Documents
Poster
Discriminant Correlation Analysis for Feature Level Fusion with Application to Multimodal Biometrics
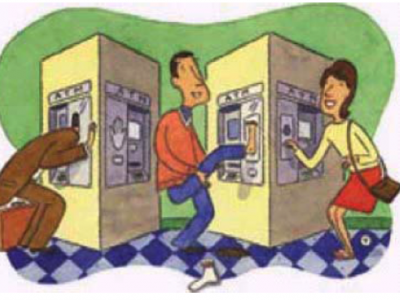
- Citation Author(s):
- Submitted by:
- Mohammad Haghighat
- Last updated:
- 16 July 2016 - 11:13pm
- Document Type:
- Poster
- Document Year:
- 2016
- Event:
- Presenters:
- Mohammad Haghighat
- Paper Code:
- IVMSP-P12.6
- Categories:
- Keywords:
- Log in to post comments
In this paper, we present Discriminant Correlation Analysis (DCA), a feature level fusion technique that incorporates the class associations in correlation analysis of the feature sets. DCA performs an effective feature fusion by maximizing the pair-wise correlations across the two feature sets, and at the same time, eliminating the between-class correlations and restricting the correlations to be within classes. Our proposed method can be used in pattern recognition applications for fusing features extracted from multiple modalities or combining different feature vectors extracted from a single modality. It is noteworthy that DCA is the first technique that considers class structure in feature fusion. Moreover, it has a very low computational complexity and it can be employed in real-time applications. Multiple sets of experiments performed on various biometric databases show the effectiveness of our proposed method, which outperforms other state-of-the-art approaches.
The MATLAB source code for the proposed DCA method is provided at http://www.mathworks.com/matlabcentral/fileexchange/55405.