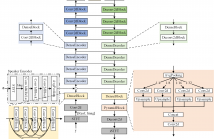
- Read more about DPCCN: Densely-Connected Pyramid Complex Convolutional Network for Robust Speech Separation And Extraction
- Log in to post comments
In recent years, a number of time-domain speech separation methods have been proposed. However, most of them are very sensitive to the environments and wide domain coverage tasks. In this paper, from the time-frequency domain perspective, we propose a densely-connected pyramid complex convolutional network, termed DPCCN, to improve the robustness of speech separation under complicated conditions. Furthermore, we generalize the DPCCN to target speech extraction (TSE) by integrating a new specially designed speaker encoder.
- Categories:
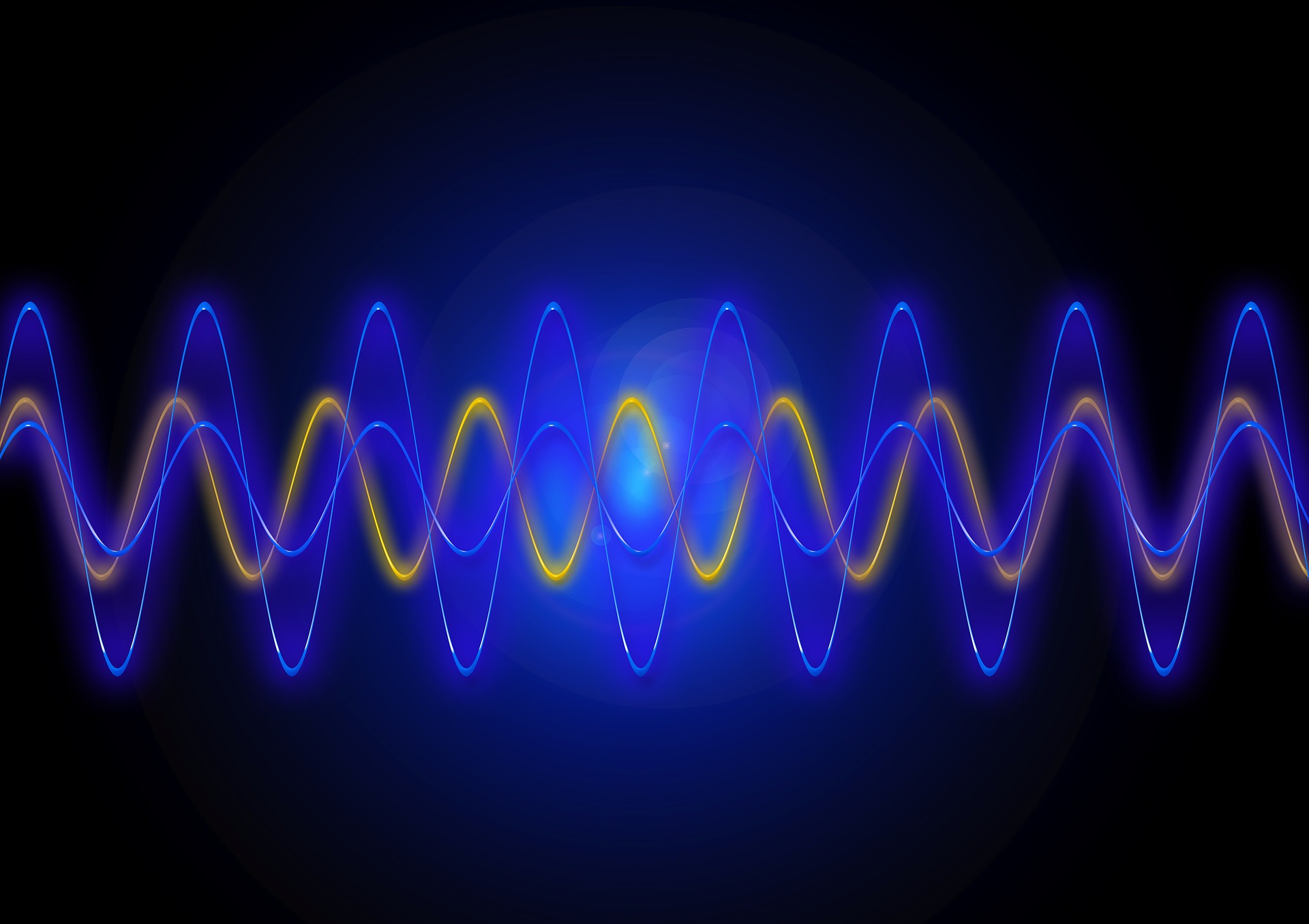
- Read more about EFFECT OF NOISE SUPPRESSION LOSSES ON SPEECH DISTORTION AND ASR PERFORMANCE
- Log in to post comments
Deep learning based speech enhancement has made rapid development towards improving quality, while models are becoming more compact and usable for real-time on-the-edge inference. However, the speech quality scales directly with the model size, and small models are often still unable to achieve sufficient quality. Furthermore, the introduced speech distortion and artifacts greatly harm speech quality and intelligibility, and often significantly degrade automatic speech recognition (ASR) rates. I
- Categories:
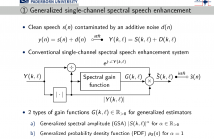
- Read more about A GENERALIZED LOG-SPECTRAL AMPLITUDE ESTIMATOR FOR SINGLE-CHANNEL SPEECH ENHANCEMENT
- Log in to post comments
The benefits of both a logarithmic spectral amplitude (LSA) estimation and a modeling in a generalized spectral domain (where short-time amplitudes are raised to a generalized power exponent, not restricted to magnitude or power spectrum) are combined in this contribution to achieve a better tradeoff between speech quality and noise suppression in single-channel speech enhancement. A novel gain function is derived to enhance the logarithmic generalized spectral amplitudes of noisy speech.
- Categories:
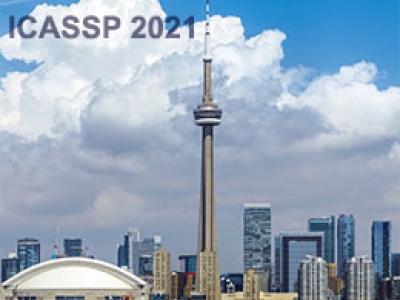
- Read more about AUDITORY FILTERBANKS BENEFIT UNIVERSAL SOUND SOURCE SEPARATION
- Log in to post comments
- Categories:
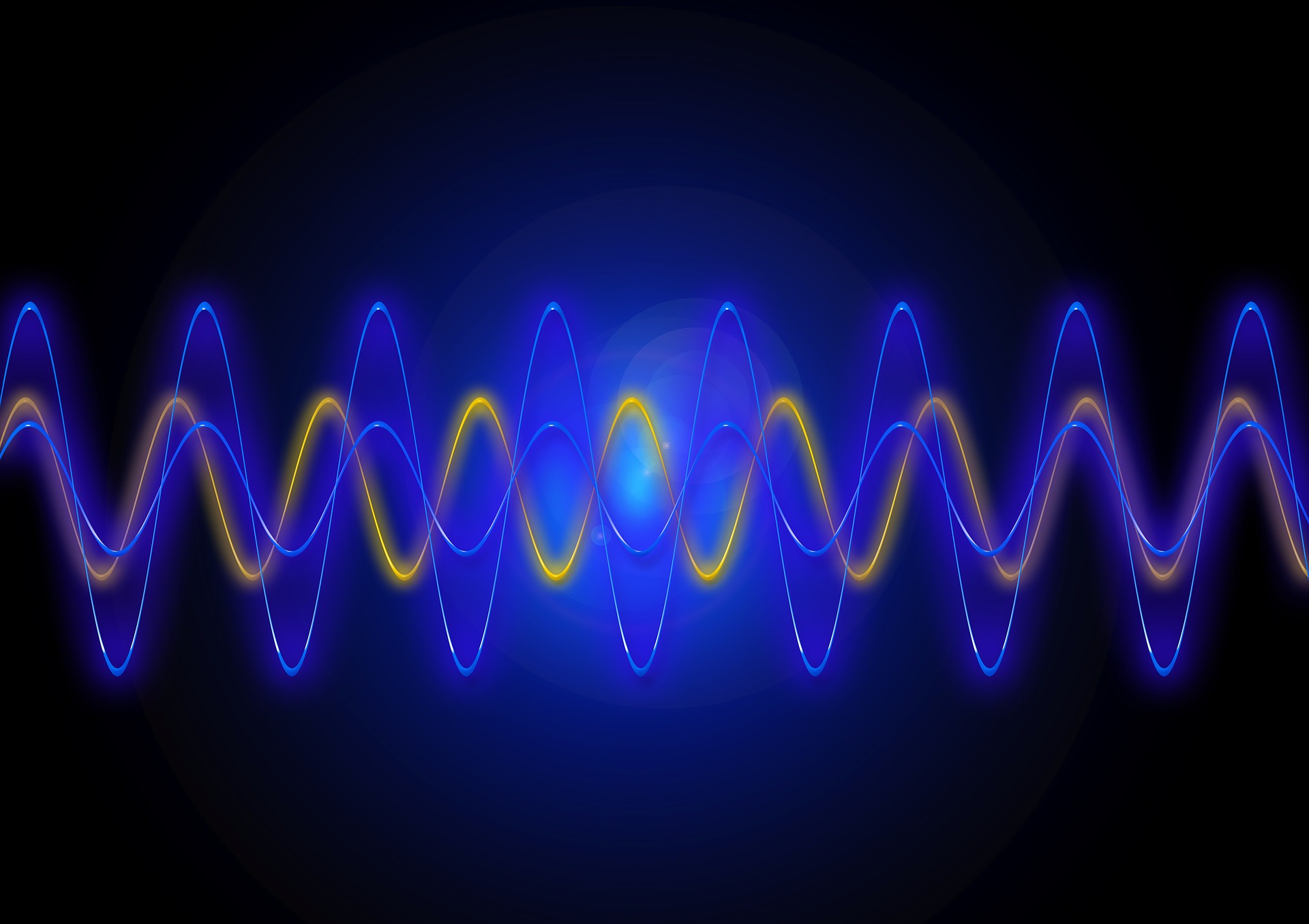
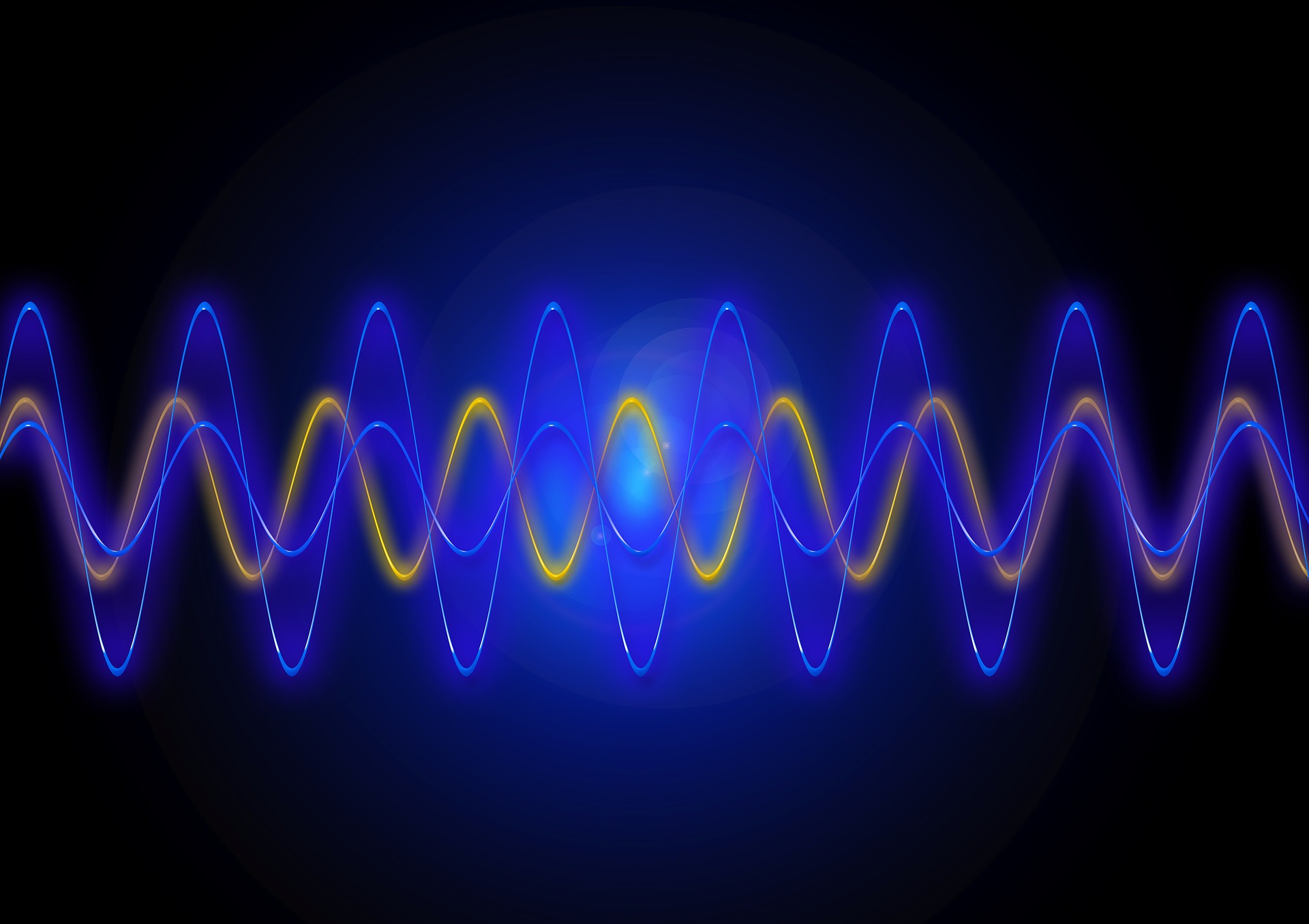
- Read more about Multi-Decoder DPRNN Presentation Slides
- Log in to post comments
- Categories:
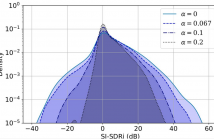
- Read more about (Poster) Unified Gradient Reweighting for Model Biasing with Applications to Source Separation
- Log in to post comments
Recent deep learning approaches have shown great improvement in audio source separation tasks. However, the vast majority of such work is focused on improving average separation performance, often neglecting to examine or control the distribution of the results. In this paper, we propose a simple, unified gradient reweighting scheme, with a lightweight modification to bias the learning process of a model and steer it towards a certain distribution of results. More specifically, we reweight the gradient updates of each batch, using a user-specified probability distribution.
- Categories:
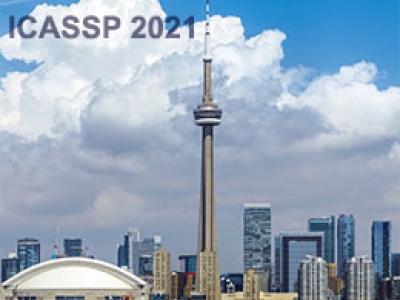
- Read more about Slides: Transcription Is All You Need: Learning To Separate Musical Mixtures With Score As Supervision
- Log in to post comments
Most music source separation systems require large collections of isolated sources for training, which can be difficult to obtain. In this work, we use musical scores, which are comparatively easy to obtain, as a weak label for training a source separation system. In contrast with previous score-informed separation approaches, our system does not require isolated sources, and score is used only as a training target, not required for inference.
- Categories:
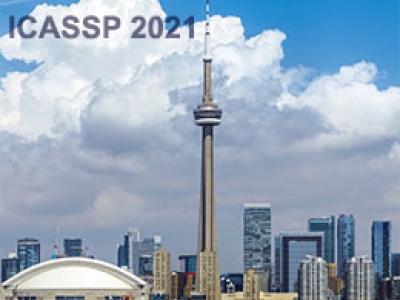
- Read more about Poster: Transcription Is All You Need: Learning To Separate Musical Mixtures With Score As Supervision
- Log in to post comments
Most music source separation systems require large collections of isolated sources for training, which can be difficult to obtain. In this work, we use musical scores, which are comparatively easy to obtain, as a weak label for training a source separation system. In contrast with previous score-informed separation approaches, our system does not require isolated sources, and score is used only as a training target, not required for inference.
- Categories:
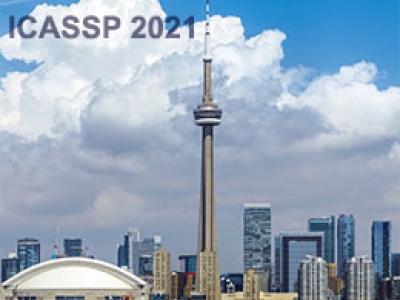
The paper deals with the hitherto neglected topic of audio dequantization. It reviews the state-of-the-art sparsity-based approaches and proposes several new methods. Convex as well as non-convex approaches are included, and all the presented formulations come in both the synthesis and analysis variants. In the experiments the methods are evaluated using the signal-to-distortion ratio (SDR) and PEMO-Q, a perceptually motivated metric.
- Categories: