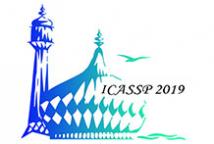
ICASSP is the world’s largest and most comprehensive technical conference focused on signal processing and its applications. The 2019 conference will feature world-class presentations by internationally renowned speakers, cutting-edge session topics and provide a fantastic opportunity to network with like-minded professionals from around the world. Visit website.
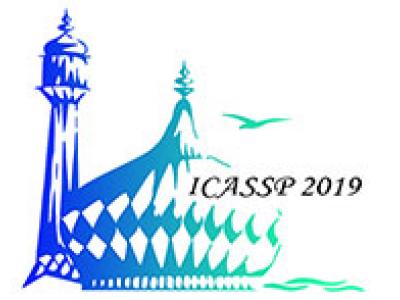
- Read more about E-CNN: Accurate Spherical Camera Rotation Estimation via Uniformization of Distorted Optical Flow Fields
- Log in to post comments
Spherical cameras, which can acquire all-round information, are effective to estimate rotation for robotic applications. Recently, Convolutional Neural Networks have shown great robustness in solving such regression problems. However they are designed for planar images and cannot deal with the non-uniform distortion present in spherical images, when expressed in the planar equirectangular projection. This can lower the accuracy of motion estimation. In this research, we propose an Equirectangular-Convolutional Neural Network (E-CNN) to solve this issue.
- Categories:
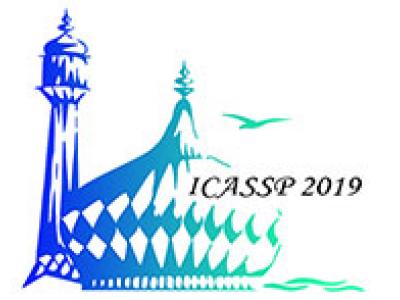
- Read more about Pairwise Approximate K-SVD
- Log in to post comments
Pairwise, or separable, dictionaries are suited for the sparse representation of 2D signals in their original form, without vectorization. They are equivalent with enforcing a Kronecker structure on a standard dictionary for 1D signals. We present a dictionary learning algorithm, in the coordinate descent style of Approximate K-SVD, for such dictionaries. The algorithm has the benefit of extremely low complexity, clearly lower than that of existing algorithms.
- Categories:
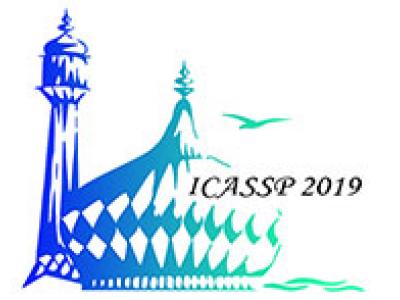
- Read more about Learning Dynamic Stream Weights for Linear Dynamical Systems using Natural Evolution Strategies
- Log in to post comments
Multimodal data fusion is an important aspect of many object localization and tracking frameworks that rely on sensory observations from different sources. A prominent example is audiovisual speaker localization, where the incorporation of visual information has shown to benefit overall performance, especially in adverse acoustic conditions. Recently, the notion of dynamic stream weights as an efficient data fusion technique has been introduced into this field.
- Categories:
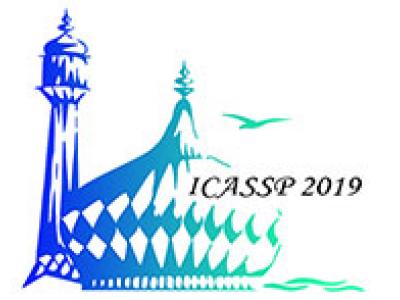
- Read more about Making Decisions with Shuffled Bits
- Log in to post comments
mwicassp19.pdf

- Categories:
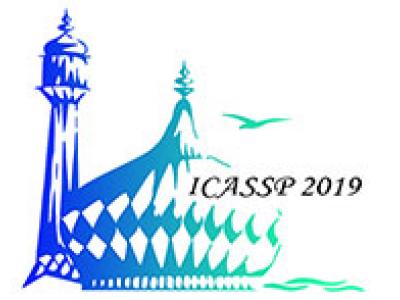
- Read more about JSR-NET: A DEEP NETWORK FOR JOINT SPATIAL-RADON DOMAIN CT RECON- STRUCTION FROM INCOMPLETE DATA
- Log in to post comments
CT image reconstruction from incomplete data, such as sparse views and limited angle reconstruction, is an important and challenging problem in medical imaging. This work proposes a new deep convolutional neural network (CNN), called JSR-Net, that jointly reconstructs CT images and their associated Radon domain projections. JSR-Net combines the traditional model-based approach with deep architecture design of deep learning. A hybrid loss function is adapted to improve the performance of the JSR-Net making it more effective in protecting important image structures.
- Categories:
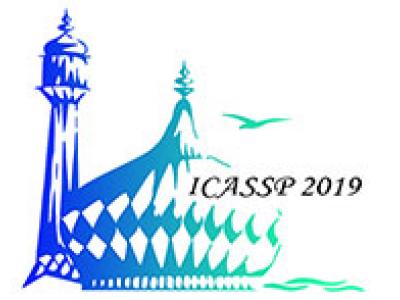
- Read more about Investigations of real-time Gaussian FFTNet and parallel WaveNet neural vocoders with simple acoustic features
- Log in to post comments
This paper examines four approaches to improving real-time neural vocoders with simple acoustic features (SAF) constructed from fundamental frequency and mel-cepstra rather than mel-spectrograms.
- Categories:
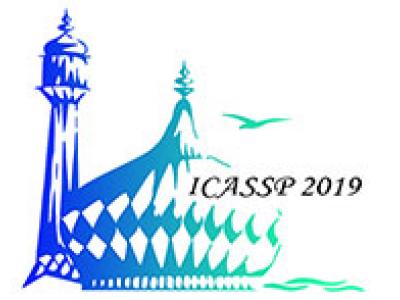
- Read more about Horizontal 3D sound field recording and 2.5D synthesis with omni-directional circular arrays
- Log in to post comments
Although 2.5D sound field synthesis with a circular loudspeaker array can be used in a 3D sound field, a 2D sound field, instead of a 3D sound field, is assumed for a sound field recording with a circular microphone array. This paper presents a horizontal 3D sound field recording and 2.5D synthesis method used in 3D sound fields with multiple co-centered omni-directional circular microphone arrays and a circular loudspeaker array without vertical derivative measurements.
- Categories:
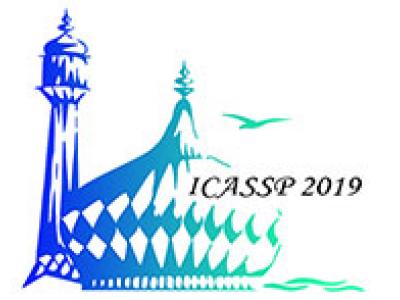
- Read more about CycleGAN-VC2: Improved CycleGAN-based Non-parallel Voice Conversion
- Log in to post comments
Non-parallel voice conversion (VC) is a technique for learning the mapping from source to target speech without relying on parallel data. This is an important task, but it has been challenging due to the disadvantages of the training conditions. Recently, CycleGAN-VC has provided a breakthrough and performed comparably to a parallel VC method without relying on any extra data, modules, or time alignment procedures. However, there is still a large gap between the real target and converted speech, and bridging this gap remains a challenge.
- Categories:
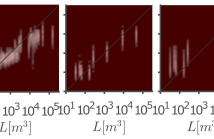
- Read more about Blind Room Volume Estimation from Single-Channel Noisy Speech
- Log in to post comments
Recent work on acoustic parameter estimation indicates that geometric room volume can be useful for modeling the character of an acoustic environment. However, estimating volume from audio signals remains a challenging problem. Here we propose using a convolutional neural network model to estimate the room volume blindly from reverberant single-channel speech signals in the presence of noise. The model is shown to produce estimates within approximately a factor of two to the true value, for rooms ranging in size from small offices to large concert halls.
- Categories:
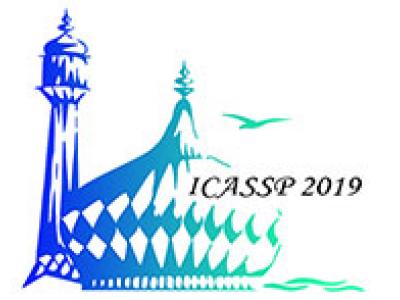
- Read more about A Learning Approach for Wavelet Design
- Log in to post comments
Wavelet analysis and perfect reconstruction filterbanks (PRFBs) are closely related. Desired properties on the wavelet could be translated to equivalent properties on a PRFB. We propose a new learning-based approach towards designing compactly supported orthonormal wavelets with a specified number of vanishing moments. We view PRFBs as a special class of convolutional autoencoders, which places the problem of wavelet/PRFB design within a learning framework. One could then deploy several state-of-the-art deep learning tools to solve the design problem.
- Categories: