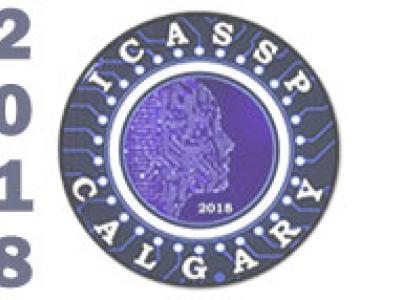
In this paper, we propose a new loss function called generalized end-to-end (GE2E) loss, which makes the training of speaker verification models more efficient than our previous tuple-based end-to-end (TE2E) loss function. Unlike TE2E, the GE2E loss function updates the network in a way that emphasizes examples that are difficult to verify at each step of the training process. Additionally, the GE2E loss does not require an initial stage of example selection.
- Categories:
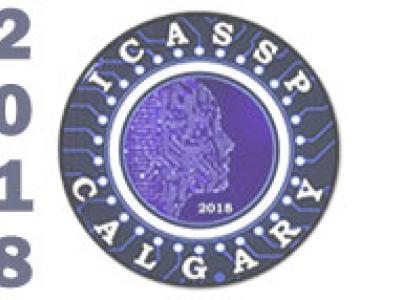
- Read more about A Random Matrix and Concentration Inequalities framework for Neural Networks Analysis
- Log in to post comments
Our article provides a theoretical analysis of the asymptotic performance of a regression or classification task performed by a simple random neural network. This result is obtained by leveraging a new framework at the crossroads between random matrix theory and the concentration of measure theory. This approach is of utmost interest for neural network analysis at large in that it naturally dismisses the difficulty induced by the non-linear activation functions, so long that these are Lipschitz functions.
- Categories:
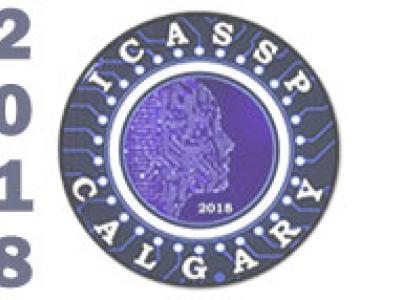
- Read more about Joint Verification-Identification in End-to-End Multi-Scale CNN Framework for Topic Identification
- Log in to post comments
We present an end-to-end multi-scale Convolutional Neural
Network (CNN) framework for topic identification (topic ID).
In this work, we examined multi-scale CNN for classification
using raw text input. Topical word embeddings are learnt at
multiple scales using parallel convolutional layers. A technique
to integrate verification and identification objectives is
examined to improve topic ID performance. With this approach,
we achieved significant improvement in identification
task. We evaluated our framework on two contrasting
- Categories:
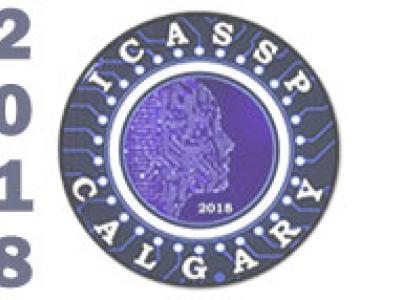
- Read more about Speaker Invariant Feature Extraction for Zero-Resource Languageswith Adversarial Learning
- Log in to post comments
We introduce a novel type of representation learning to obtain a speaker invariant feature for zero-resource languages. Speaker adaptation is an important technique to build a robust acoustic model. For a zero-resource language, however, conventional model-dependent speaker adaptation methods such as constrained maximum likelihood linear regression are insufficient because the acoustic model of the target language is not accessible. Therefore, we introduce a model-independent feature extraction based on a neural network.
- Categories:
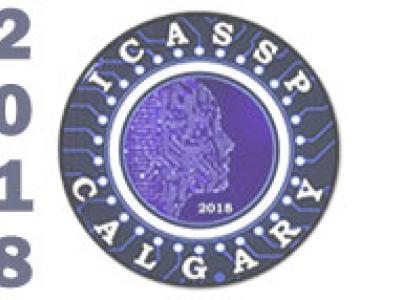
- Read more about A MULTI-CAMERA DEEP NEURAL NETWORK FOR DETECTING ELEVATED ALERTNESS IN DRIVERS
- Log in to post comments
We present a system for the detection of elevated levels of driver alertness in driver-facing video captured from multiple viewpoints. This problem is important in automotive safety as a helpful feedback signal to determine driver engagement and as a means of automatically flagging anomalous driving events. We generated a dataset of videos from 25 participants overseeing an hour each of driving sequences in a simulator consisting of a mixture of normal and near-miss driving events.
poster.pdf

- Categories:

- Read more about CATSEYES: Categorizing Seismic structures with tessellated scattering wavelet networks
- Log in to post comments
As field seismic data sizes are dramatically increasing toward exabytes, automating the labeling of ``structural monads'' --- corresponding to geological patterns and yielding subsurface interpretation --- in a huge amount of available information would drastically reduce interpretation time. Since customary designed features may not account for gradual deformations observable in seismic data, we propose to adapt the wavelet-based scattering network methodology with a tessellation of geophysical images.
- Categories:
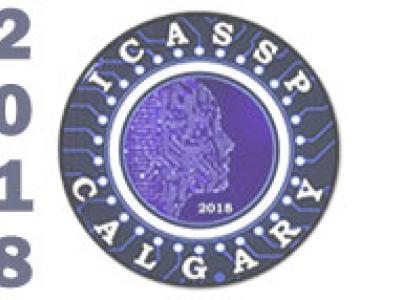
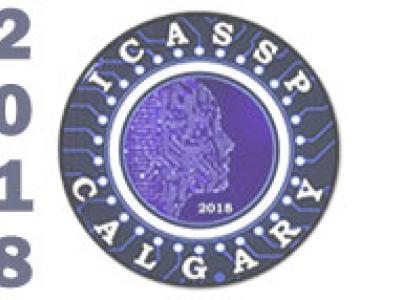
- Read more about Cofnet: Predict with Confidence
- Log in to post comments
POSTER.pdf

- Categories:
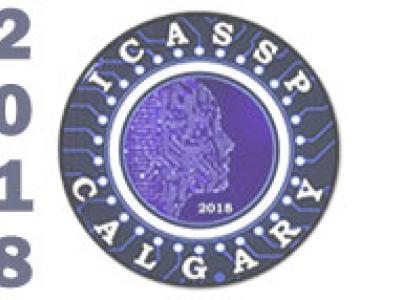
- Read more about A Supervised STDP-Based Training Algorithm for Living Neural Networks
- Log in to post comments
Neural networks have shown great potential in many applications like speech recognition, drug discovery, image classification, and object detection. Neural network models are inspired by biological neural networks, but they are optimized to perform machine learning tasks on digital computers.
- Categories:
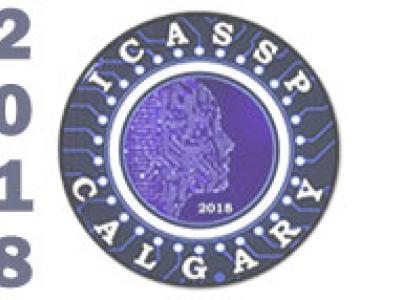
- Read more about SOLVING LINEAR INVERSE PROBLEMS USING GAN PRIORS: AN ALGORITHM WITH PROVABLE GUARANTEES
- Log in to post comments
In recent works, both sparsity-based methods as well as learning-based methods have proven to be successful in solving several challenging linear inverse problems. However, sparsity priors for natural signals and images suffer from poor discriminative capability, while learning-based methods seldom provide concrete theoretical guarantees. In this work, we advocate the idea of replacing hand-crafted priors, such as sparsity, with a Generative Adversarial Network (GAN) to solve linear inverse problems such as compressive sensing.
- Categories: