- Read more about Voice Activity Detection Based on Sequential Gaussian Mixture Model with Maximum Likelihood Criterion
- Log in to post comments
- Categories:
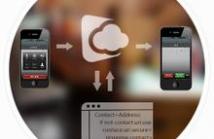
- Read more about Speech Enhancement with Binaural Cues Derived from a Priori Codebook
- Log in to post comments
In conventional codebook-driven speech enhancement, only spectral envelopes of speech and noise are considered, and at the same time, the type of noise is the priori information when we enhance the noisy speech. In this paper, we propose a novel codebook-based speech enhancement method which exploits a priori information about binaural cues, including clean cue and pre-enhanced cue, stored in the trained codebook. This method includes two main parts: offline training of cues and online enhancement by means of cues.
- Categories:
- Read more about A source/filter model with adaptive constraints for NMF-based speech separation [slides]
- Log in to post comments
- Categories:
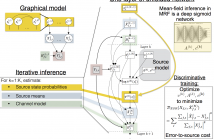
- Read more about Deep Unfolding for Multichannel Source Separation
- Log in to post comments
Deep unfolding has recently been proposed to derive novel deep network architectures from model-based approaches. In this paper, we consider its application to multichannel source separation. We unfold a multichannel Gaussian mixture model (MCGMM), resulting in a deep MCGMM computational network that directly processes complex-valued frequency-domain multichannel audio and has an architecture defined explicitly by a generative model, thus combining the advantages of deep networks and model-based approaches.
- Categories:
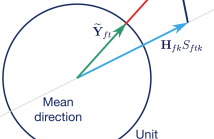
- Read more about Blind Speech Separation based on Complex Spherical k-Mode Clustering
- Log in to post comments
We present an algorithm for clustering complex-valued unit length vectors on the unit hypersphere, which we call complex spherical k-mode clustering, as it can be viewed as a generalization of the spherical k-means algorithm to normalized complex-valued vectors. We show how the proposed algorithm can be derived from the Expectation Maximization algorithm for complex Watson mixture models and prove its applicability in a blind speech separation (BSS) task with real-world room impulse response measurements.
- Categories:
- Read more about Neural Network based Spectral Mask Estimation for Acoustic Beamforming
- Log in to post comments
We present a neural network based approach to acoustic beamform- ing. The network is used to estimate spectral masks from which the Cross-Power Spectral Density matrices of speech and noise are estimated, which in turn are used to compute the beamformer co- efficients. The network training is independent of the number and the geometric configuration of the microphones. We further show that it is possible to train the network on clean speech only, avoid- ing the need for stereo data with separated speech and noise. Two types of networks are evaluated.
- Categories:
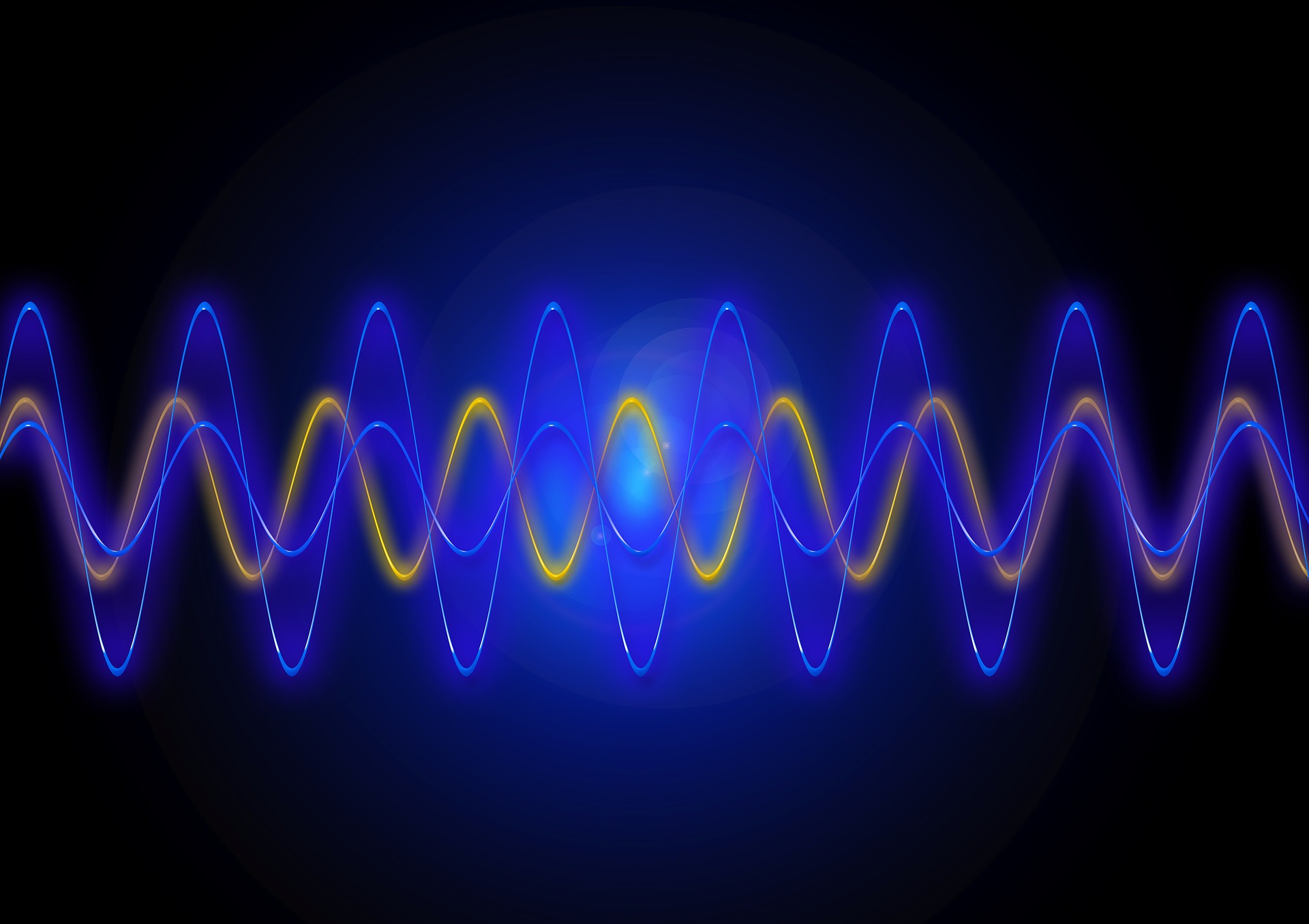
- Read more about NMF-based source separation utilizing prior knowledge on encoding vector
- Log in to post comments
- Categories:
- Read more about Variable Span Filtering for Speech Enhancement
- Log in to post comments
In this work, we consider enhancement of multichannel speech recordings. Linear filtering and subspace approaches have been considered previously for solving the problem. The current linear filtering methods, although many variants exist, have limited control of noise reduction and speech distortion. Subspace approaches, on the other hand, can potentially yield better control by filtering in the eigen-domain, but traditionally these approaches have not been optimized explicitly for traditional noise reduction and signal distortion measures.
- Categories: