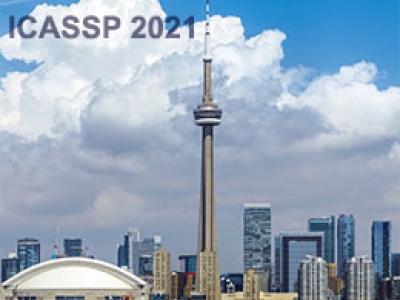
- Read more about Poster : SPARSITY DRIVEN LATENT SPACE SAMPLING FOR GENERATIVE PRIOR BASED COMPRESSIVE SENSING
- 1 comment
- Log in to post comments
- Categories:
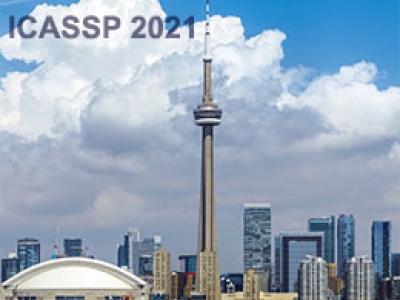
- Read more about Presentation Slides: SPARSITY DRIVEN LATENT SPACE SAMPLING FOR GENERATIVE PRIOR BASED COMPRESSIVE SENSING
- Log in to post comments
- Categories:
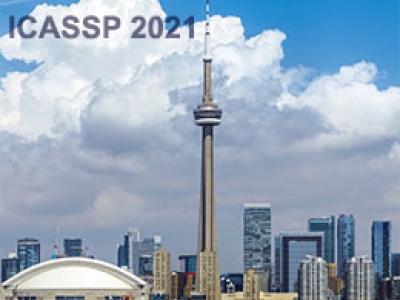
- Read more about Two-Stage Framework for Seasonal Time Series Forecasting (ICASSP 2021 Slides)
- Log in to post comments
- Categories:
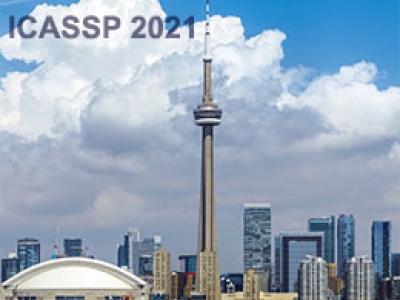
- Read more about Two-Stage Framework for Seasonal Time Series Forecasting (ICASSP 2021 Poster)
- Log in to post comments
- Categories:
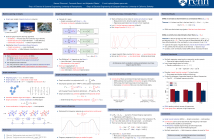
Network data can be conveniently modeled as a graph signal, where data values are assigned to the nodes of a graph describing the underlying network topology. Successful learning from network data requires methods that effectively exploit this graph structure. Graph neural networks (GNNs) provide one such method and have exhibited promising performance on a wide range of problems. Understanding why GNNs work is of paramount importance, particularly in applications involving physical networks.
- Categories:
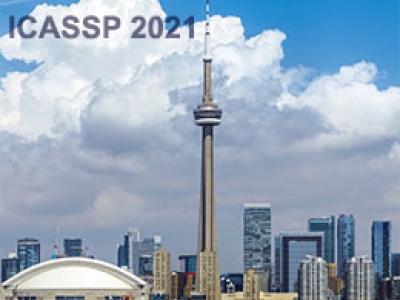
- Read more about SIG2SIG : SIGNAL TRANSLATION NETWORKS TO TAKE THE REMAINS OF THE PAST
- Log in to post comments
- Categories:
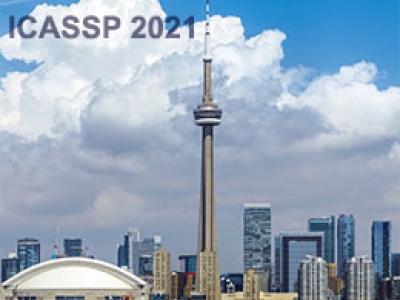
- Read more about Pipeline Safety Early Warning Method for Distributed Signal using Bilinear CNN and Lightgbm
- Log in to post comments
- Categories:
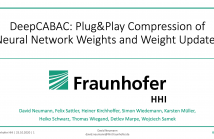
- Read more about DeepCABAC - Plug&Play Compression of Neural Network Weights and Weight Updates
- Log in to post comments
An increasing number of distributed machine learning applications require efficient communication of neural network parameterizations. DeepCABAC, an algorithm in the current working draft of the emerging MPEG-7 part 17 standard for compression of neural networks for multimedia content description and analysis, has demonstrated high compression gains for a variety of neural network models. In this paper we propose a method for employing DeepCABAC in a Federated Learning scenario for the exchange of intermediate differential parameterizations.
- Categories:
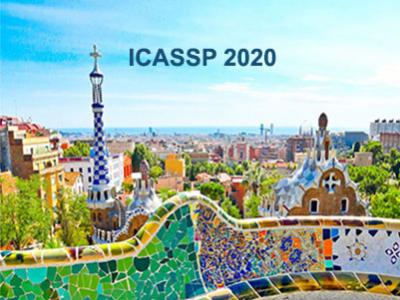
- Read more about PHONEME BOUNDARY DETECTION USING LEARNABLE SEGMENTAL FEATURES
- Log in to post comments
Phoneme boundary detection plays an essential first step for a variety of speech processing applications such as speaker diarization, speech science, keyword spotting, etc. In this work, we propose a neural architecture coupled with a parameterized structured loss function to learn segmental representations for the task of phoneme boundary detection. First, we evaluated our model when the spoken phonemes were not given as input.
- Categories:
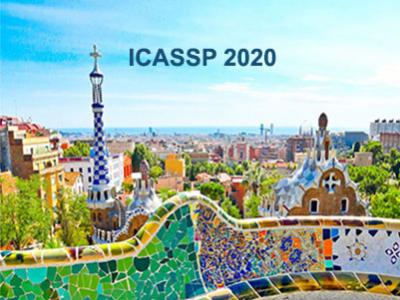
- Read more about Semi-Supervised Optimal Transport Methods for Detecting Anomalies
- Log in to post comments
Building upon advances on optimal transport and anomaly detection, we propose a generalization of an unsupervised and automatic method for detection of significant deviation from reference signals. Unlike most existing approaches for anomaly detection, our method is built on a non-parametric framework exploiting the optimal transportation to estimate deviation from an observed distribution.
- Categories: