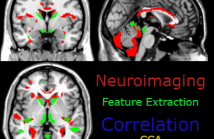
- Read more about MULTIMODAL IMAGING FEATURE EXTRACTION WITH REFERENCE CANONICAL CORRELATION ANALYSIS UNDERLYING INTELLIGENCE
- Log in to post comments
With neuroimaging data scientists have gained substantial information on the neuronal underpinning of intelligence. Yet how to integrate multimodal neuronal features effectively in relation to intelligence remains elusive. In this paper, we have developed a reference Canonical Correlation Analysis (RCCA) model that extracts latent, correlated multimodal features while enhancing correlation to a reference of interest.
- Categories:
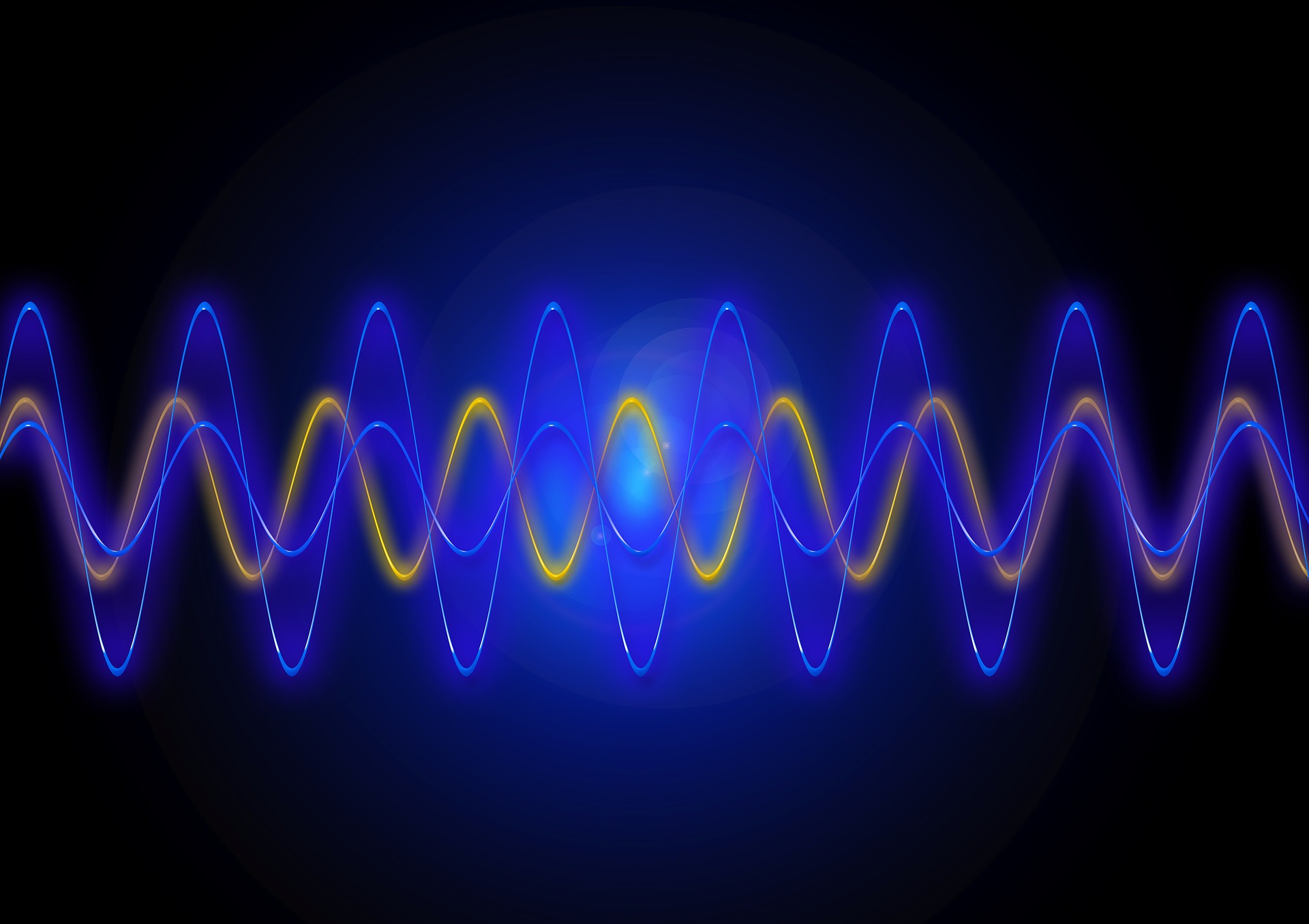
- Read more about RESIDUAL DENSE SWIN TRANSFORMER FOR CONTINUOUS DEPTH-INDEPENDENT ULTRASOUND IMAGING
- Log in to post comments
Ultrasound imaging is crucial for evaluating organ morphology and function, yet depth adjustment can degrade image quality and field-of-view, presenting a depth-dependent dilemma. Traditional interpolation-based zoom-in techniques often sacrifice detail and introduce artifacts. Motivated by the potential of arbitrary-scale super-resolution to naturally address these inherent challenges, we present the Residual Dense Swin Transformer Network (RDSTN), designed to capture the non-local characteristics and long-range dependencies intrinsic to ultrasound images.
- Categories:
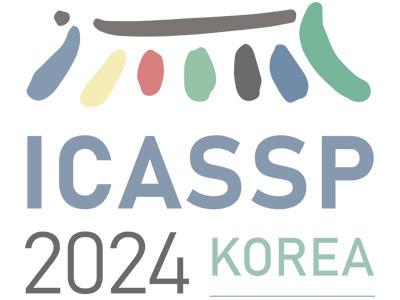
- Read more about EARLY DIAGNOSING PARKINSON'S DISEASE VIA A DEEP LEARNING MODEL BASED ON AUGMENTED FACIAL EXPRESSION DATA
- Log in to post comments
It is crucial to promptly diagnose potential Parkinson's disease (PD) patients in order to facilitate early treatment and prevent disease progression. In recent years, there has been growing interest in using facial expressions for in-vitro PD diagnosis due to the distinct "masked face" characteristics of PD patients and the cost-effectiveness of this approach. However, current facial expression-based PD diagnosis methods are hindered by limited training data on PD patients' facial expressions and weak prediction models.
- Categories:
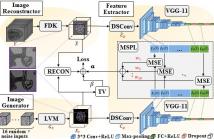
- Read more about STABLE OPTIMIZATION FOR LARGE VISION MODEL BASED DEEP IMAGE PRIOR IN CONE-BEAM CT RECONSTRUCTION
- Log in to post comments
Large Vision Model (LVM) has recently demonstrated great potential for medical imaging tasks, potentially enabling image enhancement for sparse-view Cone-Beam Computed Tomography (CBCT), despite requiring a substantial amount of data for training. Meanwhile, Deep Image Prior (DIP) effectively guides an untrained neural network to generate high-quality CBCT images without any training data. How- ever, the original DIP method relies on a well-defined forward model and a large-capacity backbone network, which is no- toriously difficult to converge.
- Categories:
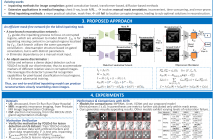
- Read more about BLIND INPAINTING WITH OBJECT-AWARE DISCRIMINATION FOR ARTIFICIAL MARKER REMOVAL
- Log in to post comments
Medical images often incorporate doctor-added markers that can hinder AI-based diagnosis. This issue highlights the need of inpainting techniques to restore the corrupted visual contents. However, existing methods require manual mask annotation as input, limiting the application scenarios. In this paper, we propose a novel blind inpainting method that automatically reconstructs visual contents within the corrupted regions without mask input as guidance. Our model includes a blind reconstruction network and an object-aware discriminator for adversarial training.
- Categories:
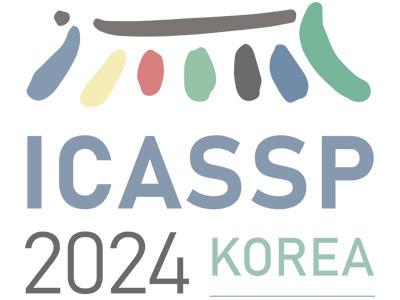
- Read more about Enhancing Generalization in Medical Visual Question Answering Tasks via Gradient-Guided Model Perturbation
- Log in to post comments
Leveraging pre-trained visual language models has become a widely adopted approach for improving performance in downstream visual question answering (VQA) applications. However, in the specialized field of medical VQA, the scarcity of available data poses a significant barrier to achieving reliable model generalization. Numerous methods have been proposed to enhance model generalization, addressing the issue from data-centric and model-centric perspectives.
- Categories:
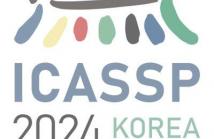
- Read more about Embedded Feature Similarity Optimization with Specific Parameter Initialization for 2D/3D Medical Image Registration
- Log in to post comments
We present a novel deep learning-based framework: Embedded Feature Similarity Optimization with Specific Parameter Initialization (SOPI) for 2D/3D medical image registration which is a most challenging problem due to the difficulty such as dimensional mismatch, heavy computation load and lack of golden evaluation standard. The framework we design includes a parameter specification module to efficiently choose initialization pose parameter and a fine-registration module to align images.
- Categories:
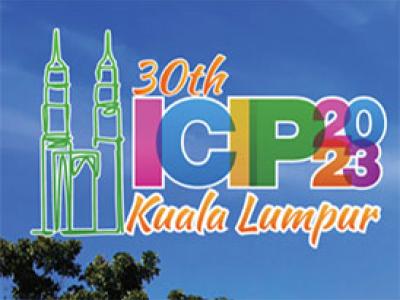
- Read more about AN AUTOMATIC COLORECTAL POLYPS DETECTION APPROACH FOR CT COLONOGRAPHY
- Log in to post comments
- Categories:
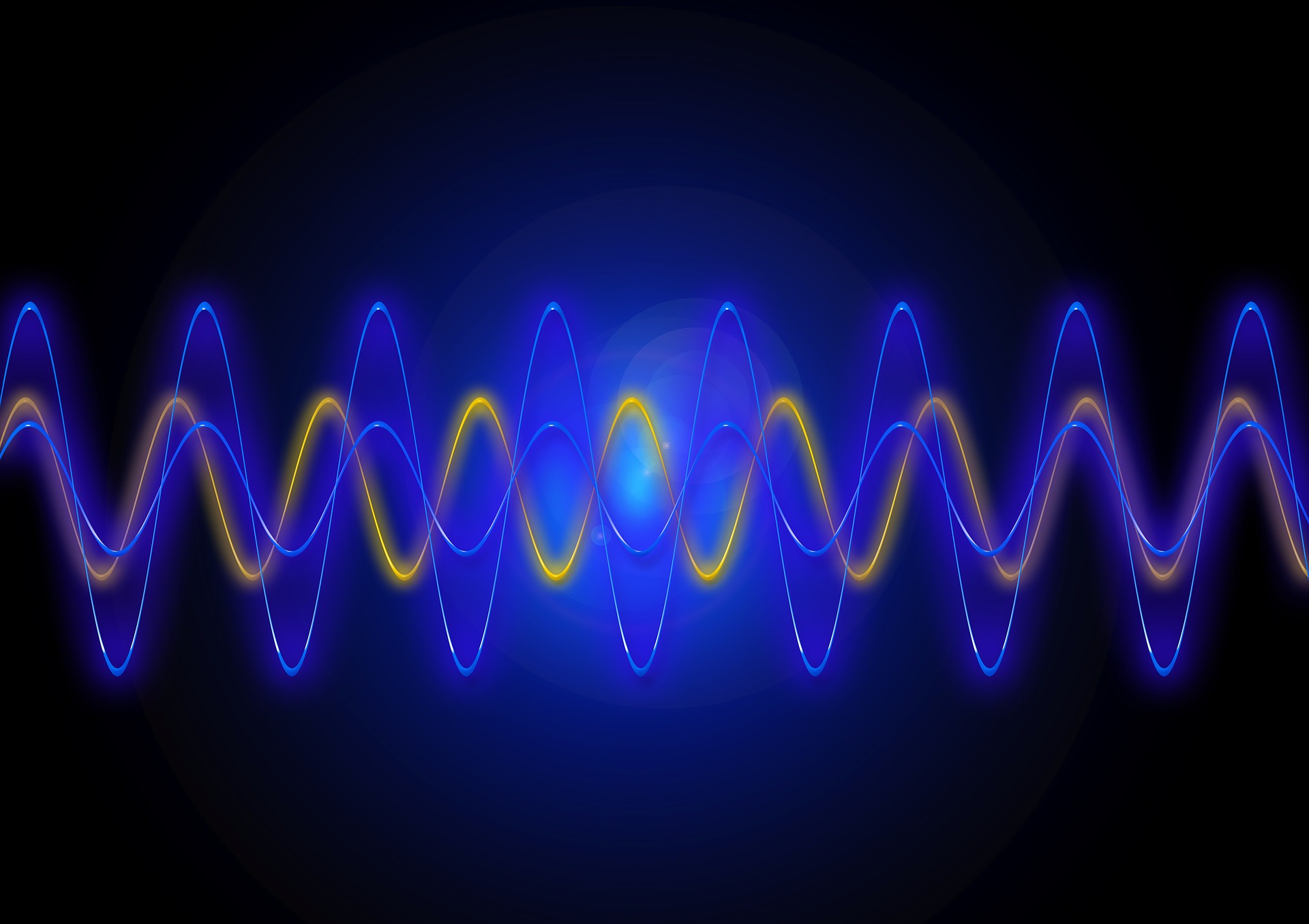
- Read more about SEMI-SUPERVISED CONTRASTIVE LEARNING OF GLOBAL AND LOCAL REPRESENTATION FOR 3D MEDICAL IMAGE SEGMENTATION
- Log in to post comments
- Categories:
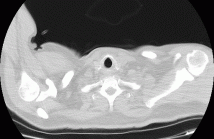
- Read more about Ensemble Methods for Enhanced COVID-19 CT scan severity analysis
- Log in to post comments
Computed Tomography (CT) scans provide a high-resolutionimage of the lungs, allowing clinicians to identify the severity of infections in COVID-19 patients. This paper presents a domain knowledge-based pipeline for extracting infection regions from COVID-19 patients using a combination of image processing algorithms and a pre-trained UNET model. Then, an infection rate-based feature vector is generated for each CT scan.
- Categories: