fusion of the hidden representation of the previous frame and the feature maps of the current frame guides the gaze prediction process on the current frame. In order to simulate motions, we also include the dense optical flow between these adjacent frames as additional inputs to FNN.
- Categories:
- Read more about LEARNING TO GENERATE IMAGES WITH PERCEPTUAL SIMILARITY METRICS (POSTER)
- Log in to post comments
- Categories:
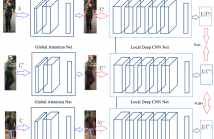
- Read more about PERSON RE-IDENTIFICATION USING VISUAL ATTENTION
- Log in to post comments
Despite recent attempts for solving the person re-identification problem, it remains a challenging task since a person’s appearance can vary significantly when large variations in view angle, human pose and illumination are involved. The concept of attention is one of the most interesting recent architectural innovations in neural networks. Inspired by that, in this paper we propose a novel approach based on using a gradient-based attention mechanism in deep convolution neural network for solving the person re-identification problem.
- Categories:
- Read more about MOTION BLUR REMOVAL VIA COUPLED AUTOENCODER
- Log in to post comments
ICIP_ppt.pdf

- Categories:
- Read more about LEARNING AUTOENCODERS WITH LOW-RANK WEIGHTS
- Log in to post comments
- Categories: