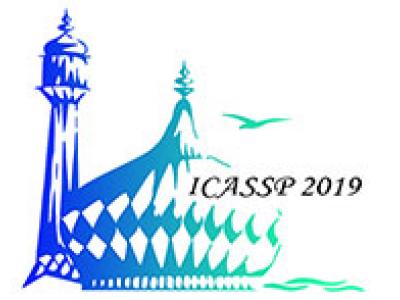
- Read more about On the Usefulness of Statistical Normalisation of Bottleneck Features for Speech Recognition
- Log in to post comments
DNNs play a major role in the state-of-the-art ASR systems. They can be used for extracting features and building probabilistic models for acoustic and language modelling. Despite their huge practical success, the level of theoretical understanding has remained shallow. This paper investigates DNNs from a statistical standpoint. In particular, the effect of activation functions on the distribution of the pre-activations and activations is investigated and discussed from both analytic and empirical viewpoints.
- Categories:
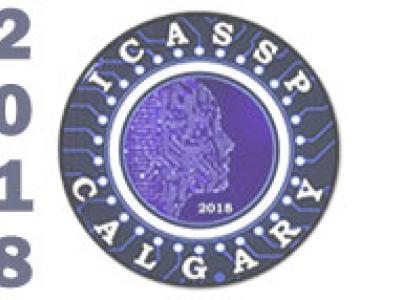
- Read more about SOUND SOURCE SEPARATION USING PHASE DIFFERENCE AND RELIABLE MASK SELECTION SELECTION
- Log in to post comments
In this paper, we present an algorithm called Reliable Mask Selection-Phase Difference Channel Weighting (RMS-PDCW) which selects the target source masked by a noise source using the Angle of Arrival (AoA) information calculated using the phase difference information. The RMS-PDCW algorithm selects masks to apply using the information about the localized sound source and the onset detection of speech.
- Categories:
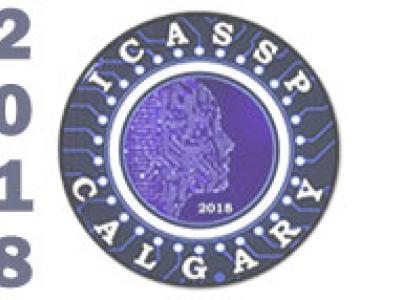
- Read more about SPECTRAL DISTORTION MODEL FOR TRAINING PHASE-SENSITIVE DEEP-NEURAL NETWORKS FOR FAR-FIELD SPEECH RECOGNITION
- Log in to post comments
In this paper, we present an algorithm which introduces phase-perturbation to the training database when training phase-sensitive deep neural-network models. Traditional features such as log-mel or cepstral features do not have have any phase-relevant information.However features such as raw-waveform or complex spectra features contain phase-relevant information. Phase-sensitive features have the advantage of being able to detect differences in time of
- Categories:
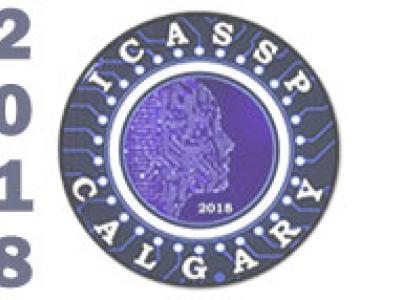
- Read more about Spectral feature mapping with mimic loss for robust speech recognition
- Log in to post comments
For the task of speech enhancement, local learning objectives are agnostic to phonetic structures helpful for speech recognition. We propose to add a global criterion to ensure de-noised speech is useful for downstream tasks like ASR. We first train a spectral classifier on clean speech to predict senone labels. Then, the spectral classifier is joined with our speech enhancer as a noisy speech recognizer. This model is taught to imitate the output of the spectral classifier alone on clean speech.
- Categories:
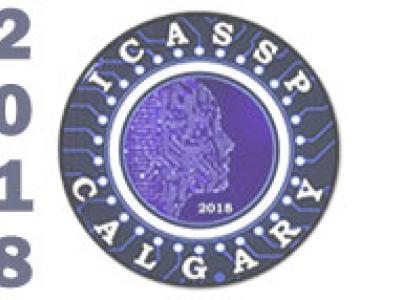
- Read more about EXPLORING THE USE OF GROUP DELAY FOR GENERALISED VTS BASED NOISE COMPENSATION
- Log in to post comments
In earlier work we studied the effect of statistical normalisation for phase-based features and observed it leads to a significant robustness improvement. This paper explores the extension of the generalised Vector Taylor Series (gVTS) noise compensation approach to the group delay (GD) domain. We discuss the problems it presents, propose some solutions and derive the corresponding formulae. Furthermore, the effects of additive and channel noise in the GD domain were studied.
- Categories:
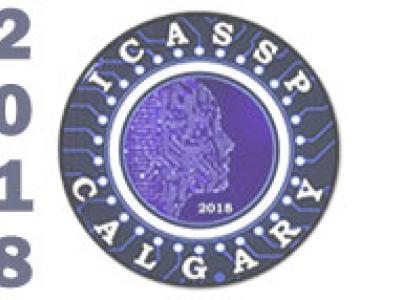
For speech recognition in noisy environments, we propose a multi-task autoencoder which estimates not only clean speech but also noise from noisy speech. We introduce the deSpeeching autoencoder, which excludes speech signals from noisy speech, and combines it with the conventional denoising autoencoder to form a unified multi-task autoencoder (MTAE). We evaluate it using the Aurora 2 data set and 6-hour noise data set collected by ourselves. It reduced WER by 15.7% from the conventional denoising autoencoder in the Aurora 2 test set A.
- Categories:
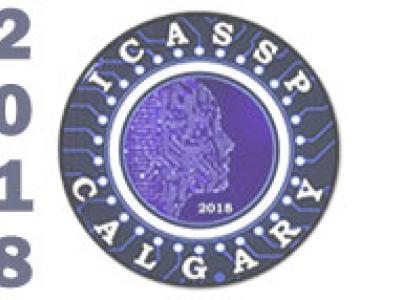
- Read more about Sequence Modeling in Unsupervised Single-channel Overlapped Speech Recognition
- Log in to post comments
Unsupervised single-channel overlapped speech recognition is one
of the hardest problems in automatic speech recognition (ASR). The
problems can be modularized into three sub-problems: frame-wise
interpreting, sequence level speaker tracing and speech recognition.
Nevertheless, previous acoustic models formulate the correlation between sequential labels implicitly, which limit the modeling effect.
In this work, we include explicit models for the sequential label
correlation during training. This is relevant to models given by both
- Categories:
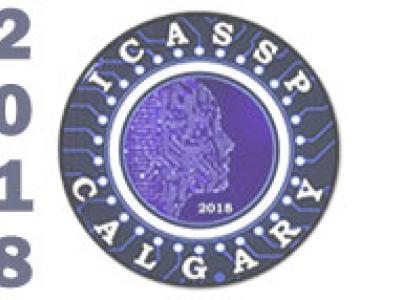
- Read more about Robust Recognition of Speech with Background Music in Acoustically Under-Resourced Scenarios
- Log in to post comments
This paper addresses the task of Automatic Speech Recognition
(ASR) with music in the background. We consider two different
situations: 1) scenarios with very small amount of labeled training
utterances (duration 1 hour) and 2) scenarios with large amount of
labeled training utterances (duration 132 hours). In these situations,
we aim to achieve robust recognition. To this end we investigate
the following techniques: a) multi-condition training of the acoustic
model, b) denoising autoencoders for feature enhancement and c)
- Categories:
- Read more about IMPROVED CEPSTRA MINIMUM-MEAN-SQUARE-ERROR NOISE REDUCTION ALGORITHM FOR ROBUST SPEECH RECOGNITION
- Log in to post comments
- Categories:
- Read more about Speech Activity Detection in Online Broadcast Transcription Using Deep Neural Networks and Weighted Finite State Transducers
- Log in to post comments
A new approach to online Speech Activity Detection (SAD) is proposed. This approach is designed for the use in a system that carries out 24/7 transcription of radio/TV broadcasts containing a large amount of non-speech segments. To improve the robustness of detection, we adopt Deep Neural Networks (DNNs) trained on artificially created mixtures of speech and non-speech signals at desired levels of Signal-to-Noise Ratio (SNR). An integral part of our approach is an online decoder based on Weighted Finite State Transducers (WFSTs); this decoder smooths the output from DNN.
poster.pdf

- Categories: