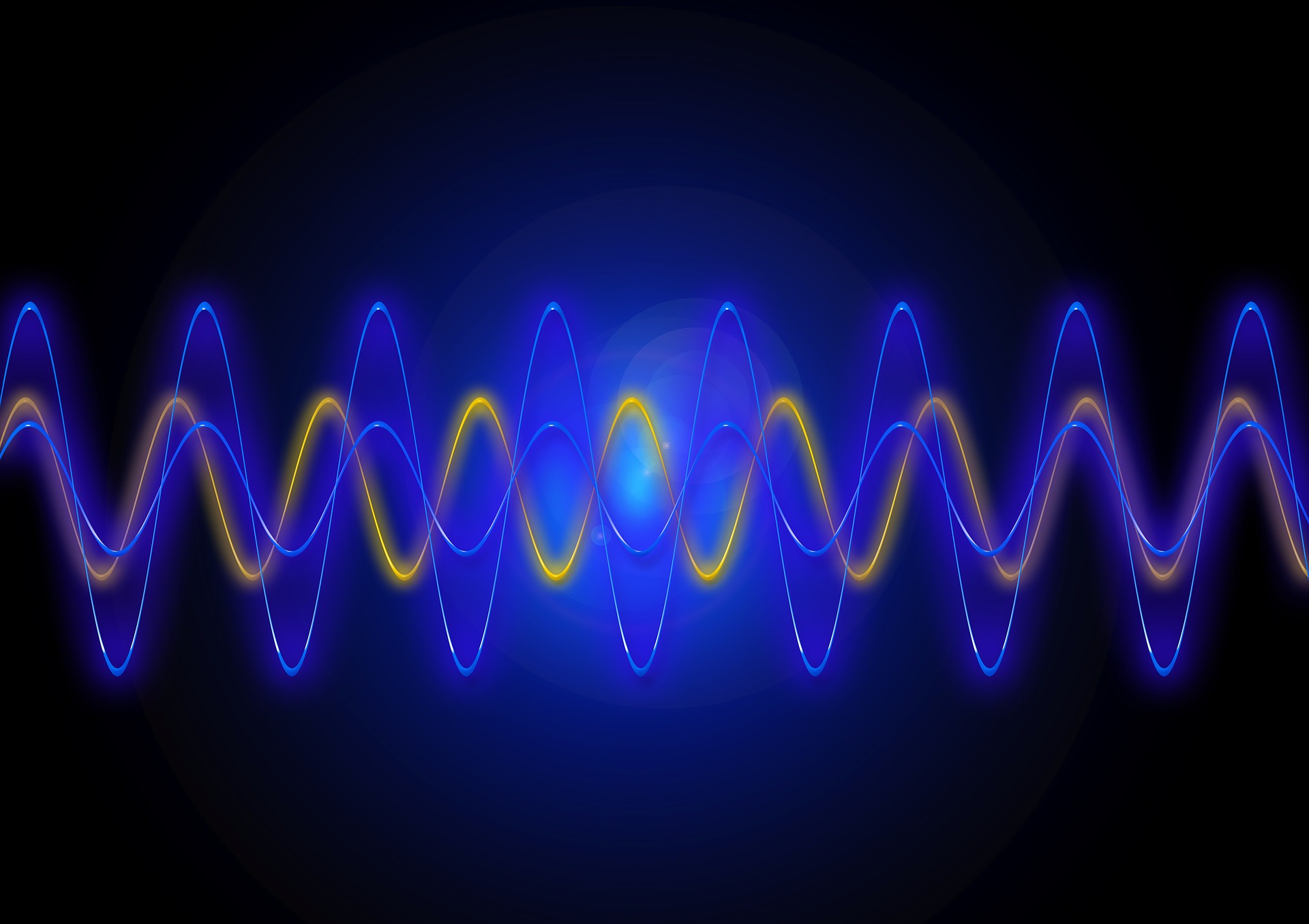
- Read more about Multivariate Fourier Distribution Perturbation: Domain Shifts with Uncertainty in Frequency Domain
- Log in to post comments
Diversifying training data techniques have achieved tremendous success in Domain Generalization (DG) tasks. The key to diversifying domain data is by increasing the types of domain styles. After investigating this issue from the perspective of the Fourier transform, the domain cue is found to be implicitly encoded in the amplitude component of Fourier features, which is more indicative of domain-specific information than statistics (means and standard deviations).
- Categories:
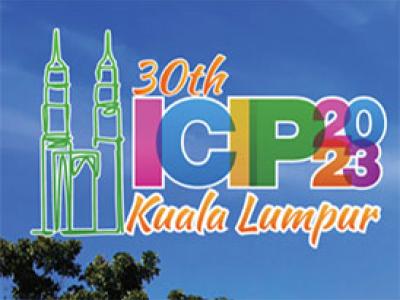
- Read more about SCENE TEXT RECOGNITION MODELS EXPLAINABILITY USING LOCAL FEATURES
- Log in to post comments
Explainable AI (XAI) is the study on how humans can be able to understand the cause of a model’s prediction. In this work, the problem of interest is Scene Text Recognition (STR) Explainability, using XAI to understand the cause of an STR model’s prediction. Recent XAI literatures on STR only provide a simple analysis and do not fully explore other XAI
- Categories:
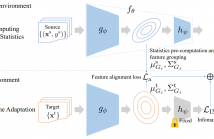
- Read more about COVARIANCE-AWARE FEATURE ALIGNMENT WITH PRE-COMPUTED SOURCE STATISTICS FOR TEST-TIME ADAPTATION TO MULTIPLE IMAGE CORRUPTIONS
- Log in to post comments
Real-world image recognition systems often face corrupted input images, which cause distribution shifts and degrade the performance of models. These systems often use a single prediction model in a central server and process images sent from various environments, such as cameras distributed in cities or cars. Such single models face images corrupted in heterogeneous ways in test time. Thus, they require to instantly adapt to the multiple corruptions during testing rather than being re-trained at a high cost.
- Categories:
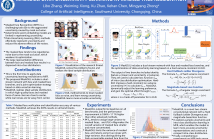
- Read more about MaskDUL: Data Uncertainty Learning in Masked Face Recognition
- Log in to post comments
Since mask occlusion causes plentiful loss of facial feature, Masked Face Recognition (MFR) is a challenging image processing task, and the recognition results are susceptible to noise. However, existing MFR methods are mostly deterministic point embedding models, which are limited in representing noise images. Moreover, Data Uncertainty Learning (DUL) fails to achieve reasonable performance in MFR.
Poster.pdf

- Categories:
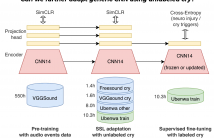
- Read more about Self-supervised learning for infant cry analysis
- Log in to post comments
In this paper, we explore self-supervised learning (SSL) for analyzing a first-of-its-kind database of cry recordings containing clinical indications of more than a thousand newborns. Specifically, we target cry-based detection of neurological injury as well as identification of cry triggers such as pain, hunger, and discomfort.
- Categories:
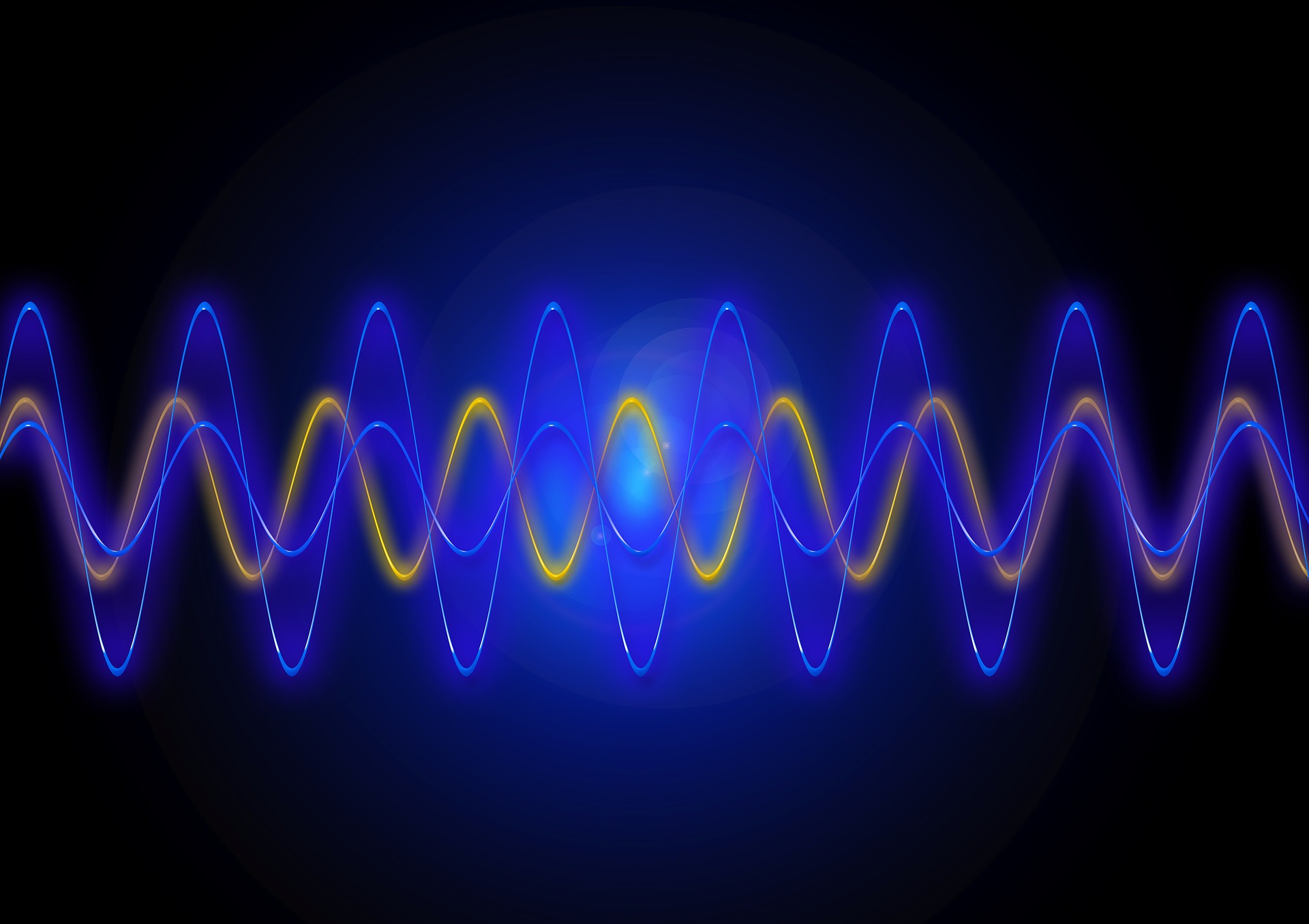
- Read more about Cross-site Generalization for imbalanced epileptic classification
- Log in to post comments
Recently, many studies have been conducted on automated epileptic seizures detection. However, few of these techniques are applied in clinical settings for several reasons. One of them is the imbalanced nature of the seizure detection task. Additionally, the current detection techniques do not really generalize to other patient populations. To address these issues, we present in this paper a hybrid CNN-LSTM model robust to cross-site variability. We investigate the use of data augmentation (DA) methods as an efficient tool to solve imbalanced training problems.
- Categories:
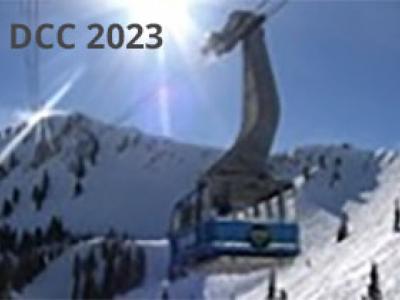
- Read more about Rate-Distortion-Classification Model In Lossy Image Compression
- 1 comment
- Log in to post comments
Rate-distortion (RD) theory is a fundamental theory for lossy image compression that treats compressing the original images to a specified bitrate with minimal signal distortion, which is an essential metric in practical application. Moreover, with the development of visual analysis applications (such as classification, detection, segmentation, etc.), the semantic distortion in compressed images are also an important dimension in the theoretical analysis of lossy image compression.
- Categories:
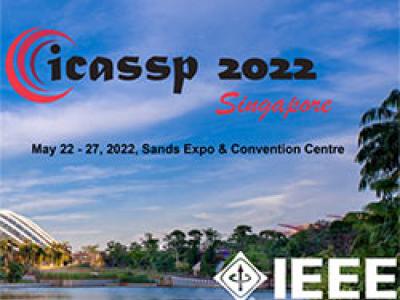
Feature selection has been explored in two ways, global feature selection and instance-wise feature selection. Global feature selection picks the same feature selector for the entire dataset, while instance-wise feature selection allows different feature selectors for different data instances. We propose group-wise feature selection, a new setting that sits between global and instance-wise feature selections.
- Categories:
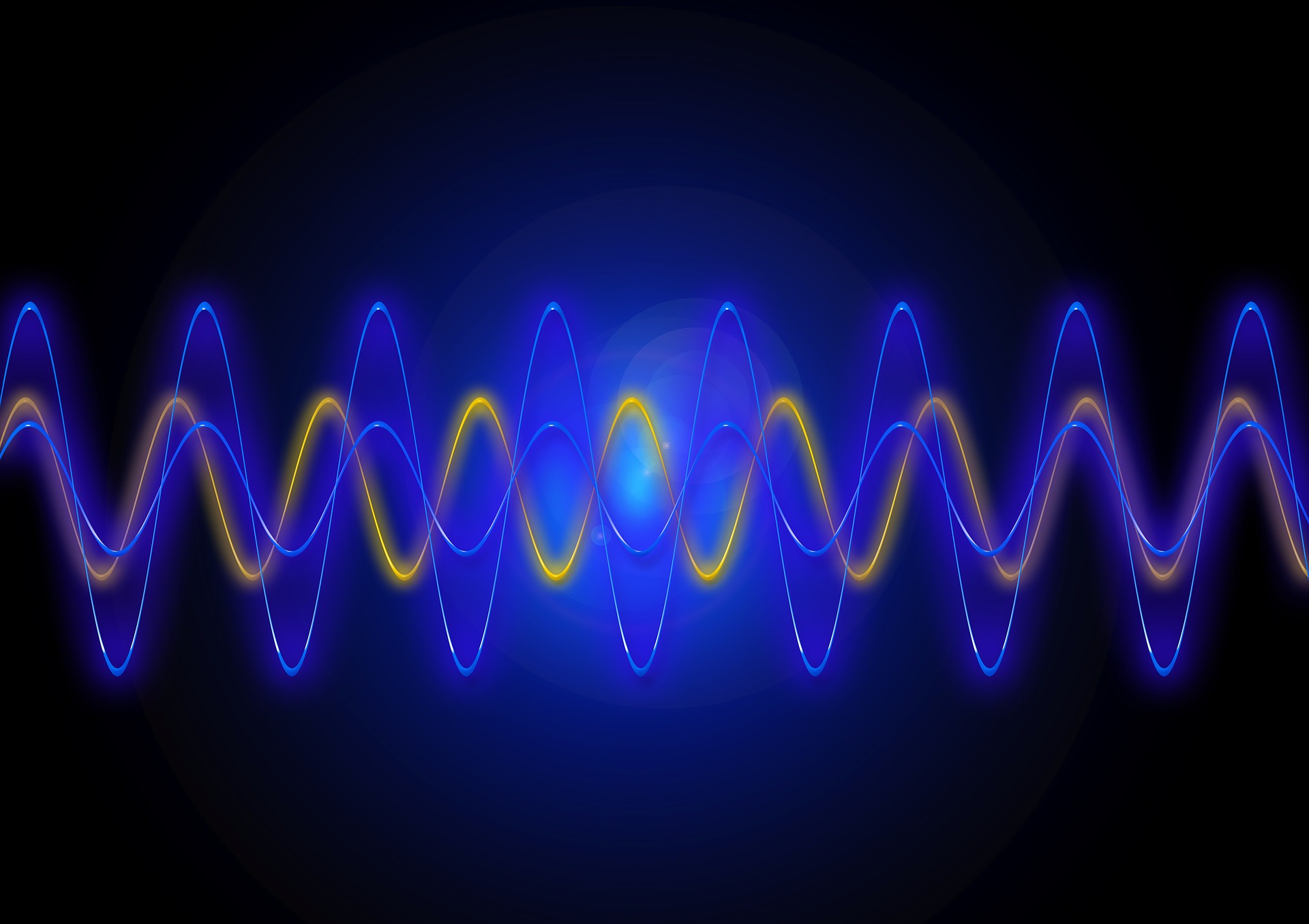
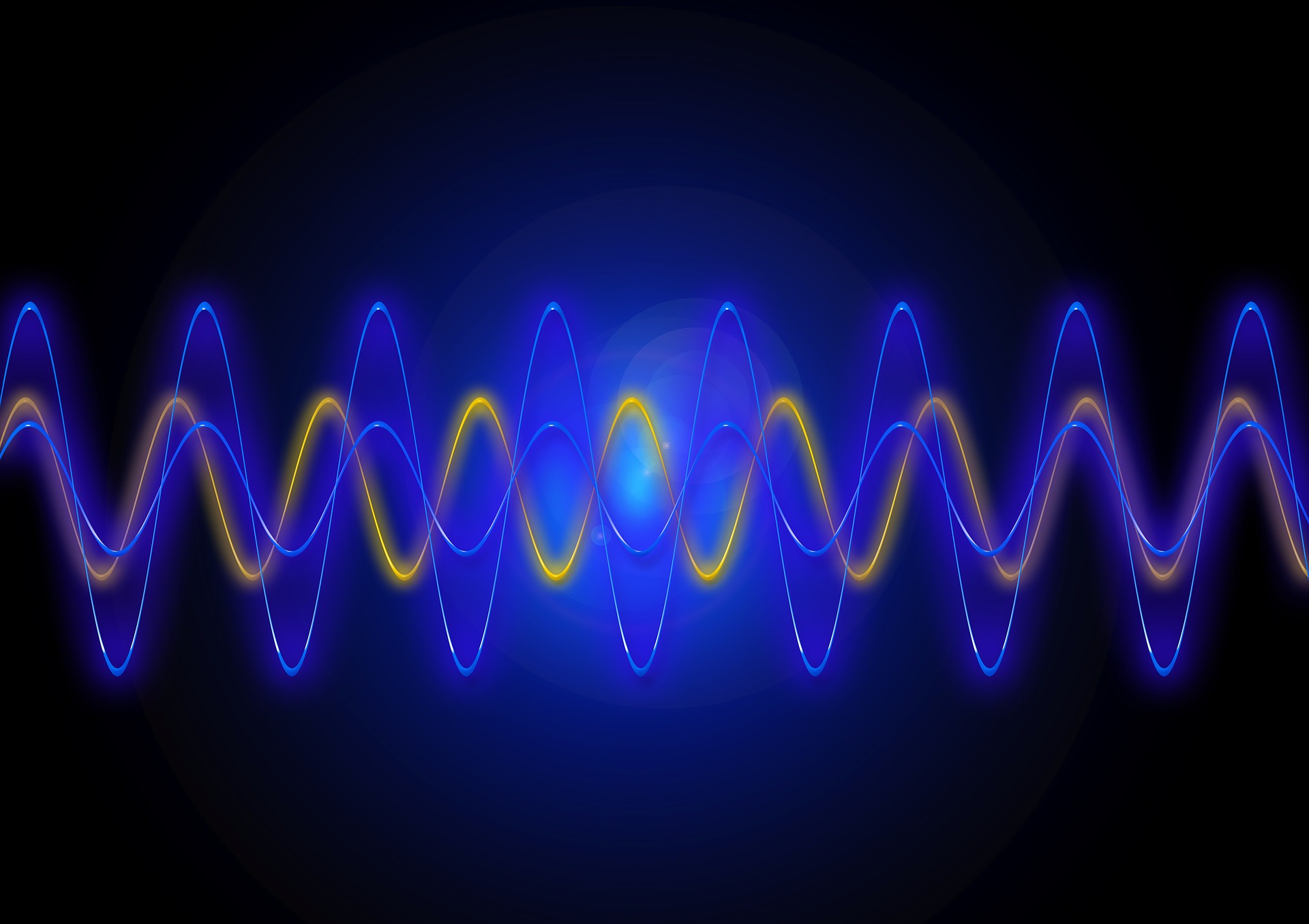