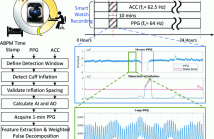
- Read more about MAML-Based 24-Hour Personalized Blood Pressure Estimation from Wrist Photoplethysmography Signals in Free-Living Context
- Log in to post comments
Systolic blood pressure (BP) variability at daytime and nighttime, also known as BP dip, has shown its clinical value in diagnosis and treatment of cardiovascular diseases (CVDs). A model agnostic meta learning (MAML)-based 24-hour personalized approach is proposed in this paper for BP estimation using wrist photoplethysmography (PPG) signals from smart watch in free-living context.
- Categories:
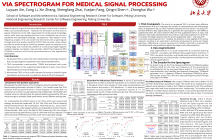
- Read more about TRLS: A TIME SERIES REPRESENTATION LEARNING FRAMEWORK VIA SPECTROGRAM FOR MEDICAL SIGNAL PROCESSING
- Log in to post comments
Representation learning frameworks in unlabeled time series have been proposed for medical signal processing. Despite the numerous excellent progresses have been made in previous works, we observe the representation extracted for the time series still does not generalize well. In this paper, we present a Time series (medical signal) Representation Learning framework via Spectrogram (TRLS) to get more informative representations. We transform the input time-
- Categories:
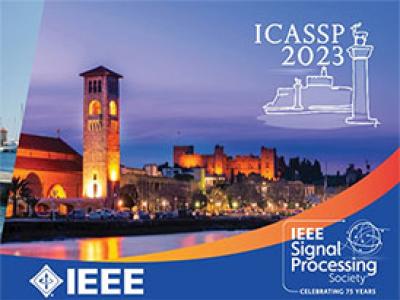
- Read more about ICASSP 2023 AUDITORY EEG DECODING CHALLENGE
- Log in to post comments
- Categories:
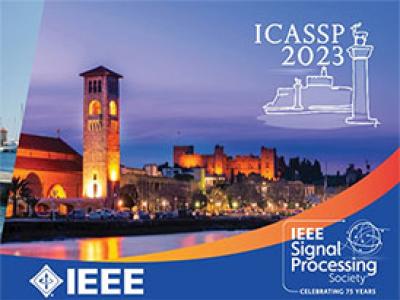
- Read more about Spatial Graph Signal Interpolation with an Application for Merging BCI Datasets with Various Dimensionalities
- Log in to post comments
BCI Motor Imagery datasets usually are small and have different electrodes setups. When training a Deep Neural Network, one may want to capitalize on all these datasets to increase the amount of data available and hence obtain good generalization results.
- Categories:
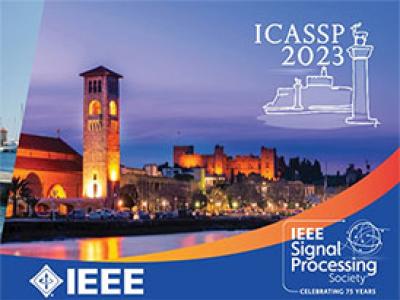
- Read more about FEDEEG__FEDERATED_EEG_DECODING_VIA_INTER_SUBJECT_STRUCTURE_MATCHING
- Log in to post comments
With sufficient centralized training data coming from multiple subjects, deep learning methods have achieved powerful EEG decoding performance. However, sending each individuals’ EEG data directly to a centralized server might cause privacy leakage. To overcome this issue, we present an inter-subject structure matching-based federated EEG decoding (FedEEG) framework. First, we introduce a center loss to each client (subject), which can learn multiple virtual class centers by averaging the corresponding class-specific EEG features.
- Categories:
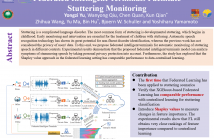
- Read more about Federated Intelligent Terminals Facilitate Stuttering Monitoring
- Log in to post comments
Stuttering is a complicated language disorder. The most common form of stuttering is developmental stuttering, which begins in childhood. Early monitoring and intervention are essential for the treatment of children with stuttering. Automatic speech recognition technology has shown its great potential for non-fluent disorder identification, whereas the previous work has not considered the privacy of users' data. To this end, we propose federated intelligent terminals for automatic monitoring of stuttering speech in different contexts.
- Categories:
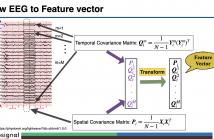
- Read more about Active selection of source patients in transfer learning for epileptic seizure detection using Riemannian Manifold
- Log in to post comments
Epileptic seizure detection from long recordings of scalp electroencephalography (EEG) is a challenging task owing to their unpredictability in nature with the inclusion of noise, artifacts and subject dependency. We hypothesize that selection of training EEG data plays important role in the model performance. Thus, we introduced an active learning based training data selection and modification method with a Riemannian geometry, centroid alignment, tangent space mapping and a support vector machine classifier.
- Categories:
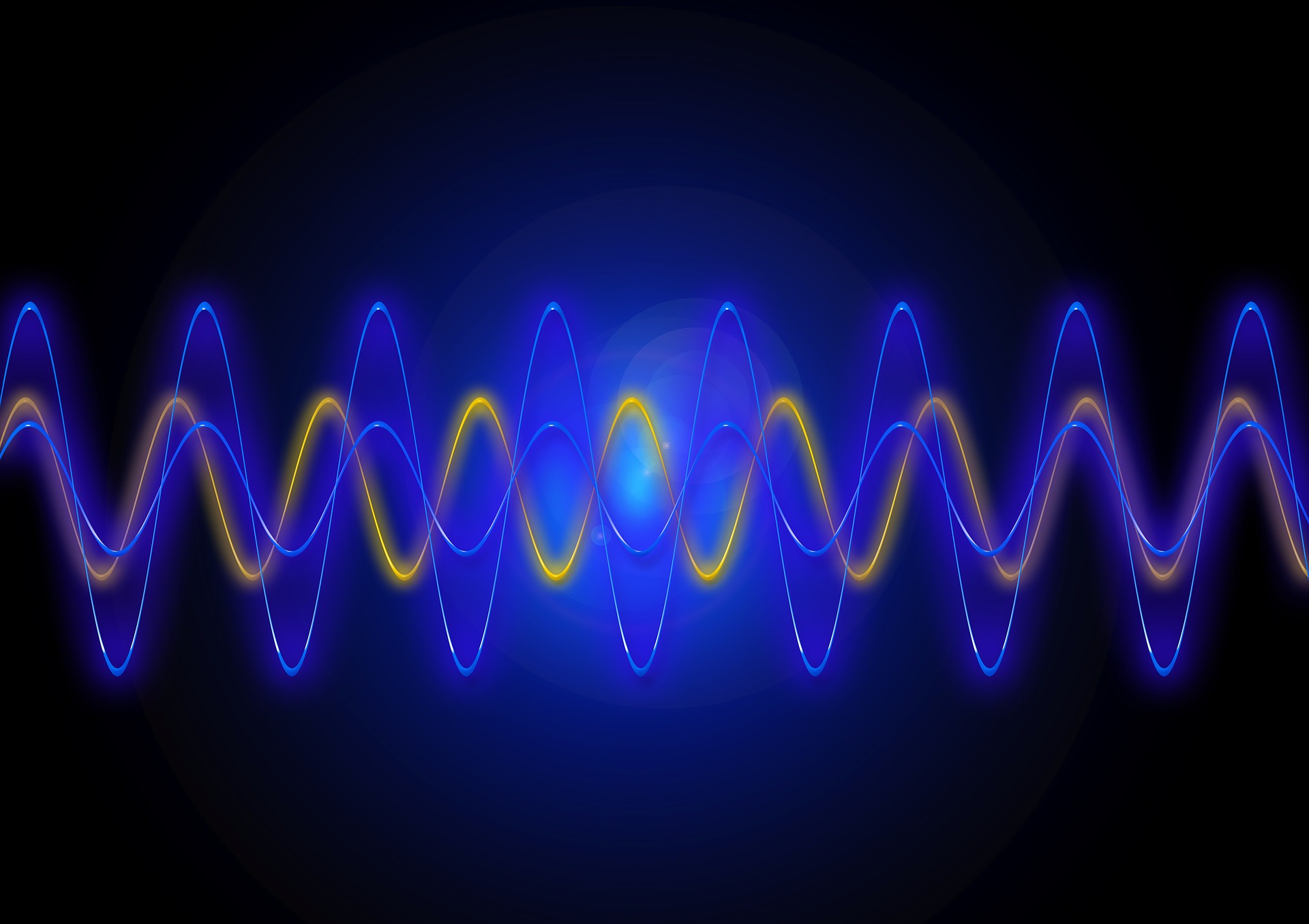
- Read more about Improving EEG-based Emotion Recognition by Fusing Time-frequency And Spatial Representations
- Log in to post comments
Using deep learning methods to classify EEG signals can accurately identify people's emotions. However, existing studies have rarely considered the application of the information in another domain's representations to feature selection in the time-frequency domain. We propose a classification network of EEG signals based on the cross-domain feature fusion method, which makes the network more focused on the features most related to brain activities and thinking changes by using the multi-domain attention mechanism.
- Categories:
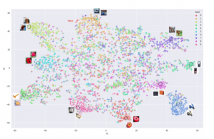
Reconstructing images using brain signals of imagined visuals may provide an augmented vision to the disabled, leading to the advancement of Brain-Computer Interface (BCI) technology. The recent progress in deep learning has boosted the study area of synthesizing images from brain signals using Generative Adversarial Networks (GAN). In this work, we have proposed a framework for synthesizing the images from the brain activity recorded by an electroencephalogram (EEG) using small-size EEG datasets.
- Categories:
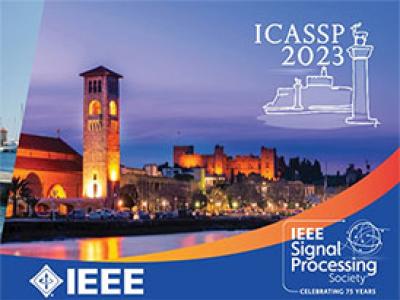
- Read more about Ensemble and Personalized Transformer Models for Subject Identification and Relapse Detection in E-Prevention Challenge
- Log in to post comments
In this short paper, we present the devised solutions for the subject identification and relapse detection tasks, which are part of the e-Prevention Challenge hosted at the ICASSP 2023 conference [1] [2] [3]. We specifically design an ensemble scheme of six models - five transformer-based ones and a CNN model - for the identification of subjects from wearable devices, while a personalized - one for each subject - scheme is used for relapse detection in psychotic disorder.
- Categories: