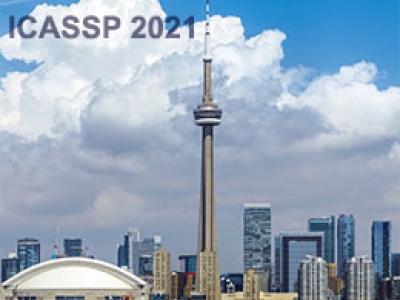
- Read more about Phase recovery with Bregman divergences for audio source separation
- Log in to post comments
Time-frequency audio source separation is usually achieved by estimating the short-time Fourier transform (STFT) magnitude of each source, and then applying a phase recovery algorithm to retrieve time-domain signals. In particular, the multiple input spectrogram inversion (MISI) algorithm has shown good performance in several recent works. This algorithm minimizes a quadratic reconstruction error between magnitude spectrograms.
- Categories:
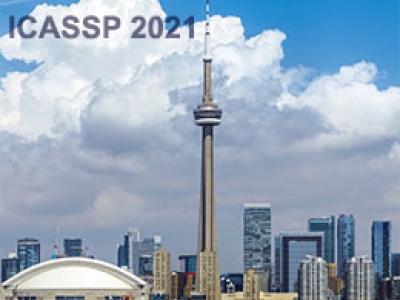
- Read more about SANDGLASSET: A LIGHT MULTI-GRANULARITY SELF-ATTENTIVE NETWORK FOR TIME-DOMAIN SPEECH SEPARATION
- Log in to post comments
One of the leading single-channel speech separation (SS) models is based on a TasNet with a dual-path segmentation technique, where the size of each segment remains unchanged throughout all layers. In contrast, our key finding is that multi-granularity features are essential for enhancing contextual modeling and computational efficiency. We introduce a self-attentive network with a novel sandglass-shape, namely Sandglasset, which advances the state-of-the-art (SOTA) SS performance at significantly smaller model size and computational cost.
- Categories:
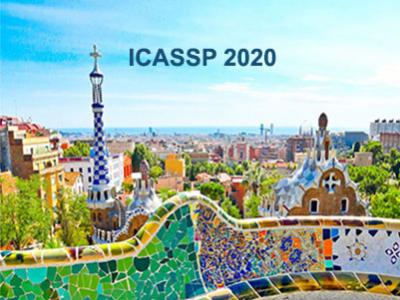
- Read more about Translation of a Higher Order Ambisonics Sound Scene Based on Parametric Decomposition
- Log in to post comments
This paper presents a novel 3DoF+ system that allows to navigate, i.e., change position, in scene-based spatial audio content beyond the sweet spot of a Higher Order Ambisonics recording. It is one of the first such systems based on sound capturing at a single spatial position. The system uses a parametric decomposition of the recorded sound field. For the synthesis, only coarse distance information about the sources is needed as side information but not the exact number of them.
handout.pdf

- Categories:
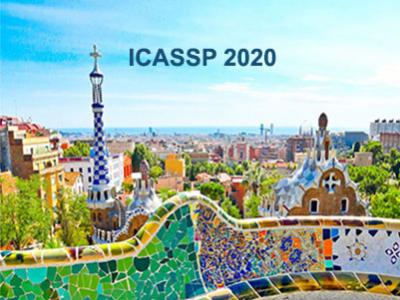
- Read more about Weighted Speech Distortion Losses for Real-time Speech Enhancement
- Log in to post comments
This paper investigates several aspects of training a RNN (recurrent neural network) that impact the objective and subjective quality of enhanced speech for real-time single-channel speech enhancement. Specifically, we focus on a RNN that enhances short-time speech spectra on a single-frame-in, single-frame-out basis, a framework adopted by most classical signal processing methods. We propose two novel mean-squared-error-based learning objectives that enable separate control over the importance of speech distortion versus noise reduction.
- Categories:
- Read more about Generalized Coherence-based Signal Enhancement
- Log in to post comments
This contribution presents a novel approach for coherence-based signal enhancement. An estimator for the coherent-to-diffuse ratio (CDR) is devised, which exploits the concept of generalized magnitude coherence and thus, unlike common state-of-the-art schemes, can simultaneously take advantage of more than two microphones. Moreover, the speech enhancement by CDR-based spectral weighting is not performed as a post-filtering step, but by enhancing the most appropriate microphone signal.
- Categories:
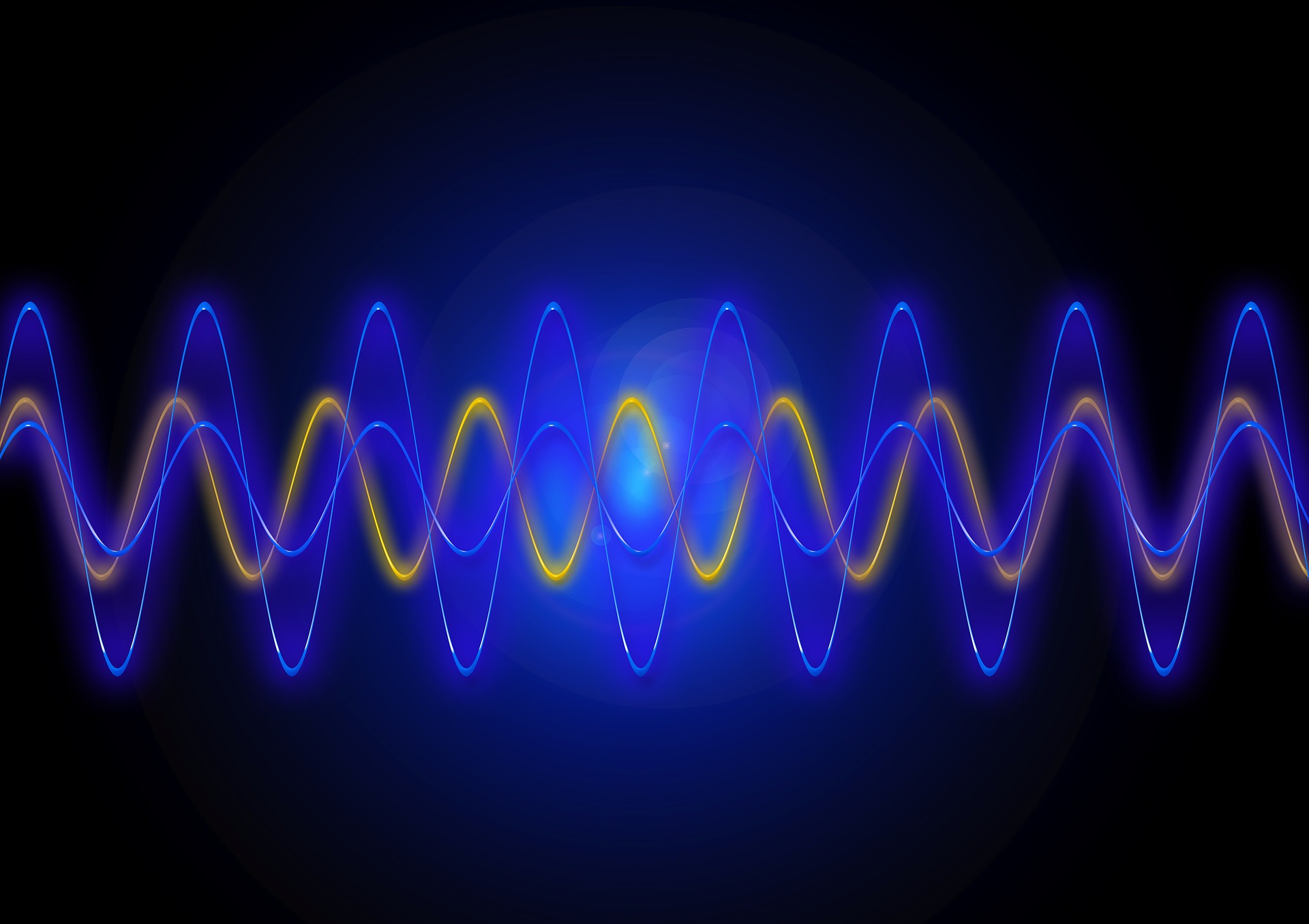
- Read more about Spatially Guided Independent Vector Analysis
- Log in to post comments
- Categories:
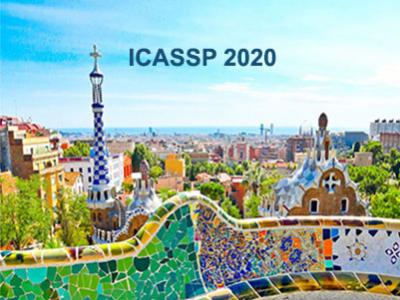
- Read more about Adaptive Blind Audio Source Extraction Supervised by Dominant Speaker Identification using X-vectors
- Log in to post comments
We propose a novel algorithm for adaptive blind audio source extraction. The proposed method is based on independent vector analysis and utilizes the auxiliary function optimization to achieve high convergence speed. The algorithm is partially supervised by a pilot signal related to the source of interest (SOI), which ensures that the method correctly extracts the utterance of the desired speaker. The pilot is based on the identification of a dominant speaker in the mixture using x-vectors. The properties of the x-vectors computed in the presence of cross-talk are experimentally analyzed.
- Categories:
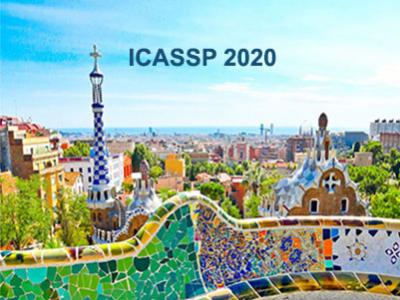
- Read more about ENHANCING END-TO-END MULTI-CHANNEL SPEECH SEPARATION VIA SPATIAL FEATURE LEARNING
- Log in to post comments
Hand-crafted spatial features (e.g., inter-channel phase difference, IPD) play a fundamental role in recent deep learning based multi-channel speech separation (MCSS) methods. However, these manually designed spatial features are hard to incorporate into the end-to-end optimized MCSS framework. In this work, we propose an integrated architecture for learning spatial features directly from the multi-channel speech waveforms within an end-to-end speech separation framework. In this architecture, time-domain filters spanning signal channels are trained to perform adaptive spatial filtering.
- Categories:
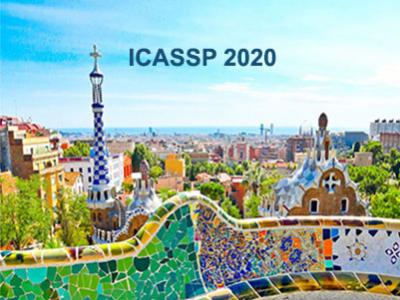
- Read more about Mask-dependent Phase Estimation for Monaural Speaker Separation
- Log in to post comments
Speaker Separation refers to isolating speech of interest in a multi-talker environment. Most methods apply real-valued Time-Frequency (T-F) masks to the mixture Short-Time Fourier Transform (STFT) to reconstruct the clean speech. Hence there is an unavoidable mismatch between the phase of the reconstruction and the original phase of the clean speech. In this paper, we propose a simple yet effective phase estimation network that predicts the phase of the clean speech based on a T-F mask predicted by a chimera++ network.
- Categories:
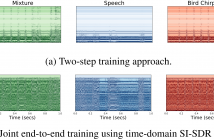
- Read more about Two-Step Sound Source Separation: Training on Learned Latent Targets (Presentation)
- Log in to post comments
In this paper, we propose a two-step training procedure for source separation via a deep neural network. In the first step we learn a transform (and it's inverse) to a latent space where masking-based separation performance using oracles is optimal. For the second step, we train a separation module that operates on the previously learned space. In order to do so, we also make use of a scale-invariant signal to distortion ratio (SI-SDR) loss function that works in the latent space, and we prove that it lower-bounds the SI-SDR in the time domain.
- Categories: