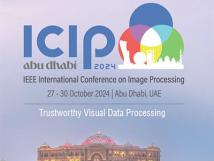
IEEE ICIP 2024 - The International Conference on Image Processing (ICIP), sponsored by the IEEE Signal Processing Society, is the premier forum for the presentation of technological advances and research results in the fields of theoretical, experimental, and applied image and video processing. ICIP has been held annually since 1994, brings together leading engineers and scientists in image and video processing from around the world. Visit website.
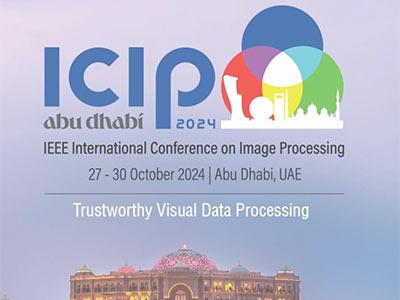
- Read more about Non-separable Wavelet Transform Using Learnable Convolutional Lifting Steps
- Log in to post comments
Wavelet transforms have been a relevant topic in signal processing for many years. One of the most common strategies when designing wavelet transforms is the use of lifting schemes, known for their perfect reconstruction properties and flexible design. This paper introduces a novel 2D non-separable lifting design methodology based on deep learning architectures. The proposed method is assessed within the context of end-to-end lossless image compression.
- Categories:
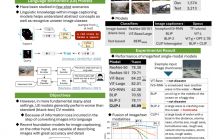
- Read more about Reading Is Believing: Revisiting Language Bottleneck Models for Image Classification
- Log in to post comments
We revisit language bottleneck models as an approach to ensuring the explainability of deep learning models for image classification. Because of inevitable information loss incurred in the step of converting images into language, the accuracy of language bottleneck models is considered to be inferior to that of standard black-box models. Recent image captioners based on large-scale foundation models of Vision and Language, however, have the ability to accurately describe images in verbal detail to a degree that was previously believed to not be realistically possible.
- Categories:
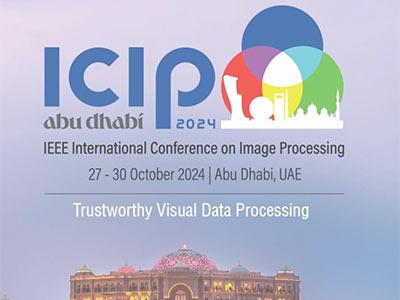
- Read more about Rethinking temporal self-similarity for repetitive action counting
- Log in to post comments
Counting repetitive actions in long untrimmed videos is a challenging task that has many applications such as rehabilitation.
- Categories:
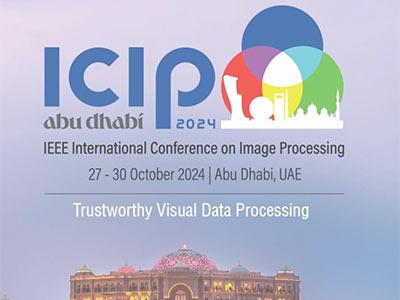
- Read more about On the exploitation of DCT-traces in the Generative-AI domain
- 1 comment
- Log in to post comments
Deepfakes represent one of the toughest challenges in the world of Cybersecurity and Digital Forensics, especially considering the high-quality results obtained with recent generative AI-based solutions. Almost all generative models leave unique traces in synthetic data that, if analyzed and identified in detail, can be exploited to improve the generalization limitations of existing deepfake detectors.
- Categories:
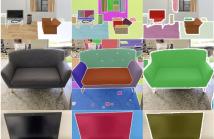
- Read more about Segment Any Object Model (SAOM): Real-to-Simulation Fine-Tuning Strategy for Multi-Class Multi-Instance Segmentation
- Log in to post comments
Multi-class multi-instance segmentation is the task of identifying masks for multiple object classes and multiple instances of the same class within an image. The Segment Anything Model (SAM) is a new foundation model designed for promptable multi-class multi-instance segmentation. SAM is able to segment objects in any image using a pre-defined point grid as an input prompt in the ``everything'' mode. However, out of the box SAM tends to output part or sub-part segmentation masks (under-segmentation) in different real-world applications.
- Categories:
Pages
- « first
- ‹ previous
- 1
- 2
- 3
- 4