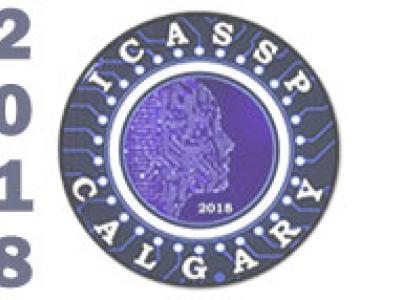
We propose a novel latent variable model for learning latent bases for time-varying non-negative data. Our model uses a mixture multinomial as the likelihood function and proposes a Dirichlet distribution with dynamic parameters as a prior, which we call the dynamic Dirichlet prior. An expectation maximization (EM) algorithm is developed for estimating the parameters of the proposed model.
ICASSP_18.pdf

- Categories:
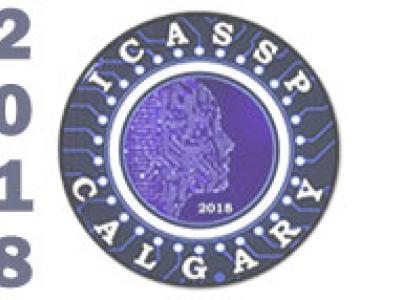
- Read more about TasNet: time-domain audio separation network for real-time, single-channel speech separation
- Log in to post comments
Robust speech processing in multi-talker environments requires effective speech separation. Recent deep learning systems have made significant progress toward solving this problem, yet it remains challenging particularly in real-time, short latency applications. Most methods attempt to construct a mask for each source in time-frequency representation of the mixture signal which is not necessarily an optimal representation for speech separation.
- Categories:
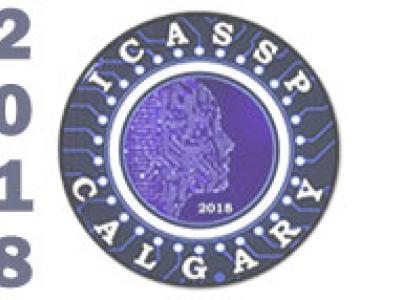
- Read more about ITERATIVE DEEP NEURAL NETWORKS FOR SPEAKER-INDEPENDENT BINAURAL BLIND SPEECH SEPARATION
- Log in to post comments
- Categories:
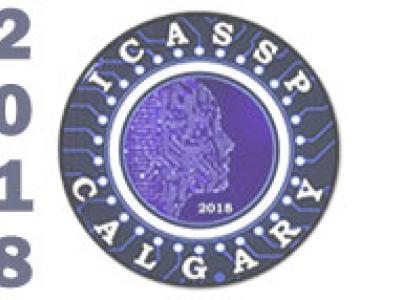
- Read more about BSS EVAL OR PEASS? PREDICTING THE PERCEPTION OF SINGING-VOICE SEPARATION
- Log in to post comments
There is some uncertainty as to whether objective metrics for predicting the perceived quality of audio source separation are sufficiently accurate. This issue was investigated by employing a revised experimental methodology to collect subjective ratings of sound quality and interference of singing-voice recordings that have been extracted from musical mixtures using state-of-the-art audio source separation. A correlation analysis between the experimental data and the measures of two objective evaluation toolkits, BSS Eval and PEASS, was performed to assess their performance.
- Categories:
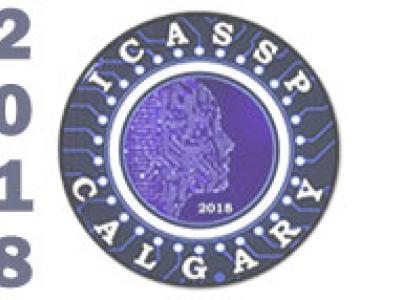
- Read more about Language and Noise Transfer in Speech Enhancement Generative Adversarial Network
- Log in to post comments
- Categories:
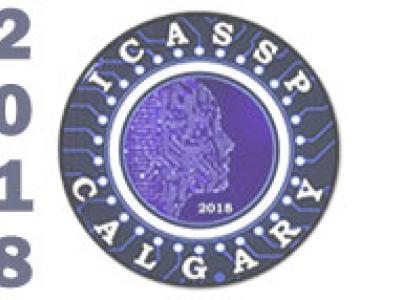
This paper presents an ultimate extension of nonnegative matrix factorization (NMF) for audio source separation based on full covariance modeling over all the time-frequency (TF) bins of the complex spectrogram of an observed mixture signal. Although NMF has been widely used for decomposing an observed power spectrogram in a TF-wise manner, it has a critical limitation that the phase values of interdependent TF bins cannot be dealt with.
- Categories:
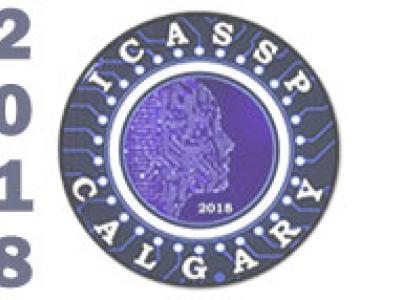
- Read more about Deep Learning Based Speech Beamforming
- Log in to post comments
Multi-channel speech enhancement with ad-hoc sensors has been a challenging task. Speech model guided beamforming algorithms are able to recover natural sounding speech, but the speech models tend to be oversimplified or the inference would otherwise be too complicated. On the other hand, deep learning based enhancement approaches are able to learn complicated speech distributions and perform efficient inference, but they are unable to deal with variable number of input channels.
- Categories:
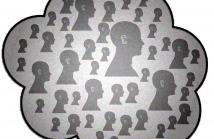
- Read more about Crowdsourced Pairwise-Comparison for Source Separation Evaluation
- Log in to post comments
Automated objective methods of audio source separation evaluation are fast, cheap, and require little effort by the investigator. However, their output often correlates poorly with human quality assessments and typically require ground-truth (perfectly separated) signals to evaluate algorithm performance. Subjective multi-stimulus human ratings (e.g. MUSHRA) of audio quality are the gold standard for many tasks, but they are slow and require a great deal of effort to recruit participants and run listening tests.
- Categories:
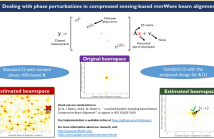
- Read more about PRIMARY-AMBIENT SOURCE SEPARATION FOR UPMIXING TO SURROUNDING SOUND SYSTEMS
- Log in to post comments
Extracting spatial information from an audio recording is a necessary step for upmixing stereo tracks to be played on surround systems. One important spatial feature is the perceived direction of the different audio sources in the recording, which determines how to remix the different sources in the surround system. The focus of this paper is the separation of two types of audio sources: primary (direct) and ambient (surrounding) sources. Several approaches have been proposed to solve the problem, based mainly on the correlation between the two channels in the stereo recording.
- Categories:
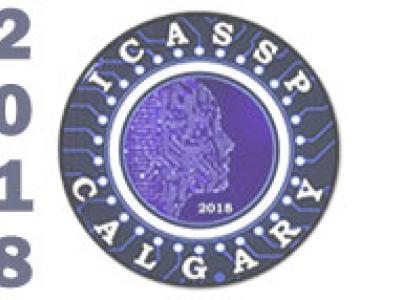
- Read more about JOINTLY TRACKING AND SEPARATING SPEECH SOURCES USING MULTIPLE FEATURES AND THE GENERALIZED LABELED MULTI-BERNOULLI FRAMEWORK
- 2 comments
- Log in to post comments
- Categories: