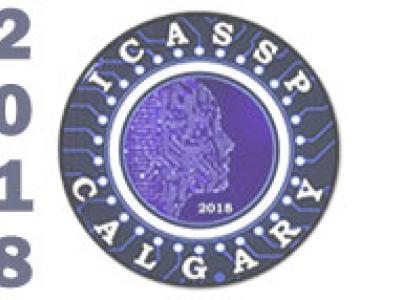
- Read more about FAST AND ADAPTIVE BLIND AUDIO SOURCE SEPARATION USING RECURSIVE LEVENBERG-MARQUARDT SYNCHROSQUEEZING
- Log in to post comments
This paper revisits the Degenerate Unmixing Estimation Technique (DUET) for blind audio separation of an arbitrary
number of sources given two mixtures through a recursively computed and adaptive time-frequency representation.
Recently, synchrosqueezing was introduced as a promising signal disentangling method which allows to compute reversible
and sharpen time-frequency representations. Thus, it can be used to reduce overlaps between the sources in the
poster.pdf

- Categories:
- Read more about ARTIFICIAL BANDWIDTH EXTENSION USING THE CONSTANT Q TRANSFORM
- Log in to post comments
- Categories:
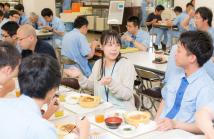
- Read more about Low-Latency Real-Time Blind Source Separation for Hearing Aids Based on Time-Domain Implementation of Online Independent Vector Analysis with Truncation of Non-Causal Components
- Log in to post comments
In this paper, we present a low-latency scheme for real-time blind source separation (BSS) based on online auxiliary-function-based independent vector analysis (AuxIVA). In many real-time audio ap- plications, especially hearing aids, low latency is highly desirable. Conventional frequency-domain BSS methods suffer from a delay caused by frame analysis. To reduce the delay, we implement sep- aration filters as multiple FIR filters in the time domain, which are converted from demixing matrices estimated by online AuxIVA in the frequency domain.
- Categories:
- Read more about Learning complex-valued latent filters with absolute cosine similarity
- Log in to post comments
- Categories:
- Read more about Learning complex-valued latent filters with absolute cosine similarity
- Log in to post comments
We propose a new sparse coding technique based on the power mean of phase-invariant cosine distances. Our approach is a generalization of sparse filtering and K-hyperlines clustering. It offers a better sparsity enforcer than the L1/L2 norm ratio that is typically used in sparse filtering. At the same time, the proposed approach scales better than the clustering counter parts for high-dimensional input. Our algorithm fully exploits the prior information obtained by preprocessing the observed data with whitening via an efficient row-wise decoupling scheme.
- Categories:
- Categories:
- Read more about PHASE ESTIMATION IN SINGLE-CHANNEL SPEECH ENHANCEMENT USING PHASE INVARIANCE CONSTRAINTS
- Log in to post comments
Phase-aware signal processing has received increasing interest
in many speech applications. The success of phase-aware
processing depends strongly on the robustness of the clean
spectral phase estimates to be obtained from a noisy observation.
In this paper, we propose a novel harmonic phase estimator
relying on the phase invariance property exploiting
relations between harmonics using the phase structure. We
present speech quality results achieved in speech enhancement
to justify the effectiveness of the proposed phase estimator
ICASSP2017.pdf

- Categories:
- Read more about Improving Music Source Separation based on DNNs through Data Augmentation and Network Blending
- Log in to post comments
This paper deals with the separation of music into individual instrument tracks which is known to be a challenging problem. We describe two different deep neural network architectures for this task, a feed-forward and a recurrent one, and show that each of them yields themselves state-of-the art results on the SiSEC DSD100 dataset. For the recurrent network, we use data augmentation during training and show that even simple separation networks are prone to overfitting if no data augmentation is used.
- Categories:
- Read more about PHASE ESTIMATION IN SINGLE-CHANNEL SPEECH ENHANCEMENT USING PHASE INVARIANCE CONSTRAINTS
- Log in to post comments
ICASSP2017.pdf

- Categories:
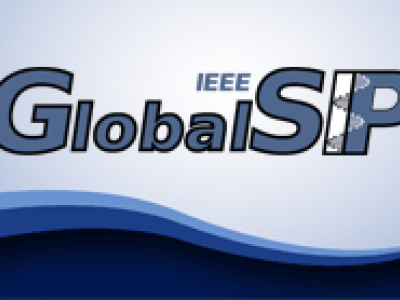
- Read more about LOW-LATENCY SOUND SOURCE SEPARATION USING DEEP NEURAL NETWORKS
- Log in to post comments
Sound source separation at low-latency requires that each in- coming frame of audio data be processed at very low de- lay, and outputted as soon as possible. For practical pur- poses involving human listeners, a 20 ms algorithmic delay is the uppermost limit which is comfortable to the listener. In this paper, we propose a low-latency (algorithmic delay ≤ 20 ms) deep neural network (DNN) based source sepa- ration method.
- Categories: