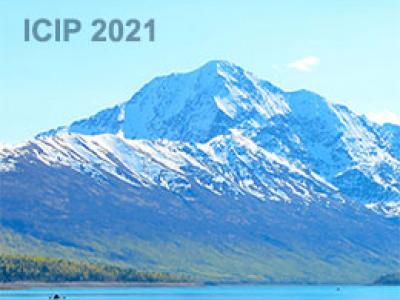
- Read more about ACCURATE COMPENSATION MAKES THE WORLD MORE CLEAR FOR THE VISUALLY IMPAIRED
- Log in to post comments
Visual impairment is one of the most serious social and public health problems in the world, therefore, it is of great theoretical and practical significance to study the image enhancement algorithms for the visually impaired, which is the basis for the development of assistive devices. In this paper, a general deep learning based image enhancement framework for the visually impaired is proposed, which can be used to enhance images to compensate for any visually impaired symptom that can be modeled.
poster_2908.pdf

- Categories:
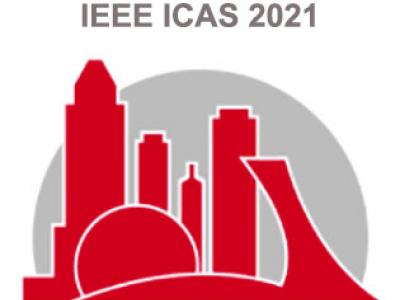
Semantic image segmentation is an important functionality in various applications, such as robotic vision for autonomous cars, drones, etc. Modern Convolutional Neural Networks (CNNs) process input RGB images and predict per-pixel semantic classes. Depth maps have been successfully utilized to increase accuracy over RGB-only input. They can be used as an additional input channel complementing the RGB image, or they may be estimated by an extra neural branch under a multitask training setting.
- Categories:
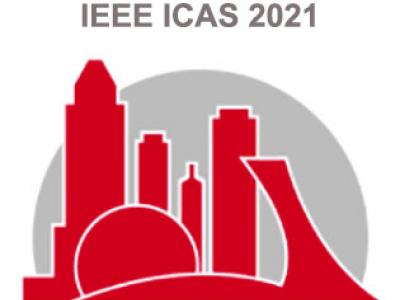
In this study, a cyber-physical system (CPS) for river flow path control is proposed using reinforcement learning. Recently, there has been a frequent occurrence of river flooding due to heavy rains, resulting in serious economic losses and victims. One cause of river flooding is the meandering due to the river bed growing and flow path change. As a mean of avoiding the meandering, river groynes can be used to regularize the flow. However, the mechanism of the flow path growing, and its optimal control is unclear.
- Categories:
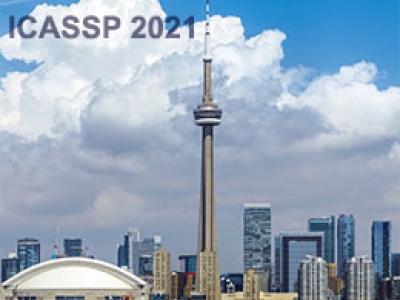
Unmanned aerial vehicles (UAV) often rely on GPS for navigation. GPS signals, however, are very low in power and easily jammed or otherwise disrupted. This paper presents a method for determining the navigation errors present at the beginning of a GPS-denied period utilizing data from a synthetic aperture radar (SAR) system. This is accomplished by comparing an online-generated SAR image with a reference image obtained a priori.
- Categories:
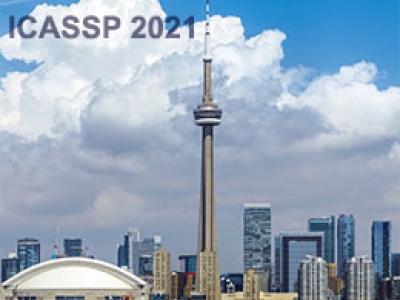
Unmanned aerial vehicles (UAV) often rely on GPS for navigation. GPS signals, however, are very low in power and easily jammed or otherwise disrupted. This paper presents a method for determining the navigation errors present at the beginning of a GPS-denied period utilizing data from a synthetic aperture radar (SAR) system. This is accomplished by comparing an online-generated SAR image with a reference image obtained a priori.
- Categories:
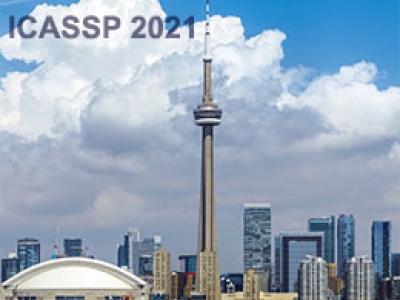
- Read more about KALMANNET: DATA-DRIVEN KALMAN FILTERING
- Log in to post comments
- Categories:
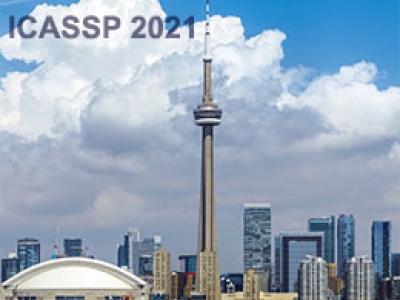
- Read more about STRUCTURED SUPPORT EXPLORATION FOR MULTILAYER SPARSE MATRIX FACTORIZATION
- Log in to post comments
Matrix factorization with sparsity constraints plays an important role in many machine learning and signal processing problems such as dictionary learning, data visualization, dimension reduction. Among the most popular tools for sparse matrix factorization are proximal algorithms, a family of algorithms based on proximal operators. In this paper, we address two problems with the application of proximal algorithms to sparse matrix factorization. On the one hand, we analyze a weakness of proximal algorithms in sparse matrix factorization: the premature convergence of the support.
- Categories:
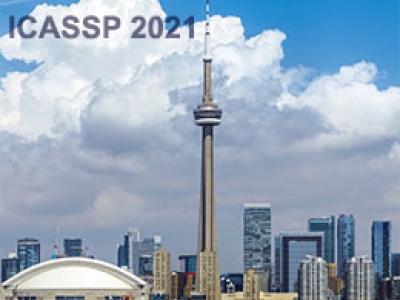
- Categories:
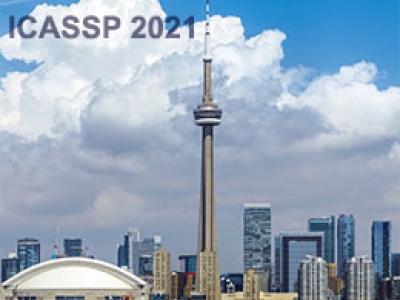
There is growing interest in the use of deep neural network
(DNN) based image denoising to reduce patient’s X-ray
dosage in medical computed tomography (CT). An effective
denoiser must remove noise while maintaining the texture
and detail. Commonly used mean squared error (MSE) loss
functions in the DNN training weight errors due to bias and
variance equally. However, the error due to bias is often more
egregious since it results in loss of image texture and detail.
In this paper, we present a novel approach to designing a loss
- Categories:
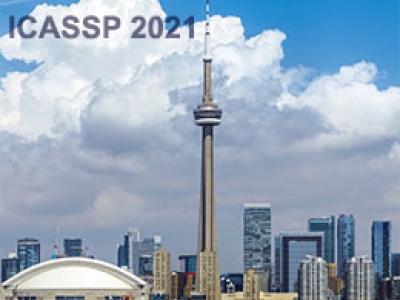
- Read more about Presentation Slides: SPARSITY DRIVEN LATENT SPACE SAMPLING FOR GENERATIVE PRIOR BASED COMPRESSIVE SENSING
- 1 comment
- Log in to post comments
- Categories: