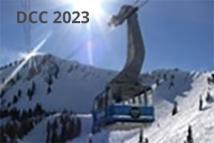
DCC 2023 Conference - The Data Compression Conference (DCC) is an international forum for current work on data compression and related applications. Both theoretical and experimental work are of interest. Visit the DCC 2023 website.
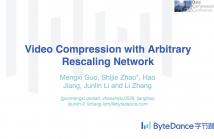
- Read more about Video Compression with Arbitrary Rescaling Network
- 1 comment
- Log in to post comments
Most video platforms provide video streaming services with different qualities, and the resolution of the videos usually adjusts the quality of the services. So high-resolution videos need to be downsampled for compression. In order to solve the problem of video coding at different resolutions, we propose a rate-guided arbitrary rescaling network (RARN) for video resizing before encoding.
- Categories:
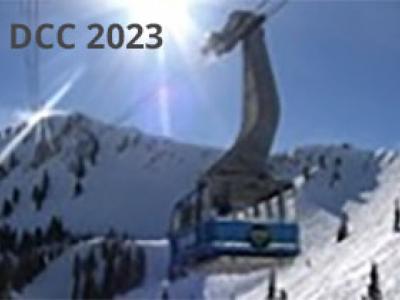
- Read more about Image Compressed Sensing Using Auxiliary Information for Efficient Coding
- Log in to post comments
- Categories:
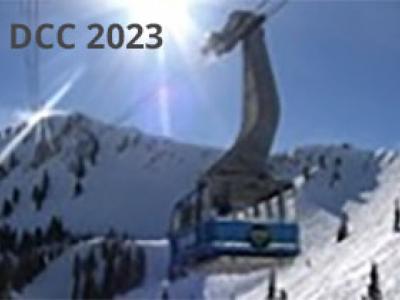
- Read more about Rate-Distortion-Classification Model In Lossy Image Compression
- 1 comment
- Log in to post comments
Rate-distortion (RD) theory is a fundamental theory for lossy image compression that treats compressing the original images to a specified bitrate with minimal signal distortion, which is an essential metric in practical application. Moreover, with the development of visual analysis applications (such as classification, detection, segmentation, etc.), the semantic distortion in compressed images are also an important dimension in the theoretical analysis of lossy image compression.
- Categories:
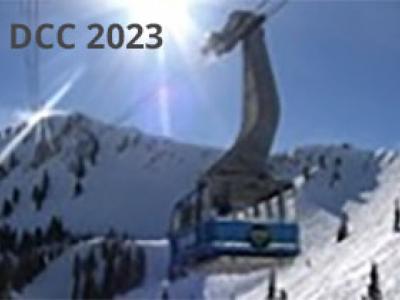
End-to-end learned image compression (LIC) has become promising alternatives for lossy image compression. However, deployments of LIC models are restricted, due to excessive network parameters and high computational complexity. Existing LIC models realized throughout with integer networks are significantly degraded in rate-distortion (R-D) performance. In this paper, we propose a novel fully integerized model for LIC that leverages channel-wise weight and activation quantization.
- Categories:
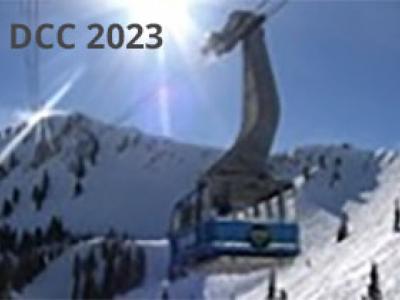
- Read more about Mixed-precision Deep Neural Network Quantization With Multiple Compression Rates
- Log in to post comments
Quantization of one deep neural network to multiple compression rates (precisions) has been recently considered for flexible deployments in real-world scenarios. However, existing methods for network quantization under multiple compression rates leverage fixed-precision bit-width allocation or heuristically search for mixed-precision strategy and cannot well balance efficiency and performance.
- Categories:
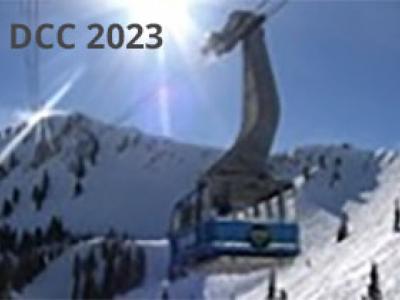
- Read more about Txt2Vid-Web: Web-based, Text-to-Video, Video Conferencing Pipeline
- Log in to post comments
Video conferencing tools have seen a significant increase in usage in the past few years but they still consume a significant bandwidth of ~100 Kbps to a few Mbps. In this work, we present Txt2Vid-Web: a practical, web-based, low bandwidth, video conferencing platform building upon the Txt2Vid work [1].
- Categories:
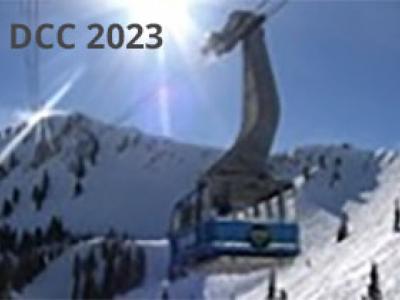
- Read more about Invert-and-project (IVP)-A Lossless Compression Method of Multi-scale JPEG Images via DCT Coefficients Prediction
- Log in to post comments
JPEG is a versatile and widely used format for images. Based an elegant design that enables the joint works of basis transformation (gross-scale decorrelation) and entropy coding (fine-scale coding), the resulting JPEG image can maintain virtually all visible features of an image while reducing its size to one tens of the original raw data.
- Categories:
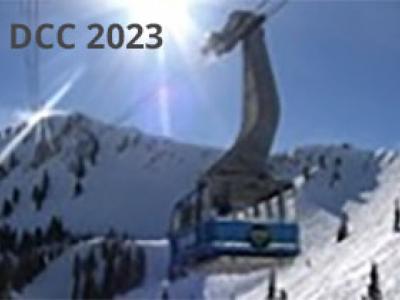
This paper studies compression techniques for parallel in-memory sparse tensor algebra. We find that applying simple existing compression schemes can lead to performance loss in some cases. To resolve this issue, we introduce an optimized algorithm for processing compressed inputs that can improve both the space usage as well as the performance compared to uncompressed inputs. We implement the compression techniques on top of a suite of sparse matrix algorithms generated by taco, a compiler for sparse tensor algebra.
dcc-23.pdf

- Categories:
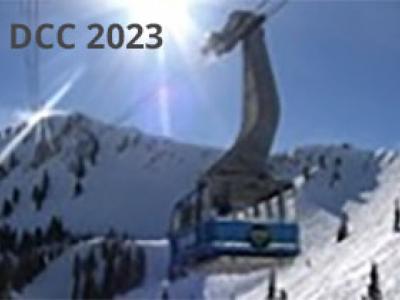
- Read more about Pixel-Wise Quantization for Image Compression
- Log in to post comments
Since the distortions on simple pixels are more noticeable than those on complex pixels, this paper proposes a pixel-wise quantization method, which allows to reduce the quantization parameters of simple pixels adaptively for the purpose of enhancing the subjective quality, and no additional syntax need to be transmitted in the bitstream. The discrimination of simple pixels is based on the texture complexity of the neighboring reconstruction pixels. The reduction of quantization parameters is related to the just noticeable difference for the human visual system.
- Categories:
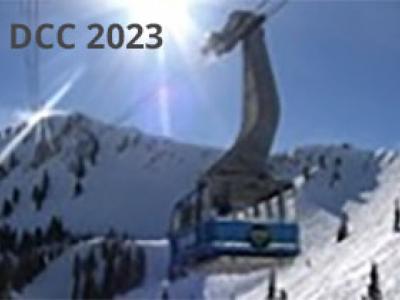
- Read more about Long-distance Information Filtering Network for Compressed Video Quality Enhancement
- Log in to post comments
Restoring high-quality videos from low-quality compressed ones is a crucial research topic in video coding. Most existing methods do not exploit the information in the long-distance compressed frames. Even when they do, these methods ignore the effect of interference information during reconstruction. In this paper, we propose a unique Long-distance Information Filtering (LIF) scheme with the 3D-CNN, which enhances compressed videos by mining filtered and valid information from long-distance frames.
- Categories: