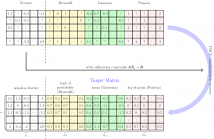
- Read more about Transductive Matrix Completion with Calibration for Multi-Task Learning
- Log in to post comments
Multi-task learning has attracted much attention due to growing multi-purpose research with multiple related data sources. Moreover, transduction with matrix completion is a useful method in multi-label learning. In this paper, we propose a transductive matrix completion algorithm that incorporates a calibration constraint for the features under the multi-task learning framework. The proposed algorithm recovers the incomplete feature matrix and target matrix simultaneously. Fortunately, the calibration information improves the completion results.
- Categories:
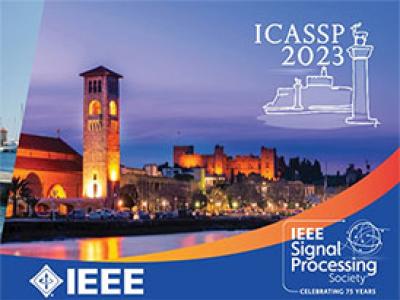
- Categories:
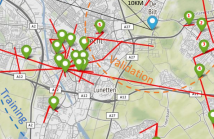
- Read more about Rain Estimation Over a Region Using CycleGan
- Log in to post comments
In the last couple of years, supervised machine learning (ML) methods have shown state-of-the-art results for near-ground rain estimation. Information is usually obtained from two kinds of sensors - rain gauges, which measure rain rate, and commercial microwave links (CMLs) which measure attenuation. These data sources are paired to create a dataset on which a model is trained.
The arising problem of such methods of training is in the need for the datasets to be constructed with a CML-rain gauge pairing relation.
- Categories:
- Read more about Calibrating AI Models for Few-Shot Demodulation via Conformal Prediction
- Log in to post comments
Artificial Intelligent (AI) tools can be useful to address model deficits in the design of communication systems. However, conventional learning-based AI algorithms yield poorly calibrated decisions, unabling to quantify their outputs uncertainty. While Bayesian learning can enhance calibration by capturing epistemic uncertainty caused by limited data availability, formal calibration guarantees only hold under strong assumptions about the ground-truth, unknown, data generation mechanism.
- Categories:
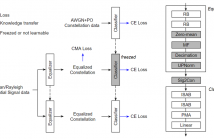
- Read more about EMC²-Net: Joint Equalization and Modulation Classification Based on Constellation Network
- Log in to post comments
Modulation classification (MC) is the first step performed at the receiver side unless the modulation type is explicitly indicated by the transmitter. Machine learning techniques have been widely used for MC recently. In this paper, we propose a novel MC technique dubbed as Joint Equalization and Modulation Classification based on Constellation Network (EMC²-Net). Unlike prior works that considered the constellation points as an image, the proposed EMC²-Net directly uses a set of 2D constellation points to perform MC.
- Categories:
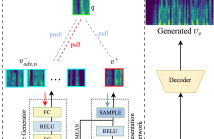
- Read more about CNEG-VC: Contrastive Learning using Hard Negative Example in Non-parallel Voice Conversion
- Log in to post comments
Contrastive learning has advantages for non-parallel voice conversion, but the previous conversion results could be better and more preserved. In previous techniques, negative samples were randomly selected in the features vector from different locations. A positive example could not be effectively pushed toward the query examples. We present contrastive learning in non-parallel voice conversion to solve this problem using hard negative examples. We named it CNEG-VC. Specifically, we teach the generator to generate negative examples. Our proposed generator has specific features.
- Categories:
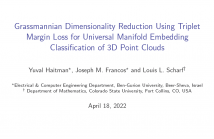
- Read more about Grassmannian Dimensionality Reduction Using Triplet Margin Loss for UME Classification of 3D Point Clouds
- Log in to post comments
- Categories:
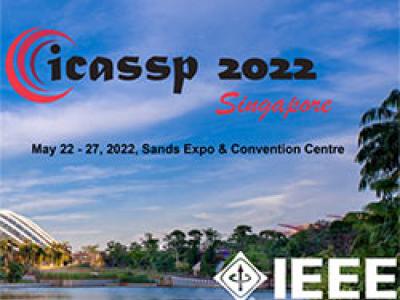
- Read more about NVC-Net: End-to-End Adversarial Voice Conversion
- Log in to post comments
- Categories:
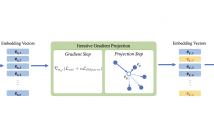
- Read more about BLOCK-SPARSE ADVERSARIAL ATTACK TO FOOL TRANSFORMER-BASED TEXT CLASSIFIERS
- Log in to post comments
Recently, it has been shown that, in spite of the significant performance of deep neural networks in different fields, those are vulnerable to adversarial examples. In this paper, we propose a gradient-based adversarial attack against transformer-based text classifiers. The adversarial perturbation in our method is imposed to be block-sparse so that the resultant adversarial example differs from the original sentence in only a few words. Due to the discrete nature of textual data, we perform gradient projection to find the minimizer of our proposed optimization problem.
- Categories:
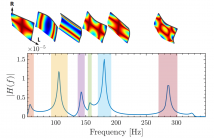
- Read more about On the Prediction of the Frequency Response of a Wooden Plate from its Mechanical Parameters
- Log in to post comments
Inspired by deep learning applications in structural mechanics, we focus on how to train two predictors to model the relation between the vibrational response of a prescribed point of a wooden plate and its material properties. In particular, the eigenfrequencies of the plate are estimated via multilinear regression, whereas their amplitude is predicted by a feedforward neural network.
- Categories: