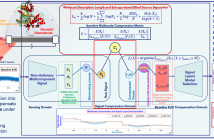
- Read more about Unsupervised Drift Compensation Based on Information Theory for Single-Molecule Sensors
- Log in to post comments
Single-molecule sensors based on carbon nanotubes transducer, enable to probe stochastic molecular dynamics thanks to long acquisition periods and high throughput measurements. With such sampling conditions, the sensor baseline may drift significantly and induce fake states and transitions in the recorded signal, leading to wrong kinetic estimates from the inferred state model.
We present MDL-AdaCHIP a multiscale signal compression technique based on the Minimum Description Length (MDL) principle, combined with an Adaptive piecewise Cubic Hermite Interpolation (AdaCHIP), both implemented into a blind source separation framework to compensate the parasitic baseline drift in single-molecule biosensors
- Categories:
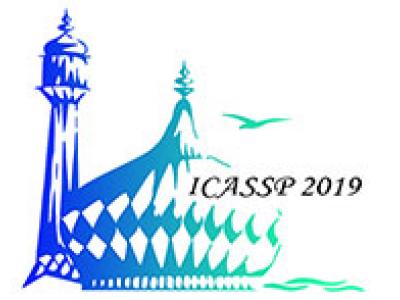
Increasingly, post-secondary instructors are incorporating innovative teaching practices into their classrooms to improve student learning outcomes. In order to assess the effect of these techniques, it is helpful to quantify the types of activity being conducted in the classroom. Unfortunately, self-reporting is unreliable and manual annotation is tedious and scales poorly.
- Categories:
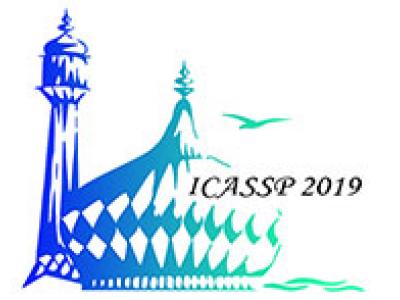
- Read more about Feature Selection for Multi-labeled Variables via Dependency Maximization
- Log in to post comments
Feature selection and reducing the dimensionality of data is an essential step in data analysis. In this work, we propose a new criterion for feature selection that is formulated as conditional information between features given the labeled variable. Instead of using the standard mutual information measure based on Kullback-Leibler divergence, we use our proposed criterion to filter out redundant features for the purpose of multiclass classification.
- Categories:
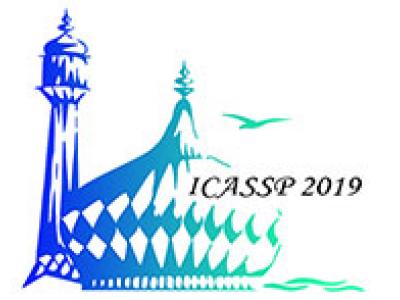
- Read more about ICASSP 2019 Poster for Paper #3198: PRIVACY-AWARE FEATURE EXTRACTION FOR GENDER DISCRIMINATION VERSUS SPEAKER IDENTIFICATION
- Log in to post comments
This paper introduces a deep neural network based feature extraction scheme that aims to improve the trade-off between utility and privacy in speaker classification tasks. In the proposed scenario we develop a feature representation that helps to maximize the performance of a gender classifier while minimizing additional speaker
- Categories:
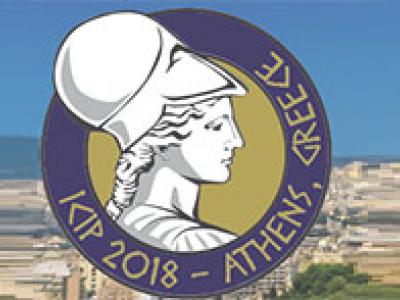
- Read more about CONDITIONAL DISTRIBUTION LEARNING WITH NEURAL NETWORKS AND ITS APPLICATION TO UNIVERSAL IMAGE DENOISING
- Log in to post comments
A simple and scalable denoising algorithm is proposed that can be applied to a wide range of source and noise models. At the core of the proposed CUDE algorithm is symbol-by-symbol universal denoising used by the celebrated DUDE algorithm, whereby the optimal estimate of the source from an unknown distribution is computed by inverting the empirical distribution of the noisy observation sequence by a deep neural network, which naturally and implicitly aggregates multiple contexts of similar characteristics and estimates the conditional distribution more accurately.
- Categories:
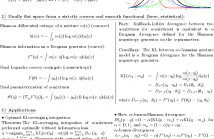
- Categories:
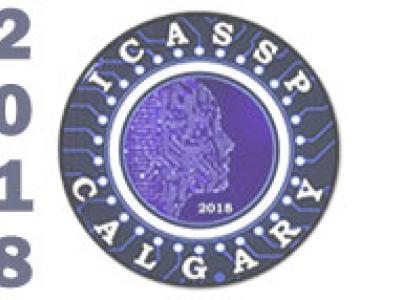
- Read more about RATE-OPTIMAL META LEARNING OF CLASSIFICATION ERROR
- Log in to post comments
Meta learning of optimal classifier error rates allows an experimenter to empirically estimate the intrinsic ability of any estimator to discriminate between two populations, circumventing the difficult problem of estimating the optimal Bayes classifier. To this end we propose a weighted nearest neighbor (WNN) graph estimator for a tight bound on the Bayes classification error; the Henze-Penrose (HP) divergence. Similar to recently proposed HP estimators [berisha2016], the proposed estimator is non-parametric and does not require density estimation.
- Categories:
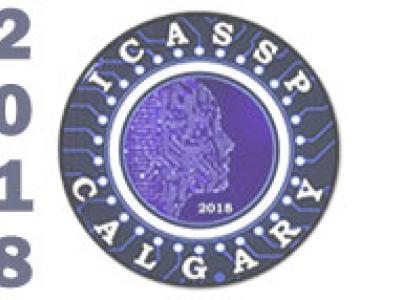
- Read more about Rate-optimal Meta Learning of Classification Error
- Log in to post comments
Meta learning of optimal classifier error rates allows an experimenter to empirically estimate the intrinsic ability of any estimator to discriminate between two populations, circumventing the difficult problem of estimating the optimal Bayes classifier. To this end we propose a weighted nearest neighbor (WNN) graph estimator for a tight bound on the Bayes classification error; the Henze-Penrose (HP) divergence. Similar to recently proposed HP estimators [berisha2016], the proposed estimator is non-parametric and does not require density estimation.
- Categories:
- Read more about A multi-layer image representation using Regularized Residual Quantization: application to compression and denoising
- Log in to post comments
A learning-based framework for representation of domain-specific images is proposed where joint compression and denoising can be done using a VQ-based multi-layer network. While it learns to compress the images from a training set, the compression performance is very well generalized on images from a test set. Moreover, when fed with noisy versions of the test set, since it has priors from clean images, the network also efficiently denoises the test images during the reconstruction.
- Categories: