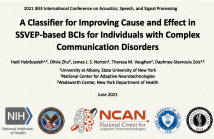
- Read more about A classifier for improving cause and effect in SSVEP-based BCIs for individuals with complex communication disorders
- Log in to post comments
We present CCACUSUM, a classifier for steady-state visual evoked potential (SSVEP)-based brain-computer interfaces (BCIs) that determines whether a user is attending to a flickering stimulus or is at rest. Correct classification of these two states establishes cause and effect between the BCI and its user, which is essential for helping individuals with complex communication disorders (CCDs) communicate.
- Categories:
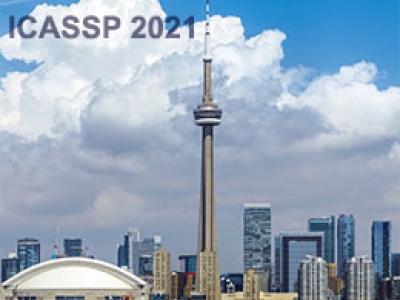
- Read more about SAGA: SPARSE ADVERSARIAL ATTACK ON EEG-BASED BRAIN COMPUTER INTERFACE
- Log in to post comments
With the recent advancement of the Brain-Computer Interface (BCI), Electroencephalogram (EEG) analytics gain a lot of research attention from various domains. Understanding the vulnerabilities of EEG analytics is important for safely applying this emerging technology in our daily life. Recent studies show that EEG analytics are vulnerable to adversarial attacks when adding small perturbations on the EEG data. However, fewer research efforts have been devoted to the robustness of EEG analytics under sparse perturbations that attack only small portions of the data.
- Categories:
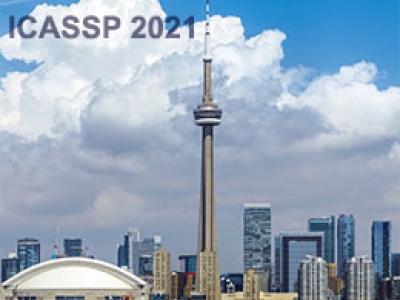
- Read more about A SPARSE CODING APPROACH TO AUTOMATIC DIET MONITORING WITH CONTINUOUS GLUCOSE MONITORS
- Log in to post comments
- Categories:
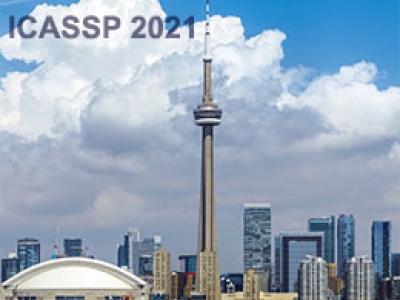
- Read more about TOWARDS THE DEVELOPMENT OF SUBJECT-INDEPENDENT INVERSE METABOLIC MODELS
- Log in to post comments
Diet monitoring is an important component of interventions
in type 2 diabetes, but is time-intensive and often inaccurate. To address this issue, we describe an approach to monitor diet automatically, by analyzing fluctuations in glucose
after a meal is consumed. In particular, we evaluate three
standardization techniques (baseline correction, feature normalization, and model personalization) that can be used to
compensate for the large individual differences that exist in
food metabolism. Then, we build machine learning models
- Categories:
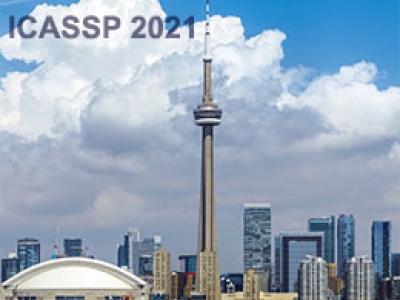
- Read more about Riemannian Geometry-Based Decoding of the Directional Focus of Auditory Attention Using EEG
- Log in to post comments
- Categories:
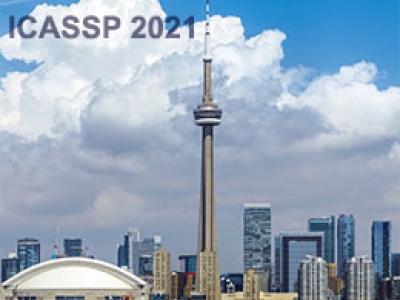
- Read more about Riemannian Geometry-Based Decoding of the Directional Focus of Auditory Attention Using EEG
- Log in to post comments
- Categories:
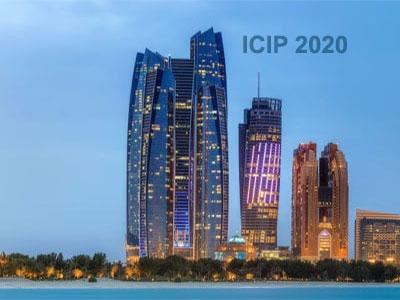
- Read more about RTip : A Fully Automated Root Tip Tracker for Measuring Plant Growth With Intermittent Perturbations
- Log in to post comments
RTip is a tool to quantify plant root growth velocity using high-resolution microscopy image sequences at sub-pixel accuracy. The fully automated RTip tracker is designed for high-throughput analysis of plant phenotyping experiments with episodic perturbations. RTip is able to auto-skip past these manual intervention perturbation activity, i.e. when the root tip is not under the microscope, the image is distorted or blurred. RTip provides the most accurate root growth velocity results with the lowest variance (i.e.
- Categories:
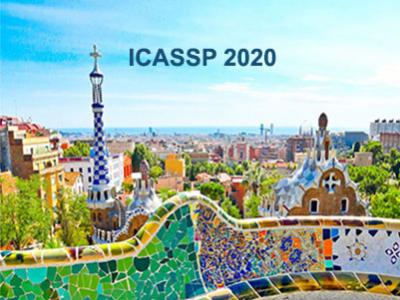
- Read more about HIGH-ACCURACY CLASSIFICATION OF ATTENTION DEFICIT HYPERACTIVITY DISORDER WITH L2,1-NORM LINEAR DISCRIMINANT ANALYSIS
- Log in to post comments
- Categories:
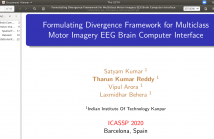
- Read more about FORMULATING DIVERGENCE FRAMEWORK FOR MULTICLASS MOTOR IMAGERY EEG BRAIN COMPUTER INTERFACE
- Log in to post comments
The ubiquitous presence of non-stationarities in the EEG signals significantly perturb the feature distribution thus deteriorating the performance of Brain Computer Interface. In this work, a novel method is proposed based on Joint Approximate Diagonalization (JAD) to optimize stationarity for multiclass motor imagery Brain Computer Interface (BCI) in an information theoretic framework. Specifically, in the proposed method, we estimate the subspace which optimizes the discriminability between the classes and simultaneously preserve stationarity within the motor imagery classes.
- Categories:
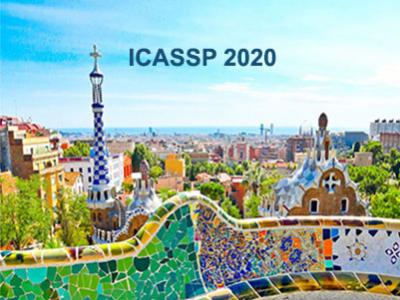
- Read more about An LSTM Based Architecture to Relate Speech Stimulus to EEG
- 1 comment
- Log in to post comments
icassp2020.pdf

- Categories: