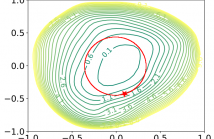
- Read more about BRINGING THE DISCUSSION OF MINIMA SHARPNESS TO THE AUDIO DOMAIN: A FILTER-NORMALISED EVALUATION FOR ACOUSTIC SCENE CLASSIFICATION
- Log in to post comments
The correlation between the sharpness of loss minima and generalisation in the context of deep neural networks has been subject to discussion for a long time. Whilst mostly investigated in the context of selected benchmark data sets in the area of computer vision, we explore this aspect for the acoustic scene classification task of the DCASE2020 challenge data. Our analysis is based on two-dimensional filter-normalised visualisations and a derived sharpness measure.
- Categories:
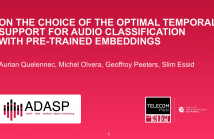
- Read more about ON THE CHOICE OF THE OPTIMAL TEMPORAL SUPPORT FOR AUDIO CLASSIFICATION WITH PRE-TRAINED EMBEDDINGS
- Log in to post comments
Current state-of-the-art audio analysis systems rely on pre-trained embedding models, often used off-the-shelf as (frozen) feature extractors. Choosing the best one for a set of tasks is the subject of many recent publications. However, one aspect often overlooked in these works is the influence of the duration of audio input considered to extract an embedding, which we refer to as Temporal Support (TS). In this work, we study the influence of the TS for well-established or emerging pre-trained embeddings, chosen to represent different types of architectures and learning paradigms.
- Categories:
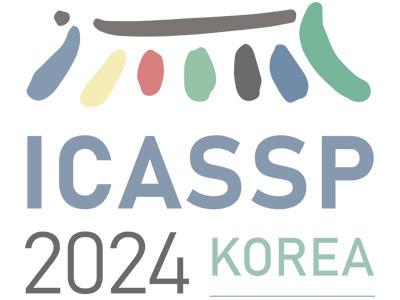
- Read more about LEARNING FROM TAXONOMY: MULTI-LABEL FEW-SHOT CLASSIFICATION FOR EVERYDAY SOUND RECOGNITION
- 1 comment
- Log in to post comments
Humans categorise and structure perceived acoustic signals into hierarchies of auditory objects. The semantics of these objects are thus informative in sound classification, especially in few-shot scenarios. However, existing works have only represented audio semantics as binary labels (e.g., whether a recording contains \textit{dog barking} or not), and thus failed to learn a more generic semantic relationship among labels. In this work, we introduce an ontology-aware framework to train multi-label few-shot audio networks with both relative and absolute relationships in an audio taxonomy.
- Categories:
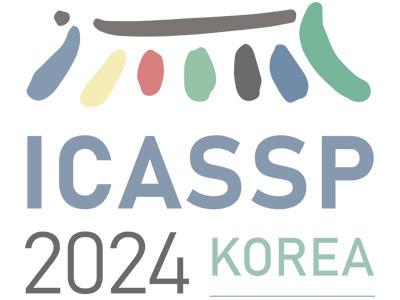
- Read more about ESVC: Combining Adaptive Style Fusion and Multi-Level Feature Disentanglement for Expressive Singing Voice Conversion
- Log in to post comments
Nowadays, singing voice conversion (SVC) has made great strides in both naturalness and similarity for common SVC with a neutral expression. However, besides singer identity, emotional expression is also essential to convey the singer's emotions and attitudes, but current SVC systems can not effectively support it. In this paper, we propose an expressive SVC framework called ESVC, which can convert singer identity and emotional style simultaneously.
- Categories:
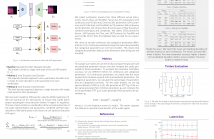
- Read more about Exploring Approaches to Multi-Task Automatic Synthesizer Programming
- Log in to post comments
Automatic Synthesizer Programming is the task of transforming an audio signal that was generated from a virtual instrument, into the parameters of a sound synthesizer that would generate this signal. In the past, this could only be done for one virtual instrument. In this paper, we expand the current literature by exploring approaches to automatic synthesizer programming for multiple virtual instruments. Two different approaches to multi-task automatic synthesizer programming are presented. We find that the joint-decoder approach performs best.
- Categories:
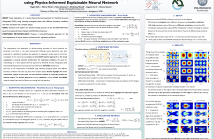
- Read more about POSTER - Grad-CAM-Inspired Interpretation of Nearfield Acoustic Holography using Physics-Informed Explainable Neural Network
- Log in to post comments
The interpretation and explanation of decision-making processes of neural networks are becoming a key factor in the deep learning field. Although several approaches have been presented for classification problems, the application to regression models needs to be further investigated. In this manuscript we propose a Grad-CAM-inspired approach for the visual explanation of neural network architecture for regression problems.
- Categories:
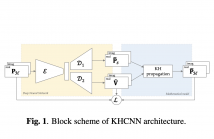
- Read more about PAPER - Grad-CAM-Inspired Interpretation of Nearfield Acoustic Holography using Physics-Informed Explainable Neural Network
- Log in to post comments
The interpretation and explanation of decision-making processes of neural networks are becoming a key factor in the deep learning field. Although several approaches have been presented for classification problems, the application to regression models needs to be further investigated. In this manuscript we propose a Grad-CAM-inspired approach for the visual explanation of neural network architecture for regression problems.
- Categories:
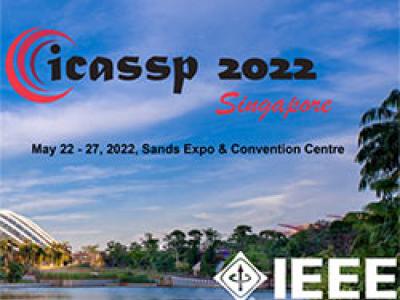
- Read more about PEER COLLABORATIVE LEARNING FOR POLYPHONIC SOUND EVENT DETECTION
- Log in to post comments
This paper describes how semi-supervised learning, called peer collaborative learning (PCL), can be applied to the polyphonic sound event detection (PSED) task, which is one of the tasks in the Detection and Classification of Acoustic Scenes and Events (DCASE) challenge. Many deep learning models have been studied to determine what kind of sound events occur where and for how long in a given audio clip.
- Categories:
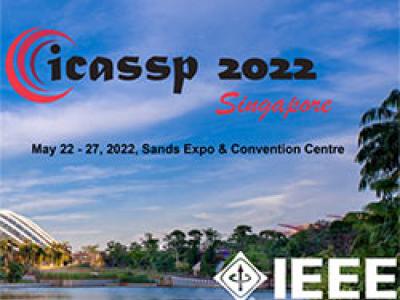
- Read more about Slides of Direct Noisy Speech Modeling for Noisy-to-Noisy Voice Conversion
- Log in to post comments
- Categories:
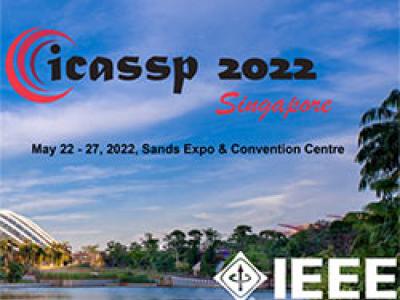
- Read more about Poster of Direct Noisy Speech Modeling for Noisy-to-Noisy Voice Conversion
- Log in to post comments
- Categories: