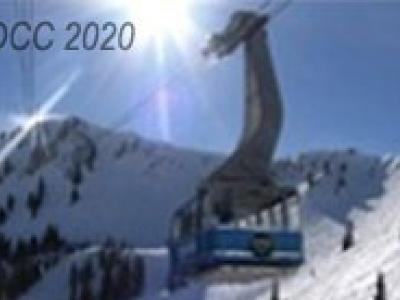
- Read more about Approximating Optimal Bidirectional Macro Schemes.
- 1 comment
- Log in to post comments
Lempel-Ziv is an easy-to-compute member of a wide family of so-called macro schemes; it restricts pointers to go in one direction only. Optimal bidirectional macro schemes are NP-complete to find, but they may provide much better compression on highly repetitive sequences. We consider the problem of approximating optimal bidirectional macro schemes. We describe a simulated annealing algorithm that usually converges quickly. Moreover, in some cases, we obtain bidirectional macro schemes that are provably a 2-approximation of the optimal.
- Categories:
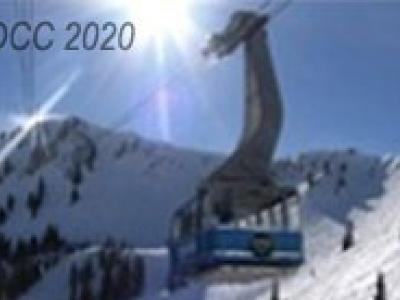
- Read more about Grammar compression with probabilistic context-free grammar
- 2 comments
- Log in to post comments
We propose a new approach for universal lossless text compression, based on grammar compression. In the literature, a target string T has been compressed as a context-free grammar G in Chomsky normal form satisfying L(G) = T. Such a grammar is often called a straight-line program (SLP). In this work, we consider a probabilistic grammar G that generates T, but not necessarily as a unique element of L(G). In order to recover the original text T unambiguously, we keep both the grammar G and the derivation tree of T from the start symbol in G, in compressed form.
dcc_poster.pdf

- Categories:
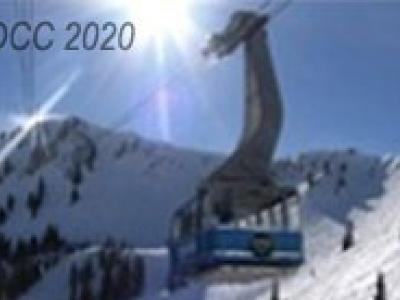
- Read more about Reverse Multi-Delimiter Compression Codes
- Log in to post comments
An enhanced version of a recently introduced family of variable length binary codes with multiple pattern delimiters is presented and discussed. These codes are complete, universal, synchronizable, they have monotonic indexing and allow a standard search in compressed files. Comparing the compression rate on natural language texts demonstrates that introduced codes appear to be much superior to other known codes with similar properties. A fast byte-aligned decoding algorithm is constructed, which operates much faster than the one for Fibonacci codes.
- Categories:
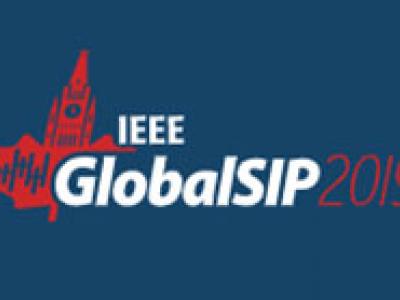
- Read more about An Accurate Evaluation of MSD Log-likelihood and its Application in Human Action Recognition
- Log in to post comments
In this paper, we examine the problem of modeling overdispersed frequency vectors that are naturally generated by several machine learning and computer vision applications.
- Categories:
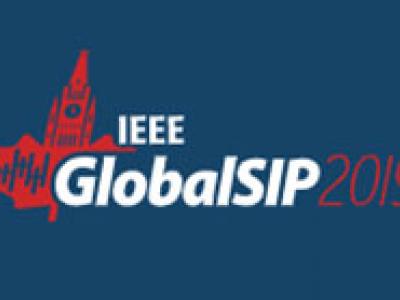
- Read more about An Accurate Evaluation of MSD Log-likelihood and its Application in Human Action Recognition
- Log in to post comments
In this paper, we examine the problem of modeling overdispersed frequency vectors that are naturally generated by several machine learning and computer vision applications.
MSD_Mesh.pdf

- Categories:
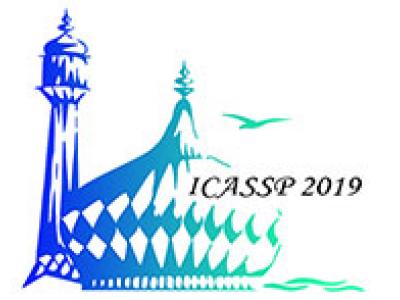
In this research, we aim to propose a data preprocessing framework particularly for financial sector to generate the rating data as input to the collaborative system. First, clustering technique is applied to cluster all users based on their demographic information which might be able to differentiate the customers’ background. Then, for each customer group, the importance of demographic characteristics which are highly associated with financial products purchasing are analyzed by the proposed fuzzy integral technique.
- Categories:
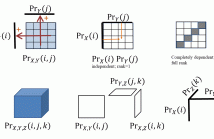
- Read more about GlobalSIP 2018 Keynote: Tensors and Probability: An Intriguing Union (N. Sidiropoulos, N. Kargas, X. Fu)
- Log in to post comments
We reveal an interesting link between tensors and multivariate statistics. The rank of a multivariate probability tensor can be interpreted as a nonlinear measure of statistical dependence of the associated random variables. Rank equals one when the random variables are independent, and complete statistical dependence corresponds to full rank; but we show that rank as low as two can already model strong statistical dependence.
- Categories:
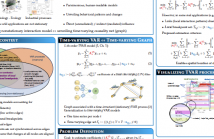
- Read more about Dynamic Network Identification From Non-stationary Vector Autoregressive Time Series
- Log in to post comments
Learning the dynamics of complex systems features a large number of applications in data science. Graph-based modeling and inference underpins the most prominent family of approaches to learn complex dynamics due to their ability to capture the intrinsic sparsity of direct interactions in such systems. They also provide the user with interpretable graphs that unveil behavioral patterns and changes.
- Categories:
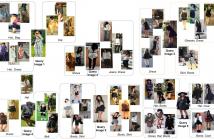
Developing recommendation system for fashion images is challenging due to the inherent ambiguity associated with what criterion a user is looking at. Suggesting multiple images where each output image is similar to the query image on the basis of a different feature or part is one way to mitigate the problem. Existing works for fashion recommendation have used Siamese or Triplet network to learn features between a similar pair and a similar dissimilar triplet respectively.
- Categories:
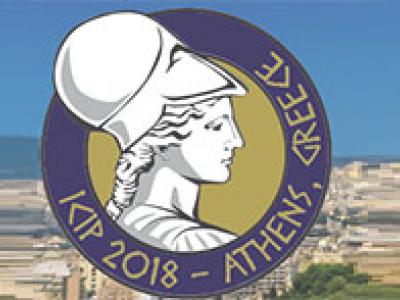
Fly Local Sensitive Hashing (FLSH) is a biomimetic data-independent hashing method inspired by the mechanism of odor processing system in drosophila. In this paper,we propose a novel Randomized Sampling-based Fly Local Sensitive Hashing (rs-FLSH) to model the randomness occurred during the establishment of synapses between neurons.Significant performance improvement can be achieved by applying a novel randomized sampling scheme in rs-FLSH,in which the sample rate is modeled by a Gaussian random variable rather than a fixed value in FLSH.
- Categories: