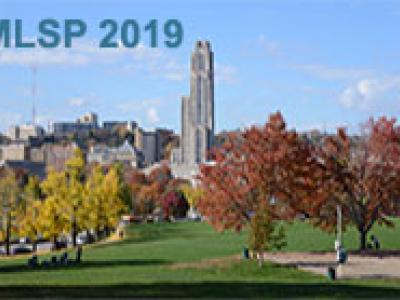
- Read more about HACD: Hierarchical Agglomerative Community Detection in Social Networks
- Log in to post comments
Communities (also referred to as clusters) are essential building blocks of all networks. Hierarchical clustering methods are common graph-based approaches for graph clustering. Traditional hierarchical clustering algorithms proceed in a bottom-up or top-down fashion to encode global information in the graph and cluster according to the global modularity of the graph.
- Categories:
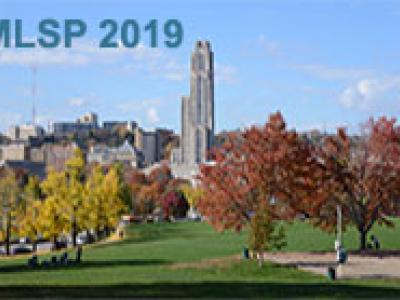
- Read more about Deep Learning for MRI Reconstruction Using a Novel Projection Based Cascaded Network
- Log in to post comments
After their triumph in various classification, recognition and segmentation problems, deep learning and convolutional networks are now making great strides in different inverse problems of imaging. Magnetic resonance image (MRI) reconstruction is an important imaging inverse problem, where deep learning methodologies are starting to make impact. In this work we will develop a new Convolutional Neural Network (CNN) based variant for MRI reconstruction. The developed algorithm is based on the recently proposed deep cascaded CNN (DC-CNN) structure.
- Categories:
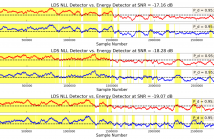
A machine learning approach to detecting unknown signals in time-correlated noise is presented. In the proposed approach, a linear dynamical system (LDS) model is trained to represent the background noise via expectation-maximization (EM). The negative log-likelihood (NLL) of test data under the learned background noise LDS is computed via the Kalman filter recursions, and an unknown signal is detected if the NLL exceeds a threshold. The proposed detection scheme is derived as a generalized likelihood ratio test (GLRT) for an unknown deterministic signal in LDS noise.
poster_mlsp.pdf

- Categories:
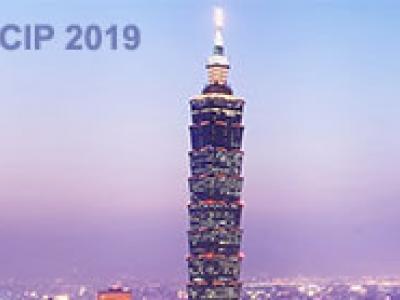
In this study, we propose an efficient approach for modelling and compressing large-scale datasets. The main idea is to subdivide each sample into smaller partitions where each partition constitutes a particular subset of attributes and then apply PCA to each partition separately. This simple approach enjoys several key advantages over the traditional holistic scheme in terms of reduced computational cost and enhanced reconstruction quality.
- Categories:
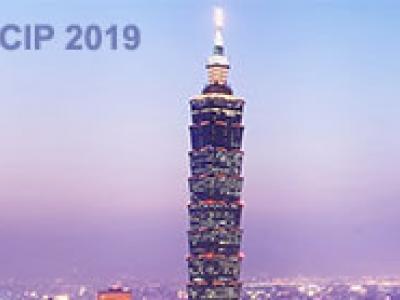
- Read more about High-Accuracy Automatic Person Segmentation with Novel Spatial Saliency Map
- Log in to post comments
In this work, we propose a person segmentation system that achieves high segmentation accuracy with a much smaller CNN network. In this approach, key-point detection annotation is incorporated for the first time and a novel spatial saliency map, in which the intensity of each pixel indicates the likelihood of forming a part of the human and reflects the distance from the body, is generated to provide more spatial information.
- Categories:
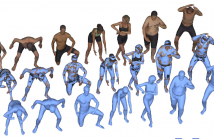
- Read more about BODYFITR: Robust automatic 3D human body fitting
- Log in to post comments
This paper proposes BODYFITR, a fully automatic method to fit a human body model to static 3D scans with complex poses. Automatic and reliable 3D human body fitting is necessary for many applications related to healthcare, digital ergonomics, avatar creation and security, especially in industrial contexts for large-scale product design. Existing works either make prior assumptions on the pose, require manual annotation of the data or have difficulty handling complex poses.
- Categories:
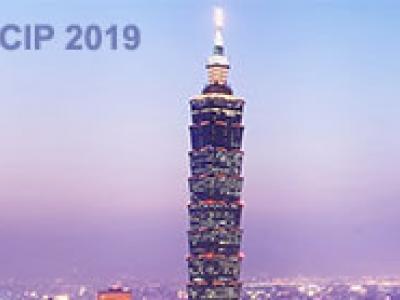
- Read more about MULTIPLE INSTANCE DENSE CONNECTED CONVOLUTION NEURAL NETWORK FOR AERIAL IMAGE SCENE CLASSIFICATION
- Log in to post comments
With the development of deep learning, many state-of-the-art natural image scene classification methods have demonstrated impressive performance. While the current convolution neural network tends to extract global features and global semantic information in a scene, the geo-spatial objects can be located at anywhere in an aerial image scene and their spatial arrangement tends to be more complicated. One possible solution is to preserve more local semantic information and enhance feature propagation.
- Categories:
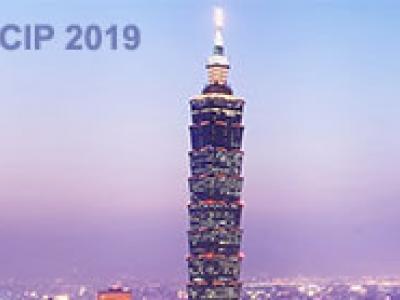
- Read more about Multi-Label Zero-Shot Learning With Transfer-Aware Label Embedding Projection
- Log in to post comments
Zero-Shot learning (ZSL) recently has drawn a lot of attention due to its ability to transfer knowledge from seen classes to novel unseen classes, which greatly reduces human labor of labelling data for building new classifiers. Much effort on ZSL however has focused on the standard multi-class setting, the more challenging multi-label zero-shot problem has received limited attention. In this paper, we propose a transfer-aware embedding projection approach to tackle multi-label zero-shot learning.
- Categories:
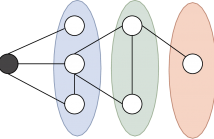
- Read more about Graph Signal Sampling via Reinforcement Learning
- Log in to post comments
We model the sampling and recovery of clustered graph signals as a reinforcement learning (RL) problem. The signal sampling is carried out by an agent which crawls over the graph and selects the most relevant graph nodes to sample. The goal of the agent is to select signal samples which allow for the most accurate recovery. The sample selection is formulated as a multi-armed bandit (MAB) problem, which lends naturally to learning efficient sampling strategies using the well-known gradient MAB algorithm.
- Categories:
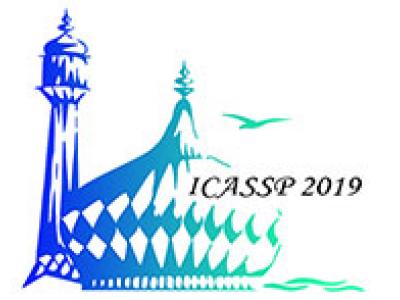
- Read more about FAST COMPRESSIVE SENSING RECOVERY USING GENERATIVE MODELS WITH STRUCTURED LATENT VARIABLES
- Log in to post comments
Deep learning models have significantly improved the visual quality and accuracy on compressive sensing recovery. In this paper, we propose an algorithm for signal reconstruction from compressed measurements with image priors captured by a generative model. We search and constrain on latent variable space to make the method stable when the number of compressed measurements is extremely limited. We show that, by exploiting certain structures of the latent variables, the proposed method produces improved reconstruction accuracy and preserves realistic and non-smooth features in the image.
- Categories: