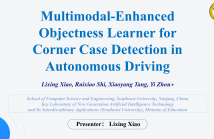
- Read more about Multimodal-Enhanced Objectness Learner for Corner Case Detection in Autonomous Driving
- 1 comment
- Log in to post comments
Previous works on object detection have achieved high accuracy in closed-set scenarios, but their performance in open-world scenarios is not satisfactory. One of the challenging open-world problems is corner case detection in autonomous driving. Existing detectors struggle with these cases, relying heavily on visual appearance and exhibiting poor generalization ability. In this paper, we propose a solution by reducing the discrepancy between known and unknown classes and introduce a multimodal-enhanced objectness notion learner.
- Categories:
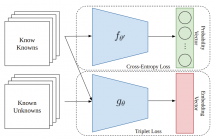
- Read more about CASCADING UNKNOWN DETECTION WITH KNOWN CLASSIFICATION FOR OPEN SET RECOGNITION
- Log in to post comments
Deep learners tend to perform well when trained under the closed set assumption but struggle when deployed under open set conditions. This motivates the field of Open Set Recognition in which we seek to give deep learners the ability to recognize whether a data sample belongs to the known classes trained on or comes from the surrounding infinite world. Existing open set recognition methods typically rely upon a single function for the dual task of distinguishing between knowns and unknowns as well as making known class distinction.
- Categories:
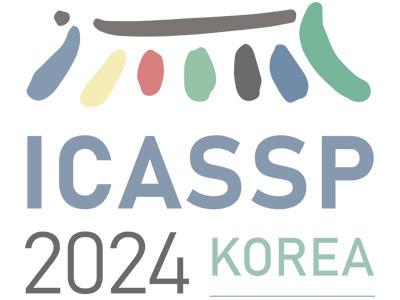
- Read more about TOWARDS MULTI-DOMAIN FACE LANDMARK DETECTION WITH SYNTHETIC DATA FROM DIFFUSION MODEL
- Log in to post comments
Recently, deep learning-based facial landmark detection for in-the-wild faces has achieved significant improvement. However, there are still challenges in face landmark detection in other domains (\eg{} cartoon, caricature, etc). This is due to the scarcity of extensively annotated training data. To tackle this concern, we design a two-stage training approach that effectively leverages limited datasets and the pre-trained diffusion model to obtain aligned pairs of landmarks and face in multiple domains.
- Categories:
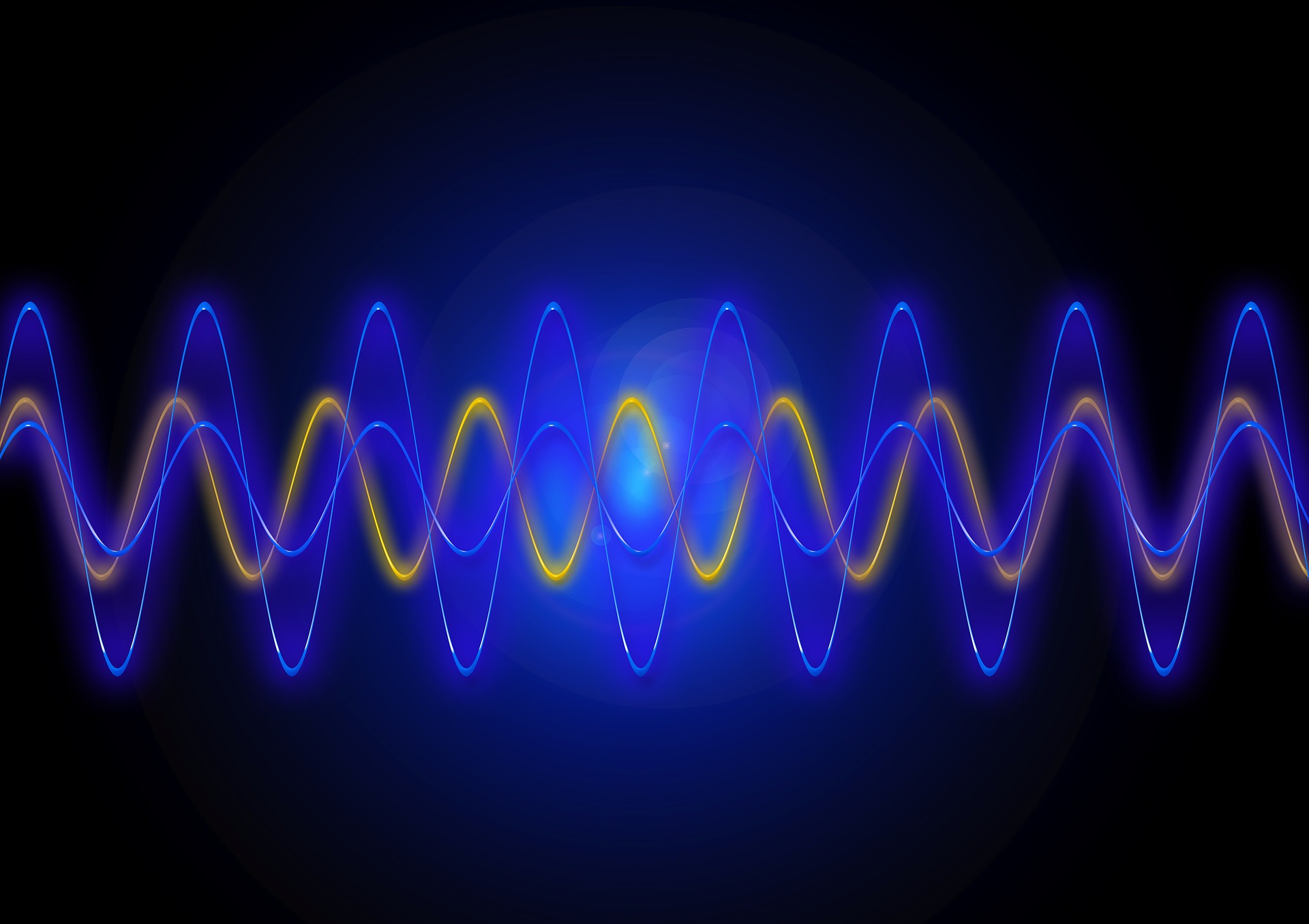
- Read more about CAG-FPN: CHANNEL SELF-ATTENTION GUIDED FEATURE PYRAMID NETWORK FOR OBJECT DETECTION
- 1 comment
- Log in to post comments
Feature Pyramid Network (FPN) plays a critical role and is indispensable for object detection methods. In recent years, attention mechanism has been utilized to improve FPN due to its excellent performance. Existing attention-based FPN methods generally work with a complex structure, resulting in an increase of computational costs. In view of this, we propose a novel Channel Self-Attention Guided Feature Pyramid Network (CAG-FPN), which not only has a simple structure but also consistently improves detection accuracy.
- Categories:
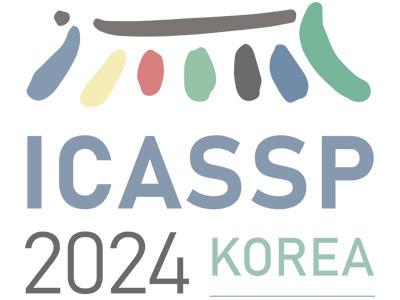
- Read more about A Parameterized Generative Adversarial Network Using Cyclic Projection for Explainable Medical Image Classification
- Log in to post comments
Although current data augmentation methods are successful to alleviate the data insufficiency, conventional augmentation are primarily intra-domain while advanced generative adversarial networks (GANs) generate images remaining uncertain, particularly in small-scale datasets. In this paper, we propose a parameterized GAN (ParaGAN) that effectively controls the changes of synthetic samples among domains and highlights the attention regions for downstream classification.
- Categories:
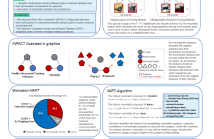
- Read more about DELVING DEEPER INTO VULNERABLE SAMPLES IN ADVERSARIAL TRAINING
- Log in to post comments
Recently, vulnerable samples have been shown to be crucial
for improving adversarial training performance. Our analysis
on existing vulnerable samples mining methods indicate that
existing methods have two problems: 1) valuable connections
among different pairs of natural samples and their adversarial
counterparts are ignored; 2) parts of vulnerable samples are
unconsidered. To better leverage vulnerable samples, we propose INter PAir ConstrainT (INPACT) and Vulnerable Aware
poster.pptx

- Categories:
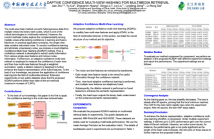
- Read more about ADAPTIVE CONFIDENCE MULTI-VIEW HASHING FOR MULTIMEDIA RETRIEVAL
- Log in to post comments
The multi-view hash method converts heterogeneous data from multiple views into binary hash codes, which is one of the critical technologies in multimedia retrieval. However, the current methods mainly explore the complementarity among multiple views while lacking confidence in learning and fusion. Moreover, in practical application scenarios, the single-view data contains redundant noise. To conduct confidence learning and eliminate unnecessary noise, we propose a novel Adaptive Confidence Multi-View Hashing (ACMVH) method.
- Categories:
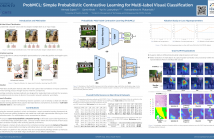
- Read more about PROBMCL: SIMPLE PROBABILISTIC CONTRASTIVE LEARNING FOR MULTI-LABEL VISUAL CLASSIFICATION
- Log in to post comments
Multi-label image classification presents a challenging task in many domains, including computer vision and medical imaging. Recent advancements have introduced graph-based and transformer-based methods to improve performance and capture label dependencies. However, these methods often include complex modules that entail heavy computation and lack interpretability. In this paper, we propose Probabilistic Multi-label Contrastive Learning (ProbMCL), a novel framework to address these challenges in multi-label image classification tasks.
- Categories:
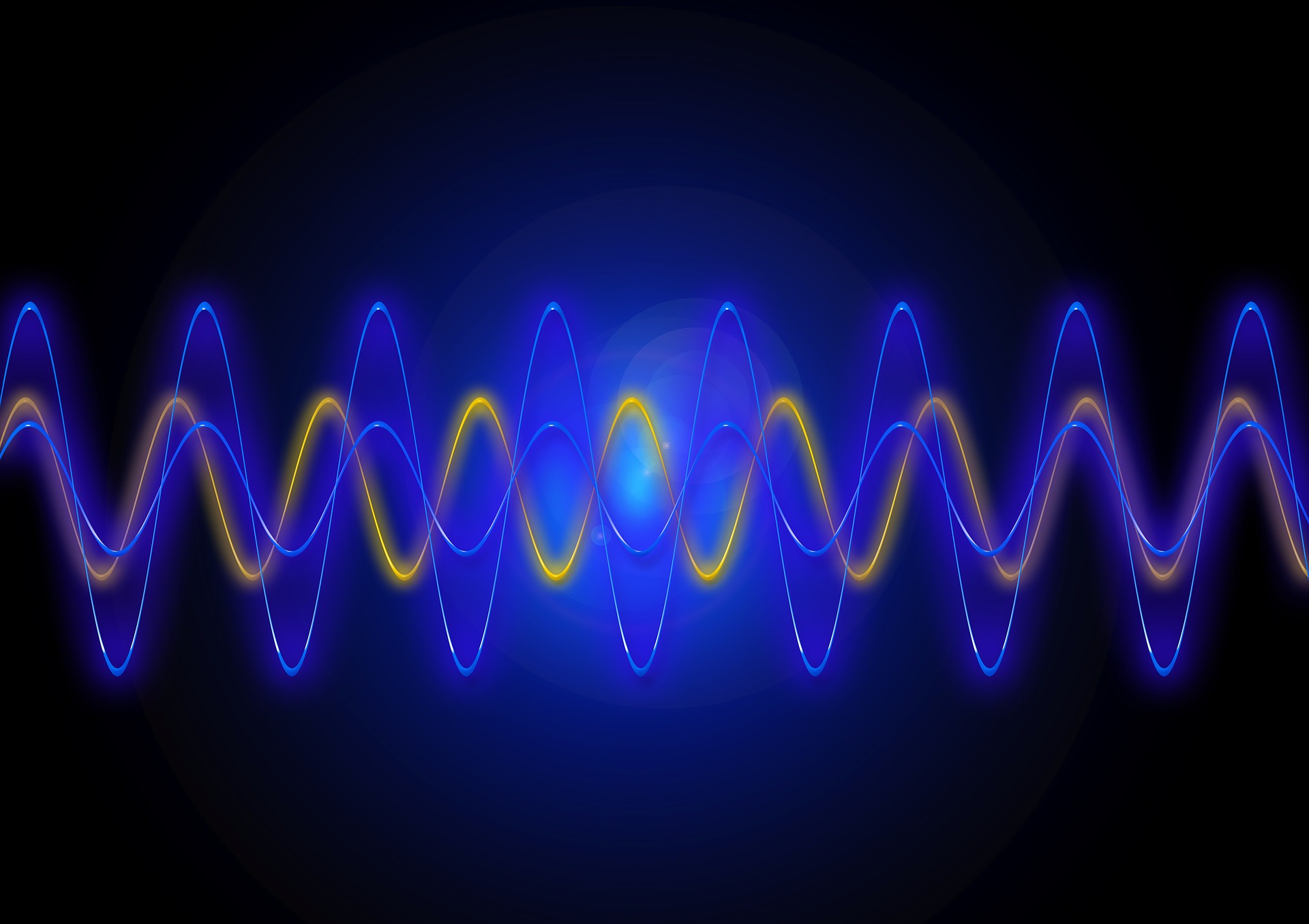
- Read more about A CONTRARIO PARADIGM FOR YOLO-BASED INFRARED SMALL TARGET DETECTION
- Log in to post comments
Detecting small to tiny targets in infrared images is a challenging task in computer vision, especially when it comes to differentiating these targets from noisy or textured backgrounds. Traditional object detection methods such as YOLO
- Categories:
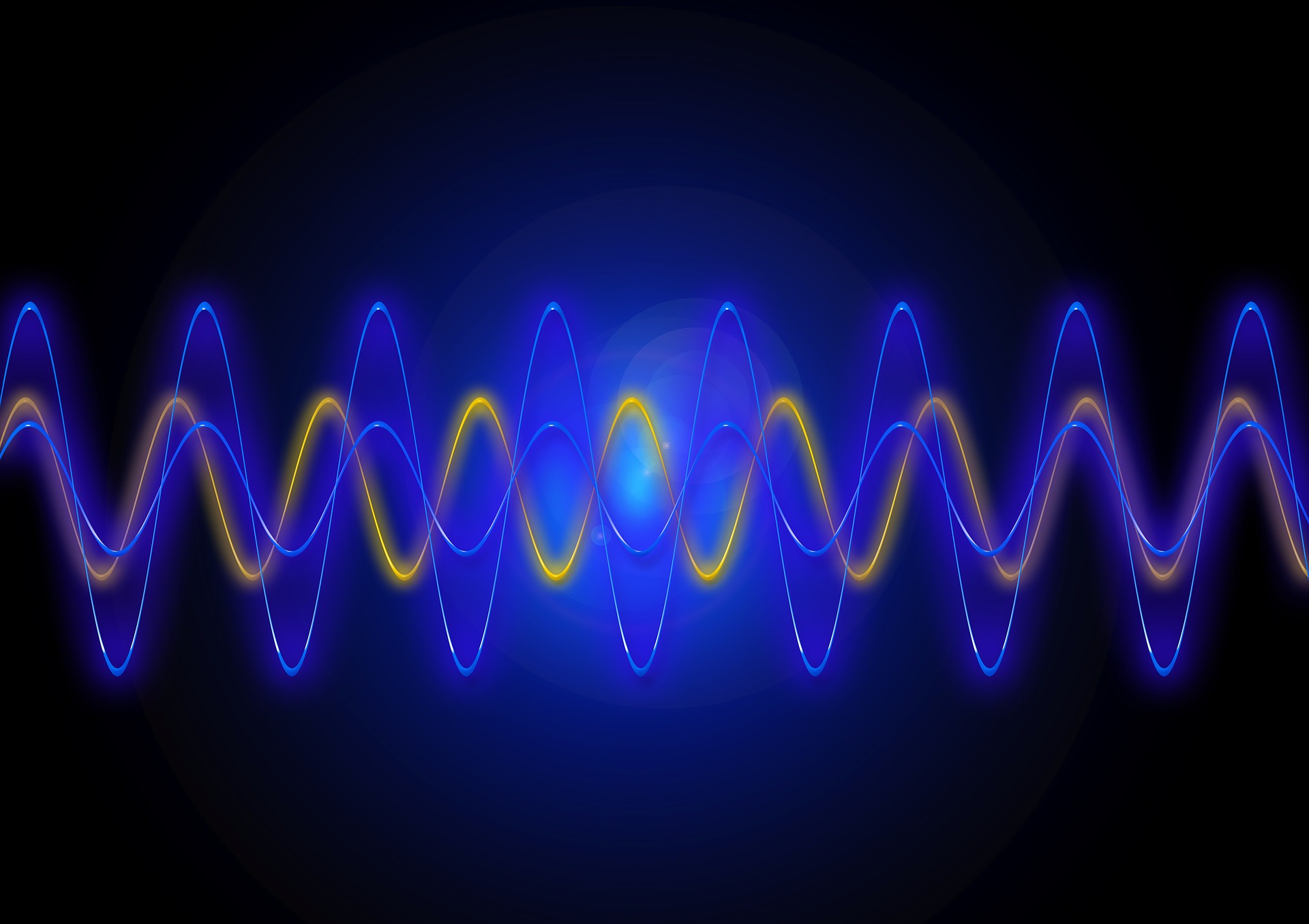
- Read more about 2D Human Pose Estimation Calibration and Keypoint Visibility Classification
- Log in to post comments
The confidence scores of 2D pose estimation are widely utilized in various fields, including multi-view 3D human pose estimation, skeleton-based human tracking, human action recognition, human re-identification, etc. Despite widespread use, confidence scores from 2D pose estimation methods are unreliable in indicating the accuracy of estimation results, particularly in occlusion situations, i.e., keypoints with high confidence scores may have low accuracy and vice versa. To address this issue, we propose a new 2D human pose estimation calibration method in this paper.
- Categories: