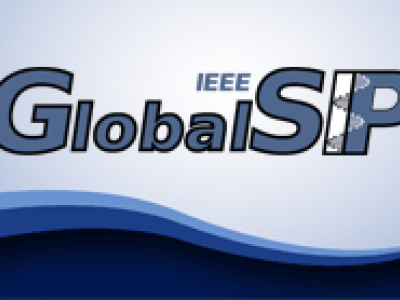
- Read more about COMPLEX INPUT CONVOLUTIONAL NEURAL NETWORKS FOR WIDE ANGLE SAR ATR
- Log in to post comments
To date, automatic target recognition (ATR) techniques in synthetic aperture radar (SAR) imagery have largely focused on features that use only the magnitude part of SAR’s complex valued magnitude-plus-phase history. While such techniques are often very successful, they inherently ignore the significant amount of discriminatory information available in the phase. This paper describes a method for exploiting the complex information for ATR by using a convolutional neural network (CNN) that accepts fully complex input features.
- Categories:
- Read more about Multi-Task Joint-Learning for Robust Voice Activity Detection
- Log in to post comments
Model based VAD approaches have been widely used and
achieved success in practice. These approaches usually cast
VAD as a frame-level classification problem and employ statistical
classifiers, such as Gaussian Mixture Model (GMM) or
Deep Neural Network (DNN) to assign a speech/silence label
for each frame. Due to the frame independent assumption classification,
the VAD results tend to be fragile. To address this
problem, in this paper, a new structured multi-frame prediction
DNN approach is proposed to improve the segment-level
- Categories:
- Read more about Lecture ICASSP 2016 Pierre Laffitte
- Log in to post comments
This presentation introduces a Deep Learning model that performs classification of the Audio Scene in the subway environment. The final goal is to detect Screams and Shouts for surveillance purposes. The model is a combination of Deep Belief Network and Deep Neural Network, (generatively pre-trained within the DBN framework and fine-tuned discriminatively within the DNN framework), and is trained on a novel database of pseudo-real signals collected in the Paris metro.
- Categories:
- Read more about Supervised Subspace Learning based on Deep Randomized Networks
- Log in to post comments
In this paper, we propose a supervised subspace learning method that exploits the rich representation power of deep feedforward networks. In order to derive a fast, yet efficient, learning scheme we employ deep randomized neural networks that have been recently shown to provide good compromise between training speed and performance.
- Categories:
- Read more about Mediated Mixture of Experts
- Log in to post comments
poster_win.pdf

- Categories:
- Read more about Face Alignment by Deep Convolutional Network with Adaptive Learning Rate
- Log in to post comments
Deep convolutional network has been widely used in face recognition while not often used in face alignment. One of the most important reasons of this is the lack of training images annotated with landmarks due to fussy and time-consuming annotation work. To overcome this problem, we propose a novel data augmentation strategy. And we design an innovative training algorithm with adaptive learning rate for two iterative procedures, which helps the network to search an optimal solution.
- Categories:
- Read more about Characterization and Classification of Sonar Targets Using Ellipsoid Features
- Log in to post comments
We present a geometry-inspired characterization of
target response for active sonar that exploits similarity between
intra-class features to distinguish between different targets
against environmental objects such as a rock. Key innovation is to
represent feature manifolds as a set of ellipsoids, each of which
geometrically encompasses a unique physical characteristic of
the target’s response. We have demonstrated over experimental
field data that for a given target class, these feature ellipsoids
- Categories:
- Read more about Deep Learning in Exploring Semantic Relatedness for Microblog Dimensionality Reduction_PPT
- Log in to post comments
- Categories:
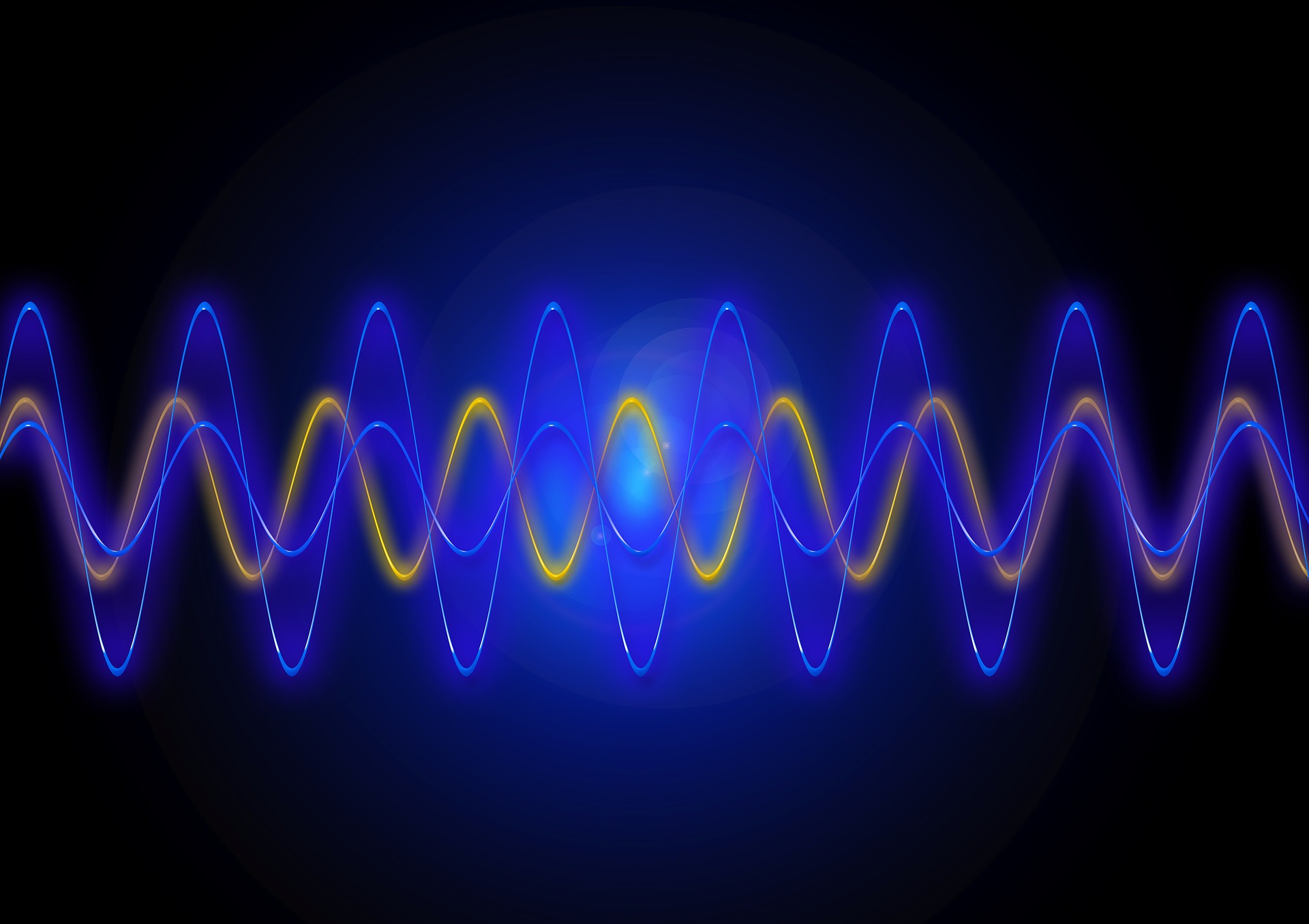
- Read more about Deep Learning in Exploring Semantic Relatedness for Microblog Dimensionality Reduction
- Log in to post comments
- Categories: