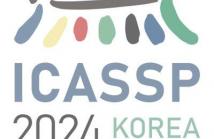
- Read more about Embedded Feature Similarity Optimization with Specific Parameter Initialization for 2D/3D Medical Image Registration
- Log in to post comments
We present a novel deep learning-based framework: Embedded Feature Similarity Optimization with Specific Parameter Initialization (SOPI) for 2D/3D medical image registration which is a most challenging problem due to the difficulty such as dimensional mismatch, heavy computation load and lack of golden evaluation standard. The framework we design includes a parameter specification module to efficiently choose initialization pose parameter and a fine-registration module to align images.
- Categories:
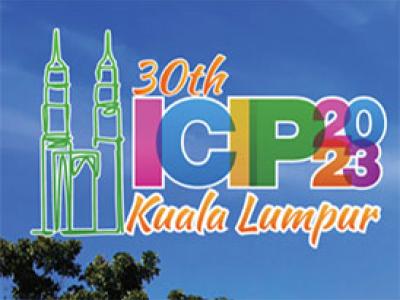
- Read more about AN AUTOMATIC COLORECTAL POLYPS DETECTION APPROACH FOR CT COLONOGRAPHY
- Log in to post comments
- Categories:
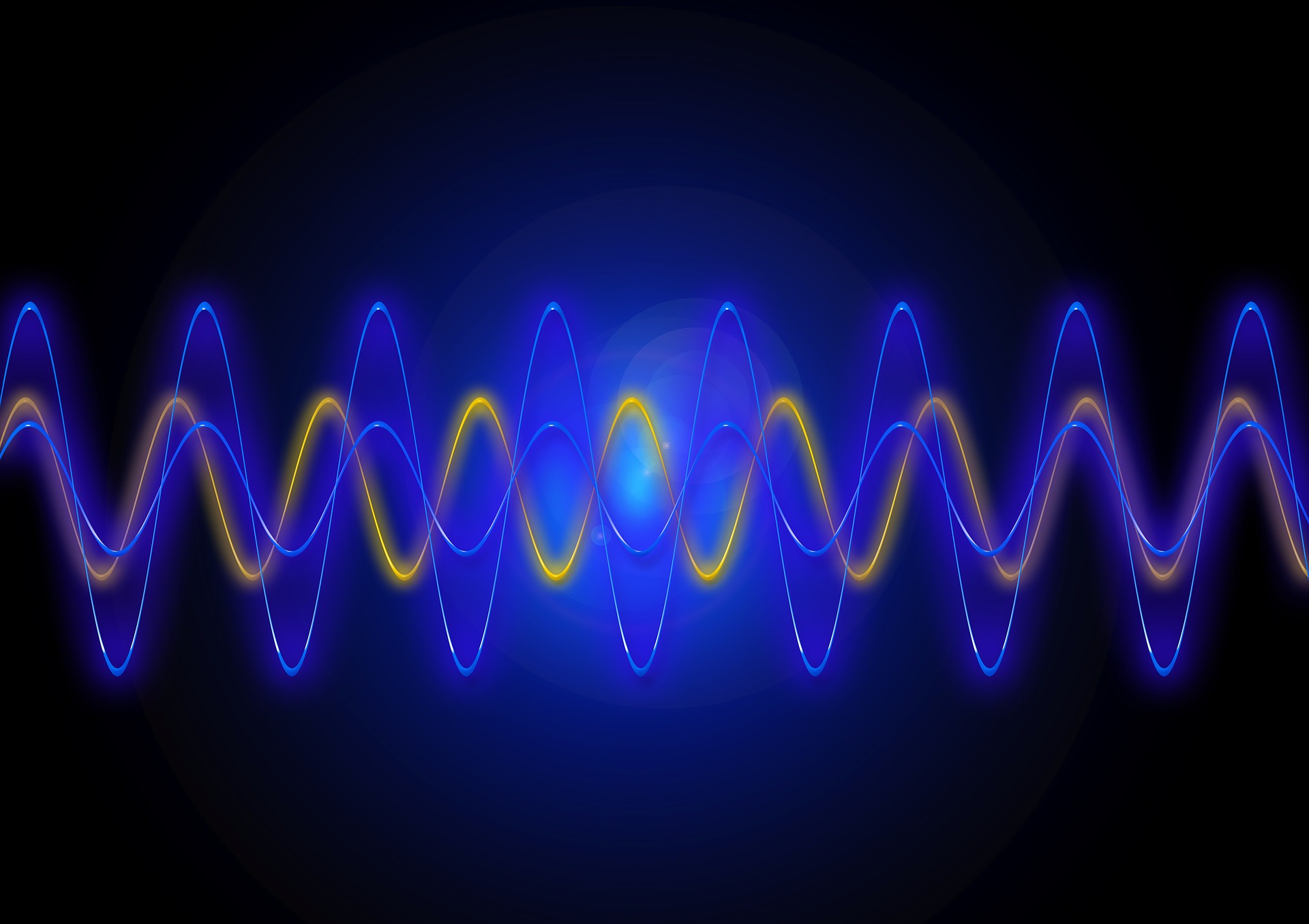
- Read more about SEMI-SUPERVISED CONTRASTIVE LEARNING OF GLOBAL AND LOCAL REPRESENTATION FOR 3D MEDICAL IMAGE SEGMENTATION
- Log in to post comments
- Categories:
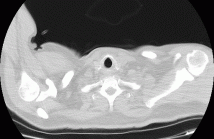
- Read more about Ensemble Methods for Enhanced COVID-19 CT scan severity analysis
- Log in to post comments
Computed Tomography (CT) scans provide a high-resolutionimage of the lungs, allowing clinicians to identify the severity of infections in COVID-19 patients. This paper presents a domain knowledge-based pipeline for extracting infection regions from COVID-19 patients using a combination of image processing algorithms and a pre-trained UNET model. Then, an infection rate-based feature vector is generated for each CT scan.
- Categories:
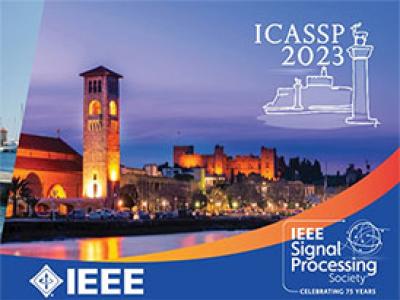
- Read more about IDEAL: Improved DEnse LocAL Contrastive Learning for Semi-Supervised Medical Image Segmentation
- Log in to post comments
Due to the scarcity of labeled data, Contrastive Self-Supervised Learning (SSL) frameworks have lately shown great potential in several medical image analysis tasks. However, the existing contrastive mechanisms are sub-optimal for dense pixel-level segmentation tasks due to their inability to mine local features. To this end, we extend the concept of metric learning to the segmentation task, using a dense (dis)similarity learning for pre-training a deep encoder network, and employing a semi-supervised paradigm to fine-tune for the downstream task.
- Categories:
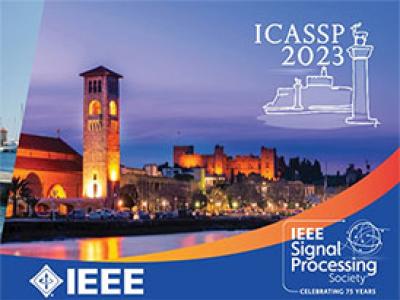
- Read more about IDEAL: Improved DEnse LocAL Contrastive Learning for Semi-Supervised Medical Image Segmentation
- Log in to post comments
Due to the scarcity of labeled data, Contrastive Self-Supervised Learning (SSL) frameworks have lately shown great potential in several medical image analysis tasks. However, the existing contrastive mechanisms are sub-optimal for dense pixel-level segmentation tasks due to their inability to mine local features. To this end, we extend the concept of metric learning to the segmentation task, using a dense (dis)similarity learning for pre-training a deep encoder network, and employing a semi-supervised paradigm to fine-tune for the downstream task.
- Categories:
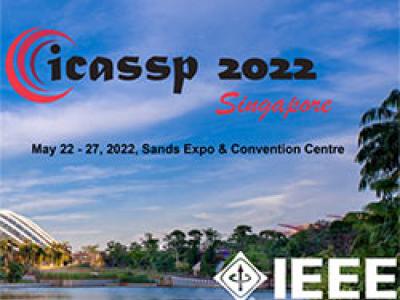
- Read more about SELF-KNOWLEDGE DISTILLATION BASED SELF-SUPERVISED LEARNING FOR COVID-19 DETECTION FROM CHEST X-RAY IMAGES
- Log in to post comments
1206-3.pdf

- Categories:
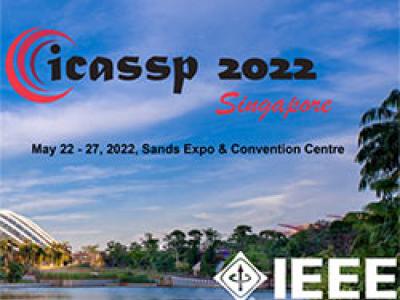
- Read more about Multiview Long-Short Spatial Contrastive Learning for 3D Medical Image Analysis
- Log in to post comments
The success of supervised deep learning heavily depends on large labeled datasets whose construction is often challenging in medical image analysis. Contrastive learning, a variant of self-supervised learning, is a potential solution to alleviate the strong demand for data annotation. In this work, we extend the contrastive learning framework to 3D volumetric medical imaging.
- Categories:
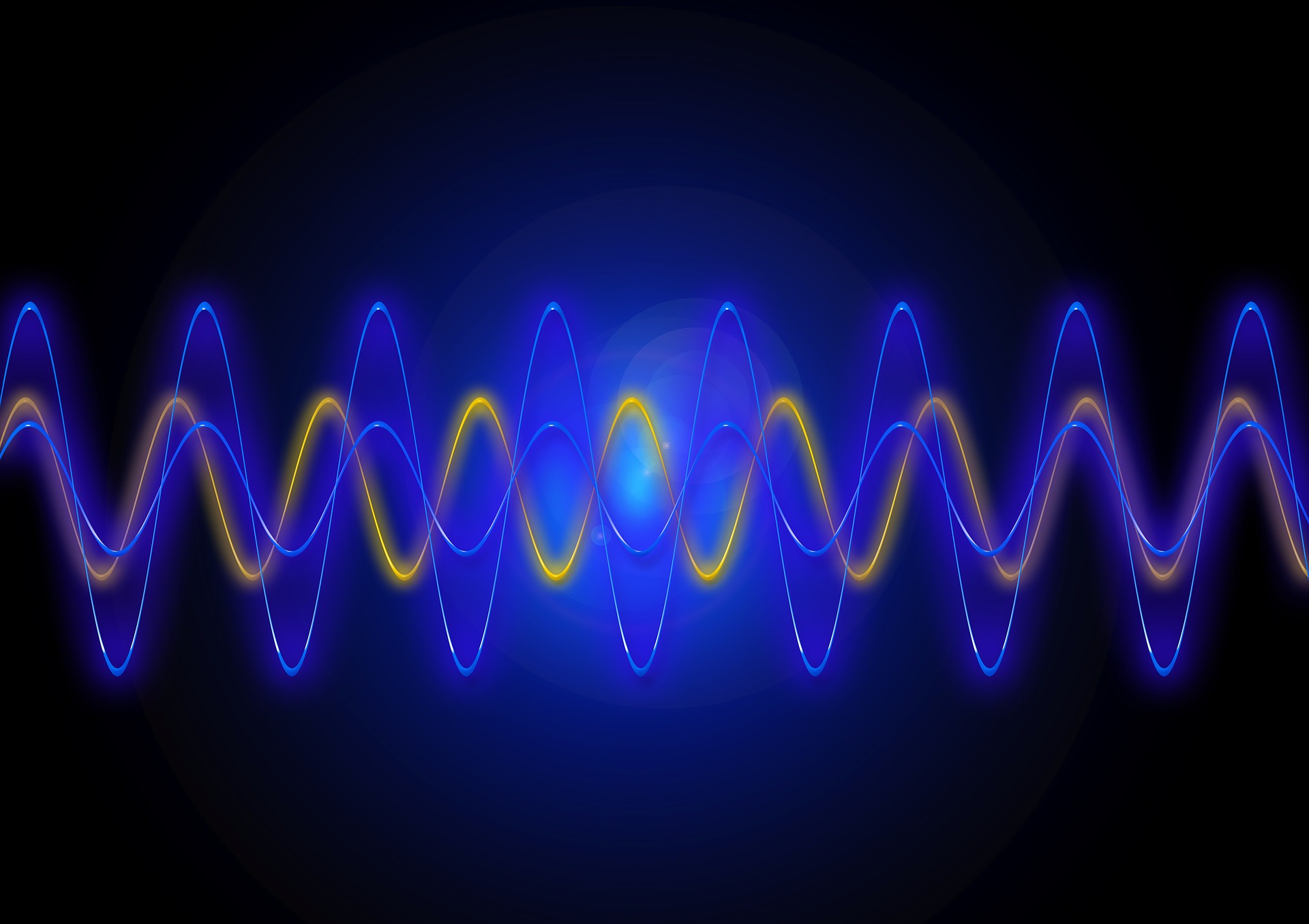
- Read more about IMPROVING BRAIN DECODING METHODS AND EVALUATION
- Log in to post comments
- Categories: