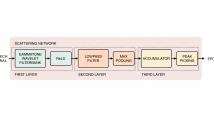
- Read more about EPOCH EXTRACTION FROM A SPEECH SIGNAL USING GAMMATONE WAVELETS IN A SCATTERING NETWORK
- Log in to post comments
In speech production, epochs are glottal closure instants where significant energy is released from the lungs. Extracting an epoch accurately is important in speech synthesis, analysis, and pitch oriented studies. The time-varying characteristics of the source and the system, and channel attenuation of low-frequency components by telephone channels make estimation of epoch from a speech signal a challenging task.
- Categories:
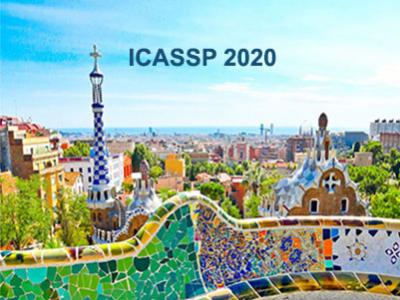
- Read more about ICASSP 2020 Presentation Poster Slides
- Log in to post comments
ONE-SHOT VOICE CONVERSION USING STAR-GAN
- Categories:
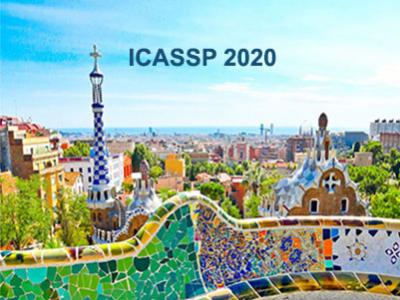
- Read more about Location-Relative Attention Mechanisms For Robust Long-Form Speech Synthesis
- Log in to post comments
Despite the ability to produce human-level speech for in-domain text, attention-based end-to-end text-to-speech (TTS) systems suffer from text alignment failures that increase in frequency for out-of-domain text. We show that these failures can be addressed using simple location-relative attention mechanisms that do away with content-based query/key comparisons. We compare two families of attention mechanisms: location-relative GMM-based mechanisms and additive energy-based mechanisms.
- Categories:
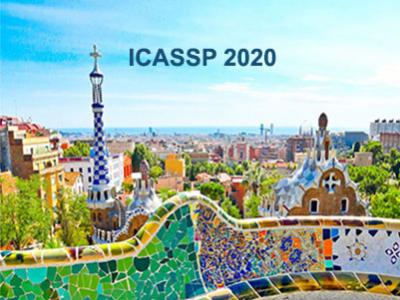
- Read more about Improving LPCNet-based Text-to-Speech with Linear Prediction-structured Mixture Density Network
- Log in to post comments
In this paper, we propose an improved LPCNet vocoder using a linear prediction (LP)-structured mixture density network (MDN).
The recently proposed LPCNet vocoder has successfully achieved high-quality and lightweight speech synthesis systems by combining a vocal tract LP filter with a WaveRNN-based vocal source (i.e., excitation) generator.
- Categories:
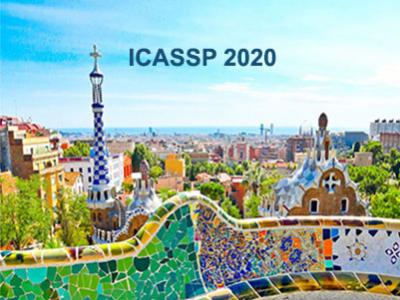
- Read more about 'EMOTIONAL VOICE CONVERSION USING MULTITASK LEARNING WITH TEXT-TO-SPEECH
- Log in to post comments
Voice conversion (VC) is a task that alters the voice of a person to suit different styles while conserving the linguistic content. Previous state-of-the-art technology used in VC was based on the sequence-to-sequence (seq2seq) model, which could lose linguistic information. There was an attempt to overcome this problem using textual supervision; however, this required explicit alignment, and therefore the benefit of using seq2seq model was lost. In this study, a voice converter that utilizes multitask learning with text-to-speech (TTS) is presented.
ICASSP_v0.1.pdf

- Categories:
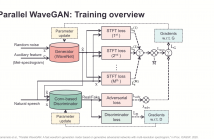
- Read more about PARALLEL WAVEGAN: A FAST WAVEFORM GENERATION MODEL BASED ON GENERATIVE ADVERSARIAL NETWORKS WITH MULTI-RESOLUTION SPECTROGRAM
- Log in to post comments
We propose Parallel WaveGAN, a distillation-free, fast, and small-footprint waveform generation method using a generative adversarial network. In the proposed method, a non-autoregressive WaveNet is trained by jointly optimizing multi-resolution spectrogram and adversarial loss functions, which can effectively capture the time-frequency distribution of the realistic speech waveform. As our method does not require density distillation used in the conventional teacher-student framework, the entire model can be easily trained.
- Categories:
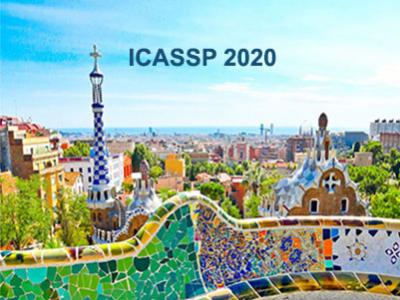
- Read more about IMPROVING PROSODY WITH LINGUISTIC AND BERT DERIVED FEATURES IN MULTI-SPEAKER BASED MANDARIN CHINESE NEURAL TTS
- Log in to post comments
Recent advances of neural TTS have made “human parity” synthesized speech possible when a large amount of studio-quality training data from a voice talent is available. However, with only limited, casual recordings from an ordinary speaker, human-like TTS is still a big challenge, in addition to other artifacts like incomplete sentences, repetition of words, etc.
- Categories:
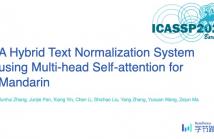
- Read more about A HYBRID TEXT NORMALIZATION SYSTEM USING MULTI-HEAD SELF-ATTENTION FOR MANDARIN
- Log in to post comments
In this paper, we propose a hybrid text normalization system using multi-head self-attention. The system combines the advantages of a rule-based model and a neural model for text preprocessing tasks. Previous studies in Mandarin text normalization usually use a set of hand-written rules, which are hard to improve on general cases. The idea of our proposed system is motivated by the neural models from recent studies and has a better performance on our internal news corpus. This paper also includes different attempts to deal with imbalanced pattern distribution of the dataset.
- Categories:
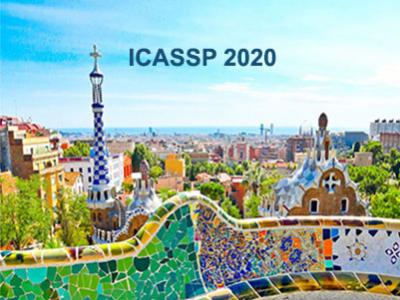
- Read more about A UNIFIED SEQUENCE-TO-SEQUENCE FRONT-END MODEL FOR MANDARIN TEXT-TO-SPEECH SYNTHESIS
- Log in to post comments
- Categories: