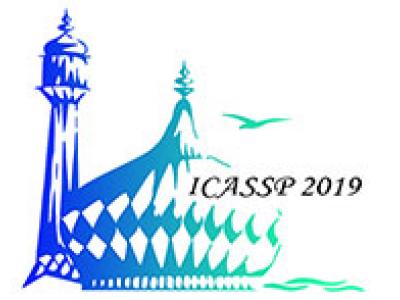
- Read more about Recurrent Neural Networks with Stochastic Layers for Acoustic Novelty Detection
- Log in to post comments
In this paper, we adapt Recurrent Neural Networks with Stochastic Layers, which are the state-of-the-art for generating text, music and speech, to the problem of acoustic novelty detection. By integrating uncertainty into the hidden states, this type of network is able to learn the distribution of complex sequences. Because the learned distribution can be calculated explicitly in terms of probability, we can evaluate how likely an observation is then detect low-probability events as novel.
- Categories:
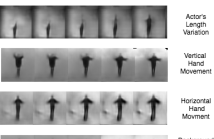
- Read more about LEARNING TEMPORAL INFORMATION FROM SPATIAL INFORMATION USING CAPSNETS FOR HUMAN ACTION RECOGNITION
- Log in to post comments
Capsule Networks (CapsNets) are recently introduced to overcome some of the shortcomings of traditional Convolutional Neural Networks (CNNs). CapsNets replace neurons in CNNs with vectors to retain spatial relationships among the features. In this paper, we propose a CapsNet architecture that employs individual video frames for human action recognition without explicitly extracting motion information. We also propose weight pooling to reduce the computational complexity and improve the classification accuracy by appropriately removing some of the extracted features.
- Categories:
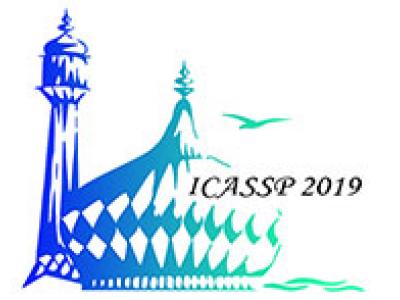
- Read more about Hierarchy-aware Loss Function on a Tree Structured Label Space for Audio Event Detection
- Log in to post comments
The paper introduces a hierarchy-aware loss function in a Deep Neural Network for an audio event detection task that has a bi-level tree structured label space. The goal is not only to improve audio event detection performance at all levels in the label hierarchy, but also to produce better audio embeddings. We exploit the label tree structure to preserve that information in the hierarchy-aware loss function. Two different loss functions are separately employed. First, a triplet loss with probabilistic multi-level batch mining is introduced.
- Categories:
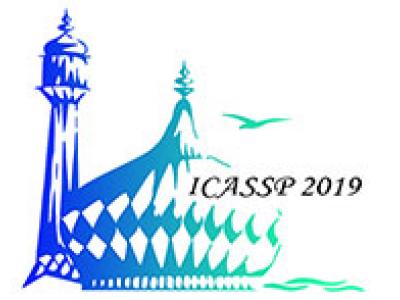
The cloud-based speech recognition/API provides developers or enterprises an easy way to create speech-enabled features in their applications. However, sending audios about personal or company internal information to the cloud, raises concerns about the privacy and security issues. The recognition results generated in cloud may also reveal some sensitive information. This paper proposes a deep polynomial network (DPN) that can be applied to the encrypted speech as an acoustic model. It allows clients to send their data in an encrypted form to the cloud to ensure that their data remains confidential, at mean while the DPN can still make frame-level predictions over the encrypted speech and return them in encrypted form. One good property of the DPN is that it can be trained on unencrypted speech features in the traditional way. To keep the cloud away from the raw audio and recognition results, a cloud-local joint decoding framework is also proposed. We demonstrate the effectiveness of model and framework on the Switchboard and Cortana voice assistant tasks with small performance degradation and latency increased comparing with the traditional cloud-based DNNs.
https://ieeexplore.ieee.org/document/8683721
- Categories:
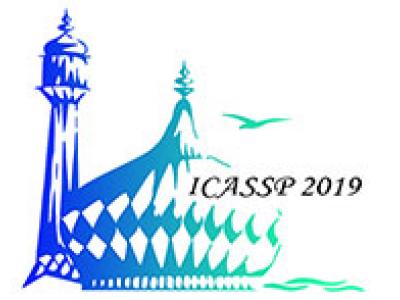
- Read more about CONTINUAL LEARNING FOR ANOMALY DETECTION WITH VARIATIONAL AUTOENCODER
- Log in to post comments
- Categories:
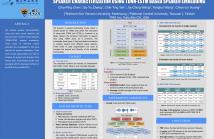
- Read more about SPEAKER CHARACTERIZATION USING TDNN-LSTM BASED SPEAKER EMBEDDING
- Log in to post comments
In this paper we propose speaker characterization using time delay neural networks and long short-term memory neural networks (TDNN-LSTM) speaker embedding. Three types of front-end feature extraction are investigated to find good features for speaker embedding. Three kinds of data augmentation are used to increase the amount and diversity of the training data. The proposed methods are evaluated with the National Institute of Standards and Technology (NIST) speaker recognition evaluation (SRE) tasks.
- Categories:
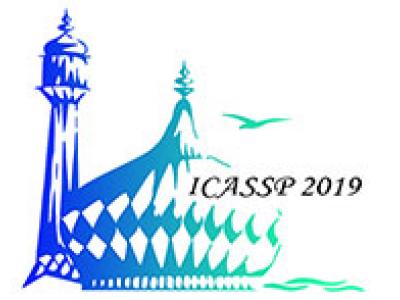
- Categories:
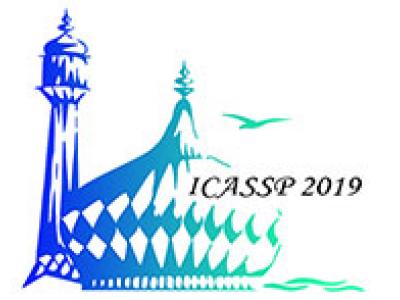
360 camera has recently become popular since it can capture the whole 360 scene. A large number of related applications have been springing up. In this paper, We propose a deep learning based object detector that can be applied directly on 360 images. The proposed detector is based on modifications of the faster RCNN model. Three modification schemes are proposed here, including (1) distortion data augmentation, (2) introducing muilti-kernel layers for improving accuracy for distorted object detection, and (3) adding position information into the model for learning spatial information.
ICASSP_poster300.pdf

- Categories: