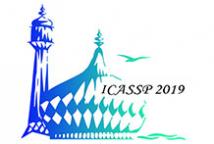
ICASSP is the world’s largest and most comprehensive technical conference focused on signal processing and its applications. The 2019 conference will feature world-class presentations by internationally renowned speakers, cutting-edge session topics and provide a fantastic opportunity to network with like-minded professionals from around the world. Visit website.
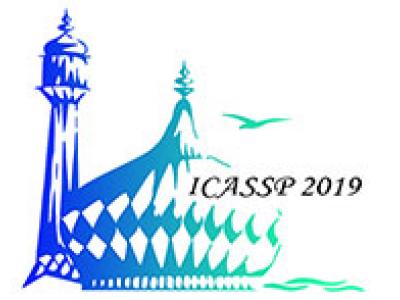
- Read more about Federated Learning for Keyword Spotting
- 1 comment
- Log in to post comments
Link to original article https://ieeexplore.ieee.org/document/8683546
poster.pdf

- Categories:
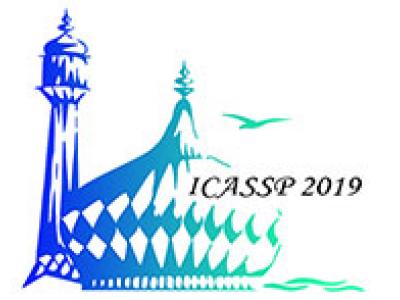
- Read more about ITERATIVELYREWEIGHTEDPENALTYALTERNATINGMINIMIZATIONMETHODS WITHCONTINUATIONFORIMAGEDEBLURRING
- Log in to post comments
- Categories:
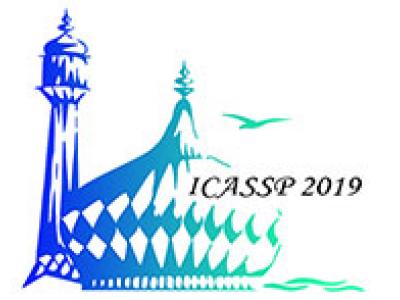
- Read more about ITERATIVELYREWEIGHTEDPENALTYALTERNATINGMINIMIZATIONMETHODS WITHCONTINUATIONFORIMAGEDEBLURRING
- Log in to post comments
- Categories:
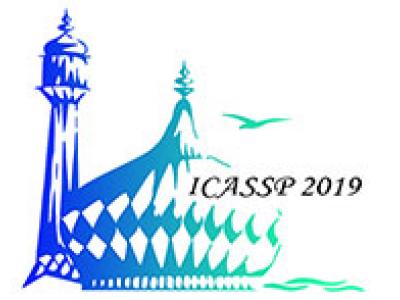
This work considers the problem of approximating continuous functions of finite Dirichlet energy from samples of these functions. It will be shown that there exists no sampling-based method which is able to approximate every function in this space from its samples. Specifically, we show that for any sampling based approximation method, the energy of the approximation tends to infinity as the number of samples is increased for almost every continuous function of finite energy. As an application, we study the problem of solving the Dirichlet problem on a bounded region.
- Categories:
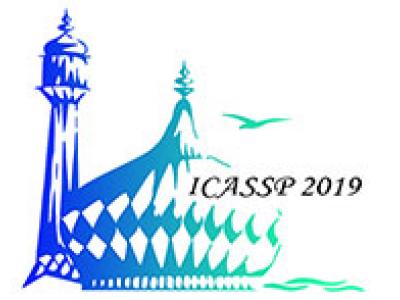
- Read more about DNN-BASED SPEAKER-ADAPTIVE POSTFILTERING WITH LIMITED ADAPTATION DATA FOR STATISTICAL SPEECH SYNTHESIS SYSTEMS
- Log in to post comments
Deep neural networks (DNNs) have been successfully deployed for acoustic modelling in statistical parametric speech synthesis (SPSS) systems. Moreover, DNN-based postfilters (PF) have also been shown to outperform conventional postfilters that are widely used in SPSS systems for increasing the quality of synthesized speech. However, existing DNN-based postfilters are trained with speaker-dependent databases. Given that SPSS systems can rapidly adapt to new speakers from generic models, there is a need for DNN-based postfilters that can adapt to new speakers with minimal adaptation data.
- Categories:
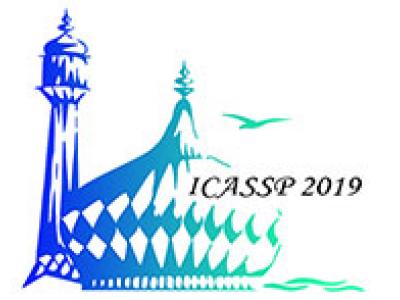
- Read more about ITERATIVELYREWEIGHTEDPENALTYALTERNATINGMINIMIZATIONMETHODS WITHCONTINUATIONFORIMAGEDEBLURRING
- Log in to post comments
- Categories:
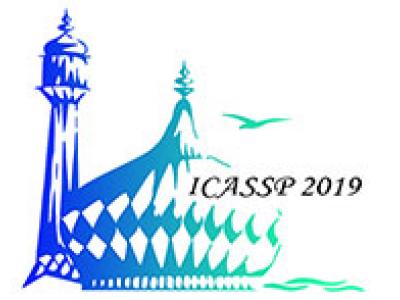
- Read more about End-to-End Sound Source Separation Conditioned On Instrument Labels
- Log in to post comments
Can we perform an end-to-end music source separation with a variable number of sources using a deep learning model? We present an extension of the Wave-U-Net model which allows end-to-end monaural source separation with a non-fixed number of sources. Furthermore, we propose multiplicative conditioning with instrument labels at the bottleneck of the Wave-U-Net and show its effect on the separation results. This approach leads to other types of conditioning such as audio-visual source separation and score-informed source separation.
ICASSP2019.pdf

- Categories:
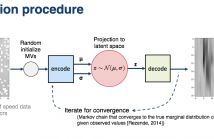
- Read more about Missing Data In Traffic Estimation: A Variational Autoencoder Imputation Method
- Log in to post comments
Road traffic forecasting systems are in scenarios where sensor or system failure occur. In those scenarios, it is known that missing values negatively affect estimation accuracy although it is being often underestimate in current deep neural network approaches. Our assumption is that traffic data can be generated from a latent space. Thus, we propose an online unsupervised data imputation method based on learning the data distribution using a variational autoencoder (VAE).
- Categories:
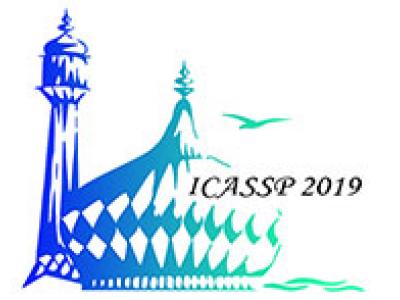
- Read more about TS-MC: Two stage matrix completion algorithm for Wireless sensor networks
- Log in to post comments
- Categories:
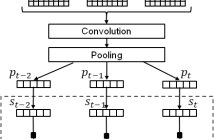
- Read more about Context-aware Neural-based Dialog Act Classification On Automatically Generated Transcriptions
- Log in to post comments
This paper presents our latest investigations on dialog act (DA) classification on automatically generated transcriptions. We propose a novel approach that combines convolutional neural networks (CNNs) and conditional random fields (CRFs) for context modeling in DA classification. We explore the impact of transcriptions generated from different automatic speech recognition systems such as hybrid TDNN/HMM and End-to-End systems on the final performance. Experimental results on two benchmark datasets (MRDA and SwDA) show that the combination CNN and CRF improves consistently the accuracy.
- Categories: