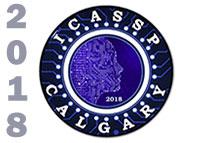
ICASSP is the world’s largest and most comprehensive technical conference focused on signal processing and its applications. The 2019 conference will feature world-class presentations by internationally renowned speakers, cutting-edge session topics and provide a fantastic opportunity to network with like-minded professionals from around the world. Visit website.
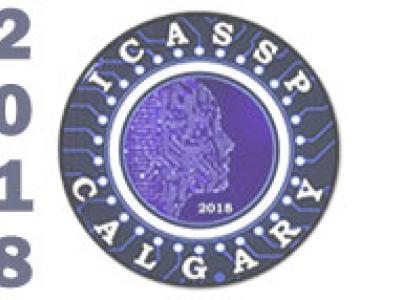
- Read more about Crime incidents embedding using Restricted Boltzmann machine
- Log in to post comments
We present a new approach for detecting related crime series, by unsupervised learning of the latent feature embeddings from narratives of crime record via the Gaussian-Bernoulli Restricted Boltzmann Machines (RBM). This is a drastically different approach from prior work on crime analysis, which typically considers only time and location and at most category information.
- Categories:
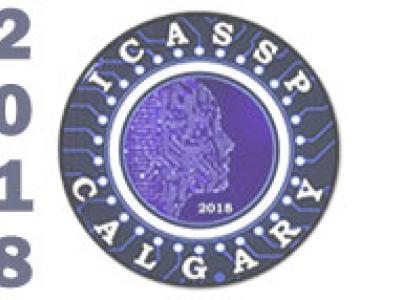
- Read more about EFFECTIVE COVER SONG IDENTIFICATION BASED ON SKIPPING BIGRAMS
- Log in to post comments
So far, few cover song identification systems that utilize index techniques achieve great success. In this paper, we propose a novel approach based on skipping bigrams that could be used for effective index. By applying Vector Quantization, our algorithm encodes signals into code sequences. Then, the bigram histograms of code sequences are used to represent the original recordings and measure their similarities. Through Vector Quantization and skipping bigrams, our model shows great robustness against speed and structure variations in cover songs.
- Categories:
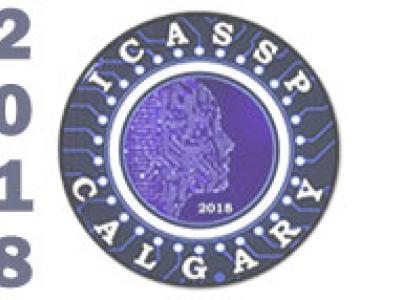
- Read more about SEQUENTIAL ADAPTIVE DETECTION FOR IN-SITU TRANSMISSION ELECTRON MICROSCOPY (TEM)
- Log in to post comments
We develop new efficient online algorithms for detecting transient sparse signals in TEM video sequences, by adopting the recently developed framework for sequential detection jointly with online convex optimization [1]. We cast the problem as detecting an unknown sparse mean shift of Gaussian observations, and develop adaptive CUSUM and adaptive SSRS procedures, which are based on likelihood ratio statistics with post-change mean vector being online maximum likelihood estimators with ℓ1. We demonstrate the meritorious performance of our algorithms for TEM imaging using real data.
icassp2018_poster.pdf

- Categories:
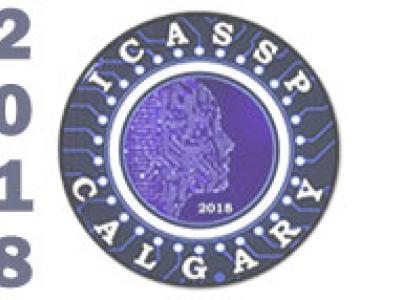
- Read more about Feature LMS Algorithms
- Log in to post comments
In recent years, there is a growing effort in the learning algorithms
area to propose new strategies to detect and exploit
sparsity in the model parameters. In many situations, the
sparsity is hidden in the relations among these coefficients
so that some suitable tools are required to reveal the potential
sparsity. This work proposes a set of LMS-type algorithms,
collectively called Feature LMS (F-LMS) algorithms, setting
forth a hidden feature of the unknown parameters, which ultimately
would improve convergence speed and steady-state
- Categories:
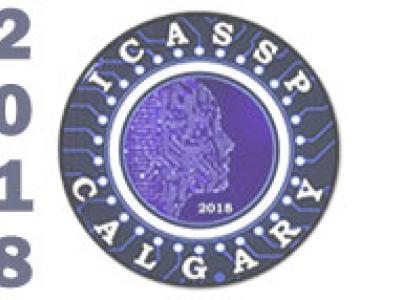
- Read more about Crowdsourcing Emotional Speech
- Log in to post comments
We describe the methodology for the collection and annotation of a large corpus of emotional speech data through crowdsourcing. The corpus offers 187 hours of data from 2,965 subjects. Data includes non-emotional recordings from each subject as well as recordings for five emotions: angry, happy-low-arousal, happy-high-arousal, neutral,
- Categories:
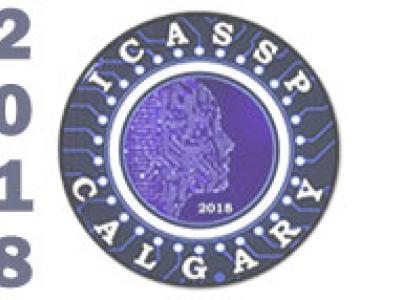
- Read more about RECURRENT NEURAL NETWORKS FOR AUTOMATIC REPLAY SPOOFING ATTACK DETECTION
- Log in to post comments
In order to enhance the security of automatic speaker verification (ASV) systems, automatic spoofing attack detection, which discriminates the fake audio recordings from genuine human speech, has gain much attention recently. Among various ways of spoofing attacks, replay attacks are one of the most effective and economical methods. In this paper, we explore using recurrent neural networks for automatic replay spoofing attack detection.
- Categories:
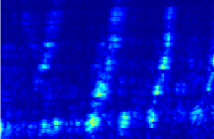
- Read more about FAULT DETECTION USING ATTENTION MODELS BASED ON VISUAL SALIENCY
- Log in to post comments
In this paper, we present an approach for detecting faults within seismic volumes using a saliency detection framework that employs a 3D-FFT local spectra and multi-dimensional plane projections. The projection scheme divides a 3D-FFT local spectrum into three distinct components, each depicting variations along different dimensions of the data. To detect seismic structures oriented at different angles and to capture directional features within 3D volume, we modify the center-surround model to incorporate directional comparisons around each voxel.
- Categories:
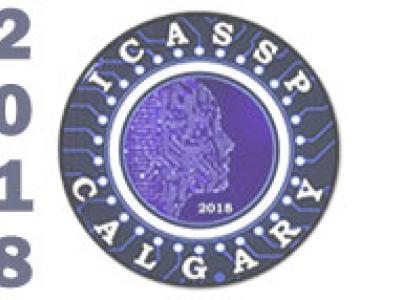
- Read more about EXPONENTIALLY CONSISTENT K-MEANS CLUSTERING ALGORITHM BASED ON KOLMOGROV-SMIRNOV TEST
- Log in to post comments
- Categories:
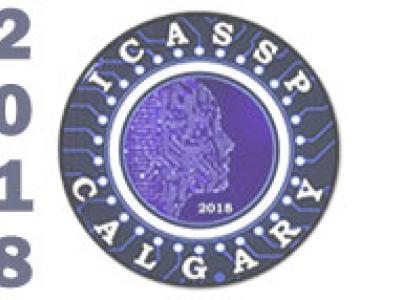
- Read more about A ROTATION-INVARIANT CONVOLUTIONAL NEURAL NETWORK FOR IMAGE ENHANCEMENT FORENSICS
- Log in to post comments
- Categories: