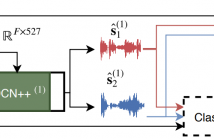
- Read more about Improving Universal Sound Separation Using Sound Classification Presentation
- Log in to post comments
Deep learning approaches have recently achieved impressive performance on both audio source separation and sound classification. Most audio source separation approaches focus only on separating sources belonging to a restricted domain of source classes, such as speech and music. However, recent work has demonstrated the possibility of "universal sound separation", which aims to separate acoustic sources from an open domain, regardless of their class.
- Categories:
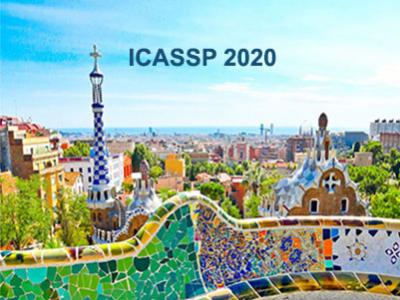
The enhancement of noisy speech is important for applications involving human-to-human interactions, such as telecommunications and hearing aids, as well as human-to-machine interactions, such as voice-controlled systems and robot audition. In this work, we focus on reverberant environments. It is shown that, by exploiting the lack of correlation between speech and the late reflections, further noise reduction can be achieved. This is verified using simulations involving actual acoustic impulse responses and noise from the ACE corpus.
- Categories:
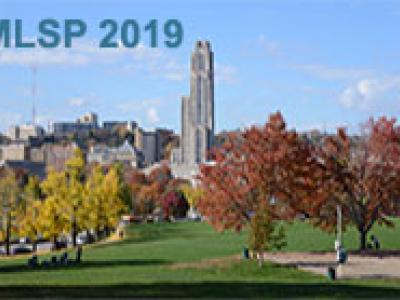
- Read more about A Bayesian Generative Model With Gaussian Process Priors For Thermomechanical Analysis Of Micro-Resonators
- Log in to post comments
Thermal analysis using resonating micro-electromechanical systems shows great promise in characterizing materials in the early stages of research. Through thermal cycles and actuation using a piezoelectric speaker, the resonant behaviour of a model drug, theophylline monohydrate, is measured across the surface whilst using a laser-Doppler vibrometer for readout. Acquired is a sequence of spectra that are strongly correlated in time, temperature and spatial location of the readout. Traditionally, each spectrum is analyzed individually to locate the resonance peak.
- Categories:
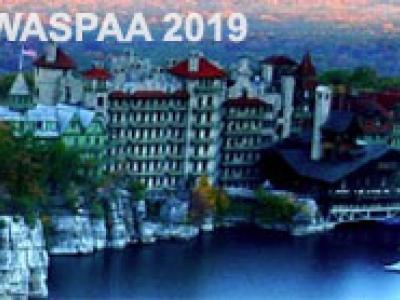
- Read more about Speech Enhancement Using Polynomial Eigenvalue Decomposition
- Log in to post comments
Speech enhancement is important for applications such as telecommunications, hearing aids, automatic speech recognition and voice-controlled system. The enhancement algorithms aim to reduce interfering noise while minimizing any speech distortion. In this work for speech enhancement, we propose to use polynomial matrices in order to exploit the spatial, spectral as well as temporal correlations between the speech signals received by the microphone array.
- Categories:
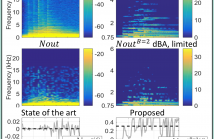
- Read more about An Improved Measure of Musical Noise Based on Spectral Kurtosis
- Log in to post comments
Audio processing methods operating on a time-frequency representation of the signal can introduce unpleasant sounding artifacts known as musical noise. These artifacts are observed in the context of audio coding, speech enhancement, and source separation. The change in kurtosis of the power spectrum introduced during the processing was shown to correlate with the human perception of musical noise in the context of speech enhancement, leading to the proposal of measures based on it. These baseline measures are here shown to correlate with human perception only in a limited manner.
- Categories:
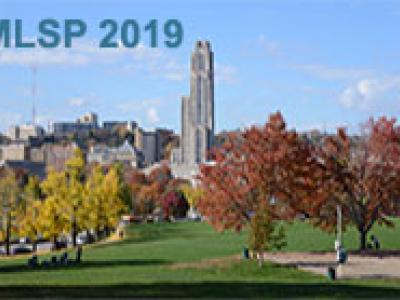
- Read more about Incorporating Intra-Spectral Dependencies With A Recurrent Output Layer For Improved Speech Enhancement
- Log in to post comments
Deep-learning based speech enhancement systems have offered tremendous gains, where the best performing approaches use long short-term memory (LSTM) recurrent neural networks (RNNs) to model temporal speech correlations. These models, however, do not consider the frequency-level correlations within a single time frame, as spectral dependencies along the frequency axis are often ignored. This results in inaccurate frequency responses that negatively affect perceptual quality and intelligibility. We propose a deep-learning approach that considers temporal and frequency-level dependencies.
- Categories:
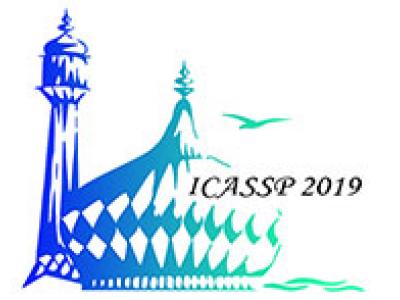
- Read more about Joint Separation and Dereverberation of Reverberant Mixture with Multichannel Variational Autoencoder
- Log in to post comments
AASP_L4_2.pdf

- Categories:
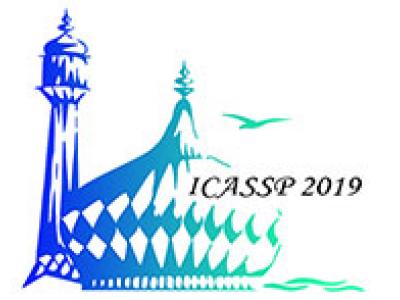
- Read more about Incremental Binarization On Recurrent Neural Networks For Single-Channel Source Separation
- Log in to post comments
This paper proposes a Bitwise Gated Recurrent Unit (BGRU) network for the single-channel source separation task. Recurrent Neural Networks (RNN) require several sets of weights within its cells, which significantly increases the computational cost compared to the fully-connected networks. To mitigate this increased computation, we focus on the GRU cells and quantize the feedforward procedure with binarized values and bitwise operations. The BGRU network is trained in two stages.
- Categories:
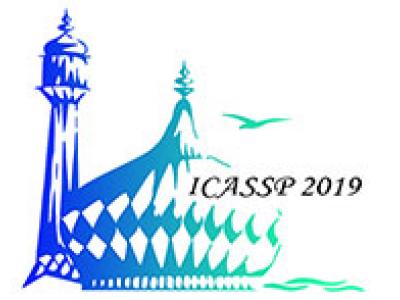
- Read more about Speech Denoising by Parametric Resynthesis
- Log in to post comments
This work proposes the use of clean speech vocoder parameters
as the target for a neural network performing speech enhancement.
These parameters have been designed for text-to-speech
synthesis so that they both produce high-quality resyntheses
and also are straightforward to model with neural networks,
but have not been utilized in speech enhancement until now.
In comparison to a matched text-to-speech system that is given
the ground truth transcripts of the noisy speech, our model is
poster.pdf

- Categories: