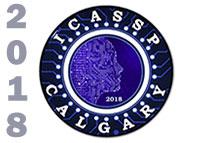
ICASSP is the world’s largest and most comprehensive technical conference focused on signal processing and its applications. The 2019 conference will feature world-class presentations by internationally renowned speakers, cutting-edge session topics and provide a fantastic opportunity to network with like-minded professionals from around the world. Visit website.

- Read more about HAND-RAISING GESTURE DETECTION IN REAL CLASSROOM
- Log in to post comments
- Categories:
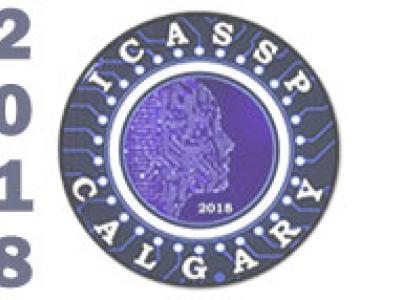
- Read more about Cognitive Analysis of Working Memory Load from EEG, by a Deep Recurrent Neural Network
- Log in to post comments
One of the common modalities for observing mental activity is electroencephalogram (EEG) signals. However, EEG recording is highly susceptible to various sources of noise and to inter-subject differences. In order to solve these problems, we present a deep recurrent neural network (RNN) architecture to learn robust features and predict the levels of the cognitive load from EEG recordings. Using a deep learning approach, we first transform the EEG time series into a sequence of multispectral images which carries spatial information.
Kuanar_ICASSP-2018.pdf

- Categories:
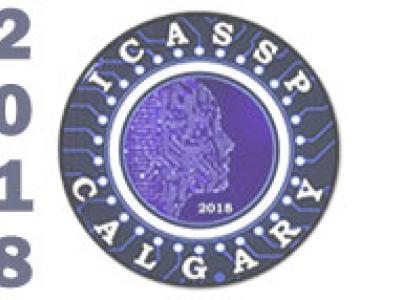
- Read more about CONVOLUTIONAL NEURAL NETWORK APPROACH FOR EEG-BASED EMOTION RECOGNITION USING BRAIN CONNECTIVITY AND ITS SPATIAL INFORMATION
- Log in to post comments
Emotion recognition based on electroencephalography (EEG) has received attention as a way to implement human-centric
services. However, there is still much room for improvement, particularly in terms of the recognition accuracy. In this paper, we propose a novel deep learning approach using convolutional neural networks (CNNs) for EEG-based emotion recognition. In particular, we employ brain connectivity features that have not been used with deep learning models in
- Categories:
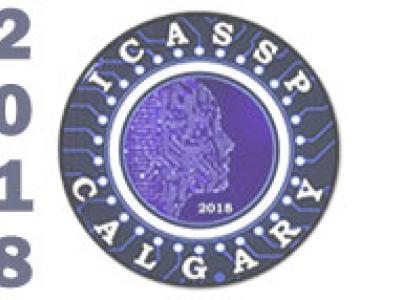
A large-scale video quality dataset called the VideoSet has been constructed recently to measure human subjective experience of H.264 coded video in terms of the just-noticeable-difference (JND). It measures the first three JND points of 5-second video of resolution 1080p, 720p, 540p and 360p. Based on the VideoSet, we propose a method to predict the satisfied-user-ratio (SUR) curves using a machine learning framework. First, we partition a video clip into local spatial-temporal segments and evaluate the quality of each seg- ment using the VMAF quality index.
- Categories:
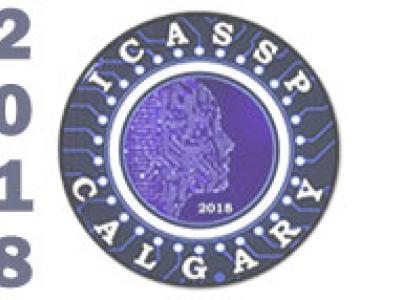
- Read more about EEG-BASED VIDEO IDENTIFICATION USING GRAPH SIGNAL MODELING AND GRAPH CONVOLUTIONAL NEURAL NETWORK
- Log in to post comments
This paper proposes a novel graph signal-based deep learning method for electroencephalography (EEG) and its application to EEG-based video identification. We present new methods to effectively represent EEG data as signals on graphs, and learn them using graph convolutional neural networks. Experimental results for video identification using EEG responses obtained while watching videos show the effectiveness of the proposed approach in comparison to existing methods. Effective schemes for graph signal representation of EEG are also discussed.
- Categories:
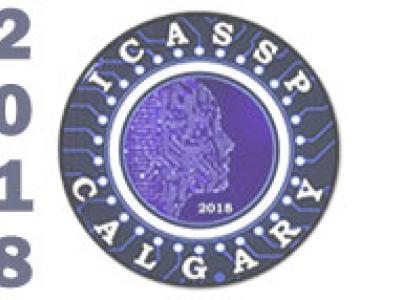
- Read more about MODELING-BY-GENERATION-STRUCTURED NOISE COMPENSATION ALGORITHM FOR GLOTTAL VOCODING SPEECH SYNTHESIS SYSTEM
- Log in to post comments
This paper proposes a novel noise compensation algorithm for a glottal excitation model in a deep learning (DL)-based speech synthesis system.
To generate high-quality speech synthesis outputs, the balance between harmonic and noise components of the glottal excitation signal should be well-represented by the DL network.
However, it is hard to accurately model the noise component because the DL training process inevitably results in statistically smoothed outputs; thus, it is essential to introduce an additional noise compensation process.
- Categories:
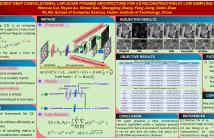
- Read more about An efficient deep convolutional laplacian pyramid architecture for CS reconstruction at low sampling ratios
- Log in to post comments
The compressed sensing (CS) has been successfully applied to image compression in the past few years as most image signals are sparse in a certain domain. Several CS reconstruction models have been proposed and obtained superior performance. However, these methods suffer from blocking artifacts or ringing effects at low sampling ratios in most cases. To address this problem, we propose a deep convolutional Laplacian Pyramid Compressed Sensing Network (LapCSNet) for CS, which consists of a sampling sub-network and a reconstruction sub-network.
- Categories:
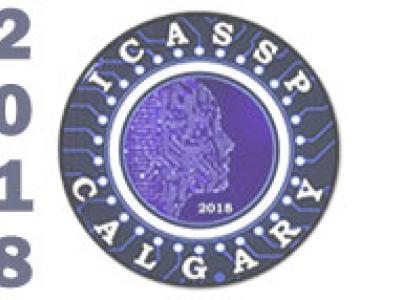
- Read more about PhaseSplit: A Variable Splitting Framework for Phase Retrieval
- Log in to post comments
We develop two techniques based on alternating minimization and
alternating directions method of multipliers for phase retrieval (PR)
by employing a variable-splitting approach in a maximum likelihood
estimation framework. This leads to an additional equality constraint,
which is incorporated in the optimization framework using a
quadratic penalty. Both algorithms are iterative, wherein the updates
are computed in closed-form. Experimental results show that: (i)
the proposed techniques converge faster than the state-of-the-art PR
- Categories:
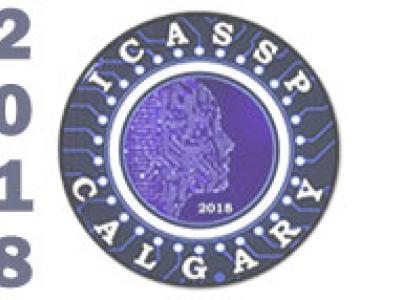
- Read more about A Novel Selective Active Noise Control Algorithm to Overcome Practical Implementation Issue
- Log in to post comments
Selective active noise control (SANC) is a method to select a pre-trained control filter for different primary noises, instead of using conventional real-time computation of the
control filter coefficients. SANC has the advantage of improving the robustness of control filter while reducing the
computational complexity. This paper presents a practical
strategy in choosing a suitable control filter based on the
frequency-band-match mechanism implemented in a partitioned frequency domain filter structure. Both simulation and
- Categories:
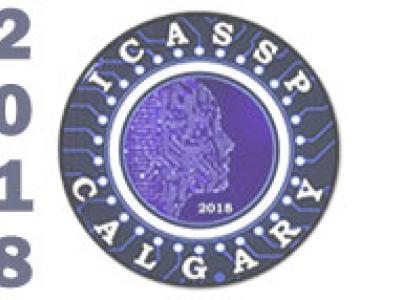
- Read more about Distributed Censoring with Energy Constraint in Wireless Sensor Networks
- Log in to post comments
In wireless sensor networks (WSNs), energy is always precious for sensor nodes. To save energy, censoring is introduced to cut the total number of transmission by only transmitting informative data. This algorithm, however, ignores the energy consumption during the delivery of parameters, which can be significant comparing to the saved power. In this paper, we consider the adaptive censoring from the energy perspective. A distributed censoring algorithm with energy constraint is developed that allows sensor nodes to make autonomous
- Categories: