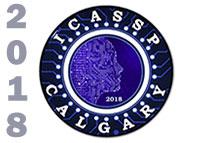
ICASSP is the world’s largest and most comprehensive technical conference focused on signal processing and its applications. The 2019 conference will feature world-class presentations by internationally renowned speakers, cutting-edge session topics and provide a fantastic opportunity to network with like-minded professionals from around the world. Visit website.
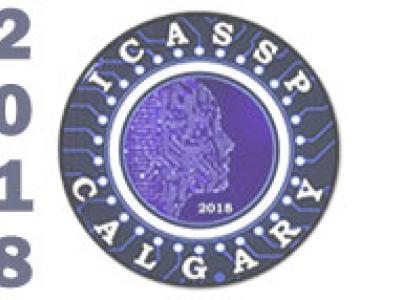
- Read more about NEURAL ADAPTIVE IMAGE DENOISER
- Log in to post comments
We propose a novel neural network-based adaptive image denoiser, dubbased as Neural AIDE. Unlike other neural network-based denoisers, which typically apply supervised training to learn a mapping from a noisy patch to a clean patch, we formulate to train a neural network to learn context- based affine mappings that get applied to each noisy pixel. Our formulation enables using SURE (Stein’s Unbiased Risk Estimator)-like estimated losses of those mappings as empirical risks to minimize.
- Categories:
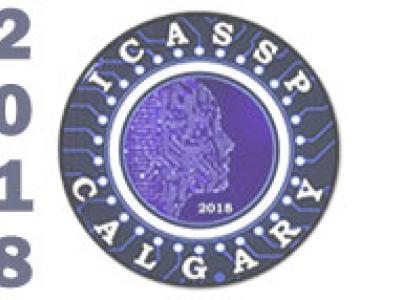
- Read more about DYNAMIC MULTI-RATER GAUSSIAN MIXTURE REGRESSION INCORPORATING TEMPORAL DEPENDENCIES OF EMOTION UNCERTAINTY USING KALMAN FILTERS
- Log in to post comments
Predicting continuous emotion in terms of affective attrib-utes has mainly been focused on hard labels, which ignored the ambiguity of recognizing certain emotions. This ambigu-ity may result in high inter-rater variability and in turn caus-es varying prediction uncertainty with time. Based on the assumption that temporal dependencies occur in the evolu-tion of emotion uncertainty, this paper proposes a dynamic multi-rater Gaussian Mixture Regression (GMR), aiming to obtain the emotion uncertainty prediction reflected by multi-raters by taking into account their temporal dependencies.
- Categories:
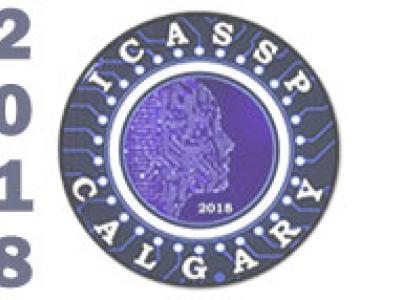
- Read more about Unbiased Distance based Non-local Fuzzy Means
- Log in to post comments
- Categories:
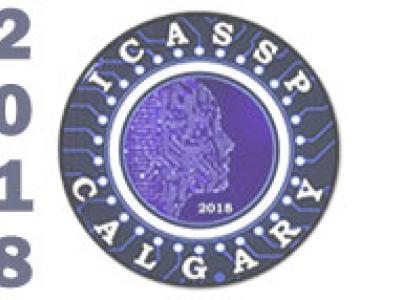
- Read more about UNIVERSAL APPROACH FOR DCT-BASED CONSTANT-TIME GAUSSIAN FILTER WITH MOMENT PRESERVATION
- Log in to post comments
- Categories:
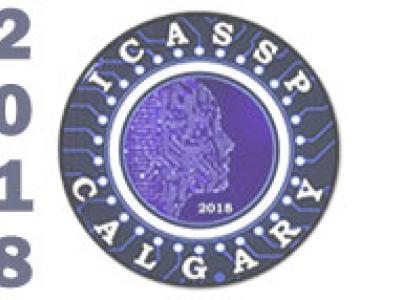
- Read more about Novel Bayesian Cluster Enumeration Criterion For Cluster Analysis With Finite Sample Penalty Term
- Log in to post comments
The Bayesian information criterion is generic in the sense that it does not include information about the specific model selection problem at hand. Nevertheless, it has been widely used to estimate the number of data clusters in cluster analysis. We have recently derived a Bayesian cluster enumeration criterion from first principles which maximizes the posterior probability of the candidate models given observations. But, in the finite sample regime, the asymptotic assumptions made by the criterion, to arrive at a computationally simple penalty term, are violated.
- Categories:
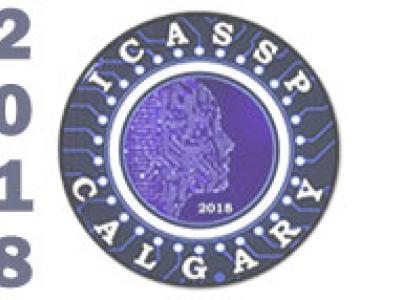
- Read more about Novel Bayesian Cluster Enumeration Criterion For Cluster Analysis With Finite Sample Penalty Term
- Log in to post comments
The Bayesian information criterion is generic in the sense that it does not include information about the specific model selection problem at hand. Nevertheless, it has been widely used to estimate the number of data clusters in cluster analysis. We have recently derived a Bayesian cluster enumeration criterion from first principles which maximizes the posterior probability of the candidate models given observations. But, in the finite sample regime, the asymptotic assumptions made by the criterion, to arrive at a computationally simple penalty term, are violated.
- Categories:
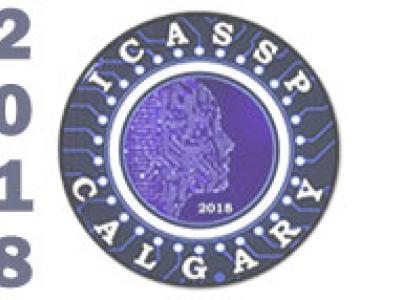
- Read more about Novel Bayesian Cluster Enumeration Criterion For Cluster Analysis With Finite Sample Penalty Term
- Log in to post comments
The Bayesian information criterion is generic in the sense that it does not include information about the specific model selection problem at hand. Nevertheless, it has been widely used to estimate the number of data clusters in cluster analysis. We have recently derived a Bayesian cluster enumeration criterion from first principles which maximizes the posterior probability of the candidate models given observations. But, in the finite sample regime, the asymptotic assumptions made by the criterion, to arrive at a computationally simple penalty term, are violated.
- Categories:
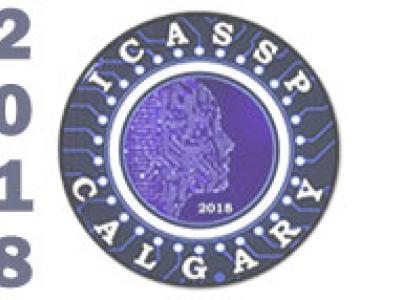
- Read more about FUNCTIONAL CONNECTIVITY STATES OF THE BRAIN USING RESTRICTED BOLTZMANN MACHINES
- Log in to post comments
Recent work on resting-state functional magnetic resonance imaging (rs-fMRI) suggests that functional connectivity (FC) is dynamic. A variety of machine learning and signal processing tools have been applied to the study of dynamic functional connectivity networks (dFCNs) of the brain, by identifying a small number of network states that describe the dynamics of connectivity during rest. Recently, deep learning (DL) methods have been applied to neuroimaging data for learning generative models.
- Categories:
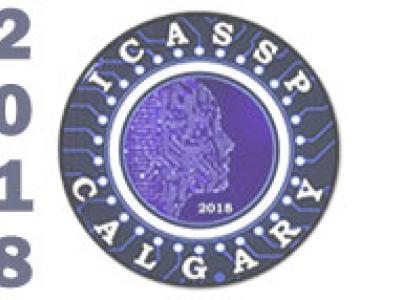
- Read more about A Study of All-Convolutional Encoders for Connectionist Temporal Classification (Poster)
- Log in to post comments
Connectionist temporal classification (CTC) is a popular sequence prediction approach for automatic speech recognition that is typically used with models based on recurrent neural networks (RNNs). We explore whether deep convolutional neural networks (CNNs) can be used effectively instead of RNNs as the "encoder" in CTC. CNNs lack an explicit representation of the entire sequence, but have the advantage that they are much faster to train. We present an exploration of CNNs as encoders for CTC models, in the context of character-based (lexicon-free) automatic speech recognition.
- Categories:
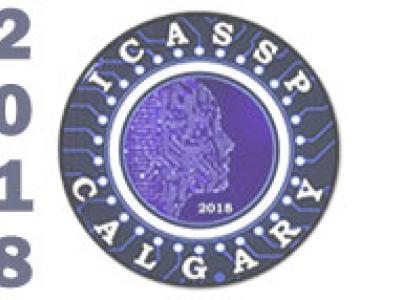
- Read more about ROBUST DETECTION OF JITTERED MULTIPLY REPEATING AUDIO EVENTS USING ITERATED TIME-WARPED ACF
- Log in to post comments
This paper proposes a novel approach for robustly detecting
multiply repeating audio events in monitoring recordings.
We consider the practically important case that
the sequence of inter onset intervals between subsequent events
is not constant but differs by some jitter. In such cases
classical approaches based on autocorrelation (ACF) are
of limited use. To overcome this problem we propose to use
ACF together with a variant of dynamic time warping. Combining
both techniques in an iterative algorithm, we obtain a
- Categories: