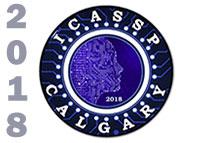
ICASSP is the world’s largest and most comprehensive technical conference focused on signal processing and its applications. The 2019 conference will feature world-class presentations by internationally renowned speakers, cutting-edge session topics and provide a fantastic opportunity to network with like-minded professionals from around the world. Visit website.
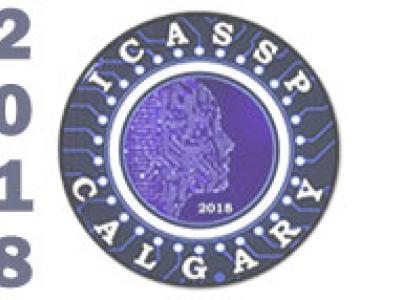
- Read more about MONAURAL SINGING VOICE SEPARATION WITH SKIP-FILTERING CONNECTIONS AND RECURRENT INFERENCE OF TIME-FREQUENCY MASK
- Log in to post comments
Singing voice separation based on deep learning relies on the usage of time-frequency masking. In many cases the masking process is not a learnable function or is not encapsulated into the deep learning optimization. Consequently, most of the existing methods rely on a post processing step using the generalized Wiener filtering. This work proposes a method that learns and optimizes (during training) a source-dependent mask and does not need the aforementioned post processing step.
- Categories:
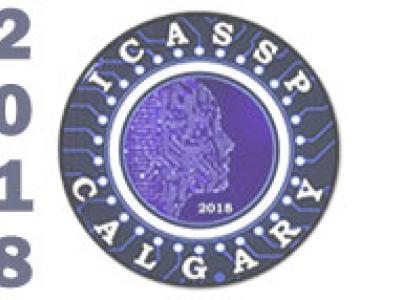
- Read more about Insights into End-to-End Learning Scheme for Language Identification
- Log in to post comments
A novel interpretable end-to-end learning scheme for language identification is proposed. It is in line with the classical GMM i-vector methods both theoretically and practically. In the end-to-end pipeline, a general encoding layer is employed on top of the front-end CNN, so that it can encode the variable-length input sequence into an utterance level vector automatically. After comparing with the state-of-the-art GMM i-vector methods, we give insights into CNN, and reveal its role and effect in the whole pipeline.
- Categories:
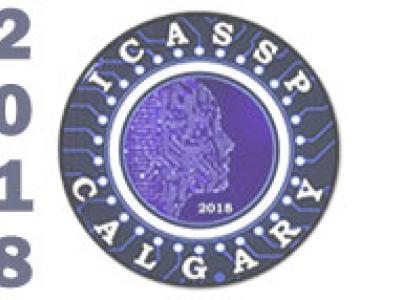
- Read more about DISTRIBUTED OPTIMAL CONSENSUS-BASED KALMAN FILTERING AND ITS RELATION TO MAP ESTIMATION
- Log in to post comments
In this paper, we address the problem of distributed state estimation, where a set of nodes are required to jointly estimate the state of a linear dynamic system based on sequential measurements. In our distributed scenario, all the nodes 1) are interested in the full state of the observed system and 2) pursue a consensus-based state estimate with high accuracy. We exploit the equivalent relation between the maximum-a-posteriori (MAP) estimation and the Kalman filter (KF) in the minimum mean square error (MMSE) sense under the Gaussian assumption.
- Categories:
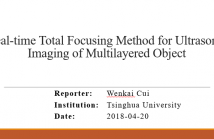
- Read more about REAL-TIME TOTAL FOCUSING METHOD FOR ULTRASONIC IMAGING OF MULTILAYERED OBJECT
- Log in to post comments
Lecture.pptx

- Categories:
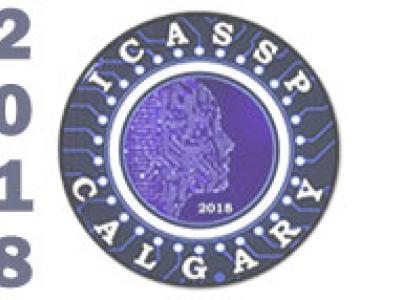
- Read more about SUPER WIDE REGRESSION NETWORK FOR UNSUPERVISED CROSS-DATABASE FACIAL EXPRESSION RECOGNITION
- Log in to post comments
Unsupervised cross-database facial expression recognition(FER) is a challenging problem, in which the training and testing samples belong to different facial expression databases. For this reason, the training (source) and testing (target) facial expression samples would have different feature distributions and hence the performance of lots of existing FER methods may decrease.
- Categories:
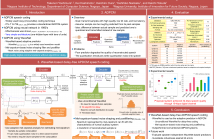
- Read more about REAL-TIME TOTAL FOCUSING METHOD IMAGING FOR ULTRASONIC INSPECTION OF THREE-DIMENSIONAL MULTILAYERED MEDIA
- Log in to post comments
Poster.pdf

- Categories:
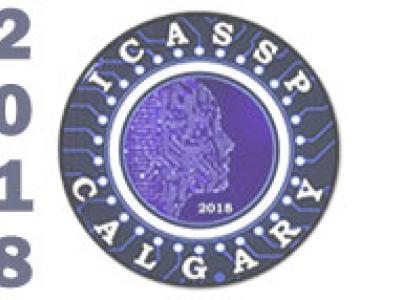
- Read more about Efficient Estimation of Scatter Matrix with Convex Structure under t-distribution
- Log in to post comments
This paper addresses structured covariance matrix estimation under t-distribution. Covariance matrices frequently reveal a particular structure due to the considered application and taking into account this structure usually improves estimation accuracy. In the framework of robust estimation, the $t$-distribution is particularly suited to describe heavy-tailed observation. In this context, we propose an efficient estimation procedure for covariance matrices with convex structure under t-distribution.
- Categories:
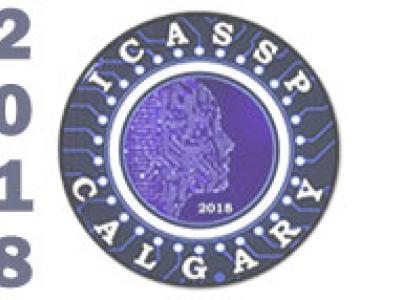
- Read more about UNSUPERVISED CROSS-CORPUS SPEECH EMOTION RECOGNITION USING DOMAIN-ADAPTIVE SUBSPACE LEARNING
- Log in to post comments
In this paper, we investigate an interesting problem, i.e., unsupervised cross-corpus speech emotion recognition (SER), in which the training and testing speech signals come from two different speech emotion corpora. Meanwhile, the training speech signals are labeled, while the label information of the testing speech signals is entirely unknown. Due to this setting, the training (source) and testing (target) speech signals may have different feature distributions and therefore lots of existing SER methods would not work.
- Categories:
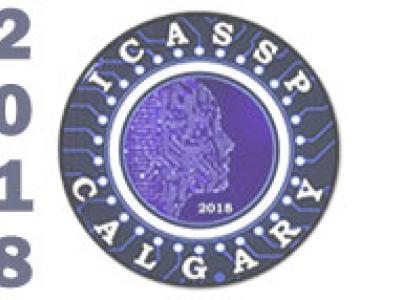
- Read more about A compressive sensing-based active user and symbol detection for massive machine type communications
- Log in to post comments
- Categories:
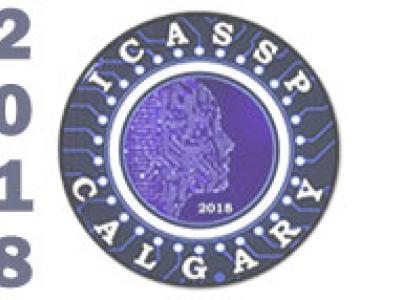
- Read more about UNSUPERVISED CROSS-CORPUS SPEECH EMOTION RECOGNITION USING DOMAIN-ADAPTIVE SUBSPACE LEARNING
- Log in to post comments
- Categories: