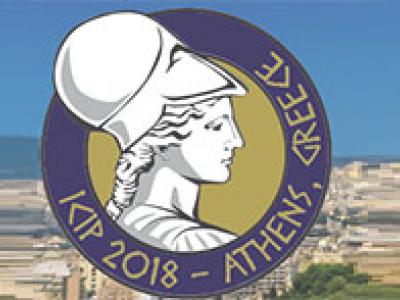
- Read more about Co-occurrence Matrix Analysis Based Semi-Supervised Training for Object Detection
- Log in to post comments
One of the most important factors in training object recognition networks using convolutional neural networks (CNN) is the provision of annotated data accompanying human judgment. Particularly, in object detection or semantic segmentation, the annotation process requires considerable human effort. In this paper, we propose a semi-supervised learning (SSL)-based training methodology for object detection, which makes use of automatic labeling of un-annotated data by applying a network previously trained from an annotated dataset.
- Categories:
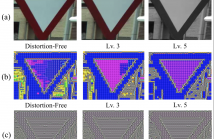
In this paper, we generate and control semantically interpretable filters that are directly learned from natural images in an unsupervised fashion. Each semantic filter learns a visually interpretable local structure in conjunction with other filters. The significance of learning these interpretable filter sets is demonstrated on two contrasting applications. The first application is image recognition under progressive decolorization, in which recognition algorithms should be color-insensitive to achieve a robust performance.
- Categories:
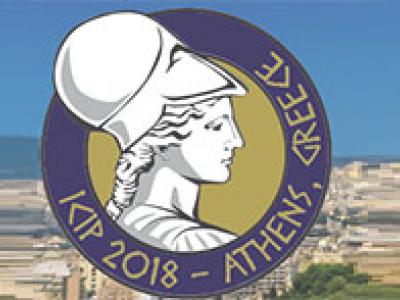
- Read more about TS-Net: Combining Modality Specific and Common Features for Multimodal Patch Matching
- Log in to post comments
- Categories:
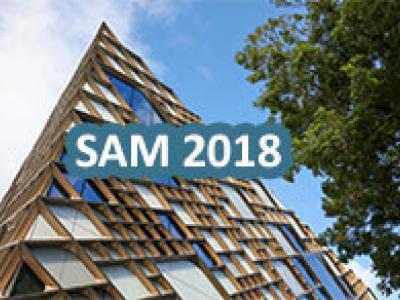
- Read more about WET-DRY CLASSIFICATION USING LSTM AND COMMERCIALMICROWAVE LINKS
- Log in to post comments
The task of rain detection, or wet-dry classification
using measurements from commercial microwave links (CMLs)
is a subject that been studied in depth. However, these studies
are based on direct measurement of the signal level, which
is known to be attenuated by rain. In this paper we present,
for the first time an empirical study on rain classification using
records of transmissions errors in the CMLs. Based on a dataset
of measurements taken from operational cellular backhaul
networks and meteorological measurements, and using long
- Categories:
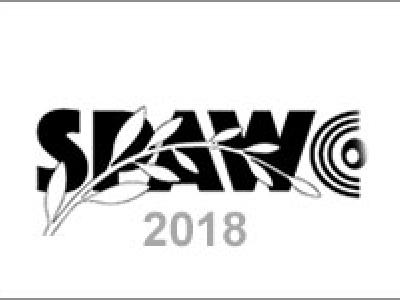
- Read more about Learning-Based Antenna Selection for Multicasting
- Log in to post comments
In multi-antenna systems, it is preferred to activate only a subset of the available transmit antennas in order to save hardware and energy resources, without seriously degrading the system performance. However, antenna selection often poses very hard optimization problems. Joint multicast beamforming and antenna selection is one particular example, which is often approached by Semi-Definite Relaxation (SDR) type approximations. The drawback is that SDR lifts the problem to a much higher dimension, leading to considerably high memory and computational complexities.
- Categories:
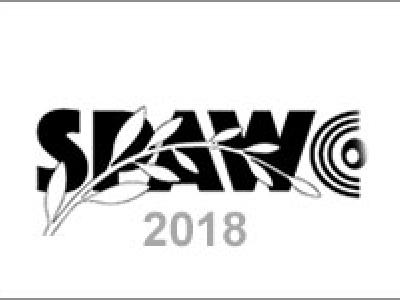
- Read more about Time Series Prediction via Recurrent Neural Networks with the Information Bottleneck Principle
- Log in to post comments
- Categories:
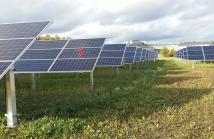
- Read more about PREDICTIVE MAINTENANCE OF PHOTOVOLTAIC PANELS VIA DEEP LEARNING
- Log in to post comments
We apply convolutional neural networks (CNN) for monitoring the
operation of photovoltaic panels. In particular, we predict the daily
electrical power curve of a photovoltaic panel based on the power
curves of neighboring panels. An exceptionally large deviation between
predicted and actual (observed) power curve indicates a malfunctioning
panel. The problem is challenging because the power
curve depends on many factors such as weather conditions and the
surrounding objects causing shadows with a regular time pattern. We
- Categories:
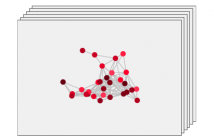
- Read more about Convolutional Neural Networks via Node-Varying Graph Filters
- Log in to post comments
Convolutional neural networks (CNNs) are being applied to an increasing number of problems and fields due to their superior performance in classification and regression tasks. Since two of the key operations that CNNs implement are convolution and pooling, this type of networks is implicitly designed to act on data described by regular structures such as images. Motivated by the recent interest in processing signals defined in irregular domains, we advocate a CNN architecture that operates on signals supported on graphs.
- Categories:
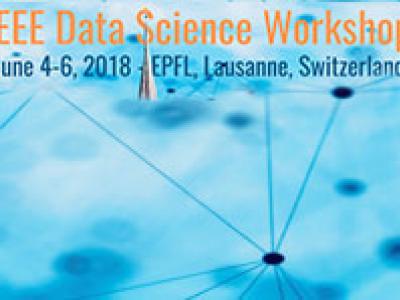
- Read more about Deep CNN Sparse Coding Analysis
- Log in to post comments
Deep Convolutional Sparse Coding (D-CSC) is a framework reminiscent
of deep convolutional neural nets (DCNN), but by omitting the learning of the
dictionaries one can more transparently analyse the role of the
activation function and its ability to recover activation paths
through the layers. Papyan, Romano, and Elad conducted an analysis of
such an architecture \cite{2016arXiv160708194P}, demonstrated the
relationship with DCNNs and proved conditions under which a D-CSC is
guaranteed to recover activation paths. A technical innovation of
- Categories:
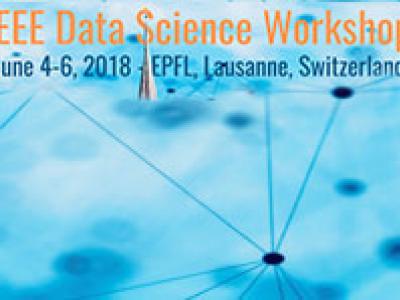
- Read more about MOTIFNET: A MOTIF-BASED GRAPH CONVOLUTIONAL NETWORK FOR DIRECTED GRAPHS
- Log in to post comments
Deep learning on graphs and in particular, graph convolutional neural networks, have recently attracted significant attention in the machine learning community. Many of such
MotifNet.pdf

- Categories: