ICASSP is the world's largest and most comprehensive technical conference on signal processing and its applications. It provides a fantastic networking opportunity for like-minded professionals from around the world. ICASSP 2016 conference will feature world-class presentations by internationally renowned speakers and cutting-edge session topics.
- Read more about Detection of cyclostationarity in the presence of temporal or spatial structure with applications to cognitive radio
- Log in to post comments
Poster.pdf

- Categories:
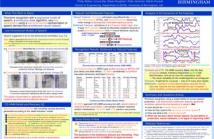
Recent advances in automatic speech recognition have used
large corpora and powerful computational resources to train
complex statistical models from high-dimensional features, to
attempt to capture all the variability found in natural speech.
Such models are difficult to interpret and may be fragile, and
contradict or ignore knowledge of human speech produc-
tion and perception. We report progress towards phoneme
recognition using a model of speech which employs very few
parameters and which is more faithful to the dynamics and
ICASSP2016.pdf

- Categories:
- Read more about Proximity Without Consensus in Online Multi-Agent Optimization -- poster
- Log in to post comments
- Categories:
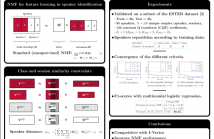
- Read more about Group nonnegative matrix factorisation with speaker and session variability compensation for speaker identification
- Log in to post comments
This paper presents a feature learning approach for speaker identification that is based on nonnegative matrix factorisation. Recent studies have shown that with such models, the dictionary atoms can represent well the speaker identity. The approaches proposed so far focused only on speaker variability and not on session variability. However, this later point is a crucial aspect in the success of the I-vector approach that is now the state-of-the-art in speaker identification.
- Categories:
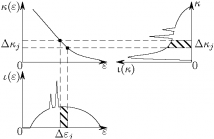
- Read more about Blind polychromatic X-Ray CT reconstruction from Poisson measurements
- Log in to post comments
X-ray sources are polychromatic. Ignoring this fact when performing reconstruction leads to artifacts, such as cupping and streaking, in reconstructed images. We first propose a new model parameterization that allows for blind correction of these artifacts and then develop reconstruction algorithms based on this parameterization.
Here, blind correction means that we do not know
- incident spectrum (which is an X-ray machine characteristic) and
- mass attenuation (inspected material).
poster.pdf

- Categories:
- Read more about MUTUAL INFORMATION BASED RADAR WAVEFORM DESIGN FOR JOINT RADAR AND CELLULAR COMMUNICATION SYSTEMS
- Log in to post comments
- Categories:
- Read more about UNMIXING MULTITEMPORAL HYPERSPECTRAL IMAGES WITH VARIABILITY: AN ONLINE ALGORITHM
- Log in to post comments
Hyperspectral unmixing consists in determining the reference spectral
signatures composing a hyperspectral image and their relative
abundance fractions in each pixel. In practice, the identified signatures
may be affected by a significant spectral variability resulting
for instance from the temporal evolution of the imaged scene. This
phenomenon can be accounted for by using a perturbed linear mixing
model. This paper studies an online estimation algorithm for the
parameters of this extended linear mixing model. This algorithm is
- Categories:
- Read more about ON ADAPTIVE SELECTION OF ESTIMATION BANDWIDTH FOR ANALYSIS OF LOCALLY STATIONARY MULTIVARIATE PROCESSES
- Log in to post comments
This is an overview poster on recent research of adaptive selection of estimation bandwidth for analysis of locally stationary multivariate processes.
- Categories:
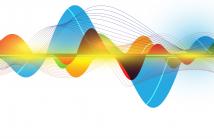
- Read more about Multiplicative Update of AR gains in Codebook-driven Speech Enhancement
- Log in to post comments
- Categories:
- Read more about Ship wake detection for sar images with complex backgrounds based on morphological dictionary learning
- Log in to post comments
- Categories: