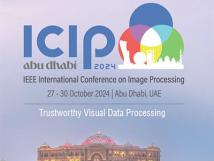
IEEE ICIP 2024 - The International Conference on Image Processing (ICIP), sponsored by the IEEE Signal Processing Society, is the premier forum for the presentation of technological advances and research results in the fields of theoretical, experimental, and applied image and video processing. ICIP has been held annually since 1994, brings together leading engineers and scientists in image and video processing from around the world. Visit website.
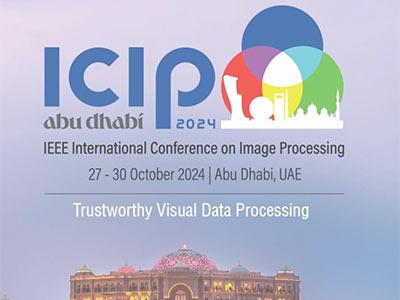
- Read more about Fast Unsupervised Tensor Restoration via Low-rank Deconvolution
- Log in to post comments
Low-rank Deconvolution (LRD) has appeared as a new multi-dimensional representation model that enjoys important efficiency and flexibility properties. In this work we ask ourselves if this analytical model can compete against Deep Learning (DL) frameworks like Deep Image Prior (DIP) or Blind-Spot Networks (BSN) and other classical methods in the task of signal restoration. More specifically, we propose to extend LRD with differential regularization.
- Categories:
- Read more about RESSCAL3D++: Joint Acquisition and Semantic Segmentation of 3D Point Clouds
- Log in to post comments
3D scene understanding is crucial for facilitating seamless interaction between digital devices and the physical world. Real-time capturing and processing of the 3D scene are essential for achieving this seamless integration. While existing approaches typically separate acquisition and processing for each frame, the advent of resolution-scalable 3D sensors offers an opportunity to overcome this paradigm and fully leverage the otherwise wasted acquisition time to initiate processing.
- Categories:
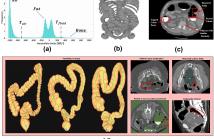
- Read more about Accurate colon segmentation using 2D convolutional neural networks with 3D contextual information
- Log in to post comments
This study introduces an innovative framework for accurate colon segmentation in abdomen CT scans, addressing the unique challenges of this task. Our architecture enhances well-established 2D segmentation models by incorporating 3D contextual information through a novel method that generates an attention map for a given slice by considering its neighboring slices. This approach achieves effective colon segmentation without complex 3D convolutional neural networks (CNNs) or Long Short-Term Memory (LSTM) networks by combining 2D CNNs.
- Categories:

- Read more about CORRELATION-AWARE JOINT PRUNING-QUANTIZATION USING GRAPH NEURAL NETWORKS
- Log in to post comments
Deep learning in image classification has achieved remarkable success but at the cost of high resource demands. Model compression through automatic joint pruning-quantization addresses this issue, yet most existing techniques overlook a critical aspect: layer correlations. These correlations are essential as they expose redundant computations across layers, and leveraging them facilitates efficient design space exploration. This study employs Graph Neural Networks (GNN) to learn these inter-layer relationships, thereby optimizing the pruning-quantization strategy for the targeted model.
- Categories:
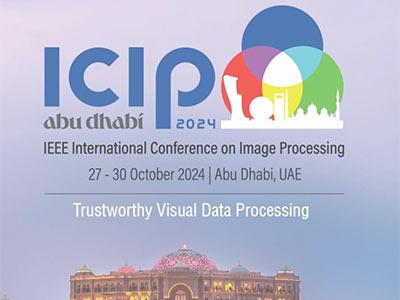
- Read more about TEMPORAL TRANSFORMER ENCODER FOR VIDEO CLASS INCREMENTAL LEARNING
- Log in to post comments
Current video classification approaches suffer from catastrophic forgetting when they are retrained on new databases.
Continual learning aims to enable a classification system with learning from a succession of tasks without forgetting.
In this paper we propose to use a transformer-based video class incremental learning model. During a succession of
learning steps, at each training time, the transformer is used to extract characteristic spatio-temporal features from videos
- Categories:
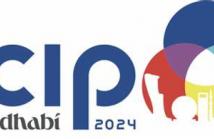
- Read more about LIGHTWEIGHT UNDERWATER IMAGE ENHANCEMENT VIA IMPULSE RESPONSE OF LOW-PASS FILTER BASED ATTENTION NETWORK
- Log in to post comments
In this paper, we propose an improved model of Shallow-UWnet for underwater image enhancement. In the proposed method, we enhance the learning process and solve the vanishing gradient problem by a skip connection, which concatenates the raw underwater image and the low-pass filter (LPF) impulse response into Shallow-UWnet. Additionally, we integrate the simple, parameter-free attention module (SimAM) into each Convolution Block to enhance the visual quality of images.
- Categories:
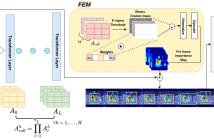
- Read more about ET: Explain to Train: Leveraging Explanations to Enhance the Training of A Multimodal Transformer
- Log in to post comments
Explainable Artificial Intelligence (XAI) has become increasingly vital for improving the transparency and reliability of neural network decisions. Transformer architectures have emerged as the state-of-the-art for various tasks across single modalities such as video, language, or signals, as well as for multimodal approaches. Although XAI methods for transformers are available, their potential impact during model training remains underexplored.
- Categories:
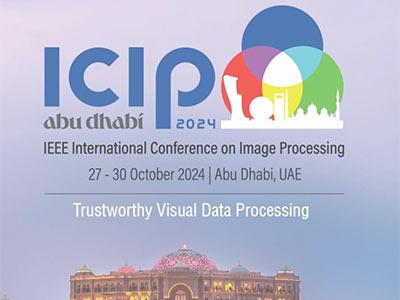
- Read more about A Needle In A (Medical) Haystack: Detecting A Biopsy Needle In Ultrasound Images Using Vision Transformers
- Log in to post comments
Needle localization in ultrasound images is pivotal for the successful execution of ultrasound-guided core needle biopsies. Automating the needle detection process can decrease the procedure time and lead to a more precise diagnosis. In this article, we introduce an automatic method for detecting the core needle and determining its trajectory in 2D ultrasound images.
- Categories:
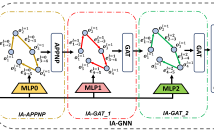
- Read more about Driving through Graphs: A Bipartite Graph for Traffic Scene Analysis
- Log in to post comments
We introduce a novel approach for traffic scene analysis in driving videos by exploring spatio-temporal relationships captured by a temporal frame-to-frame (f2f) bipartite graph, eliminating the need for complex image-level high-dimensional feature extraction. Instead, we rely on object detectors that provide bounding box information. The proposed graph approach efficiently connects objects across frames where nodes represent essential object attributes, and edges signify interactions based on simple spatial metrics such as distance and angles between objects.
- Categories:
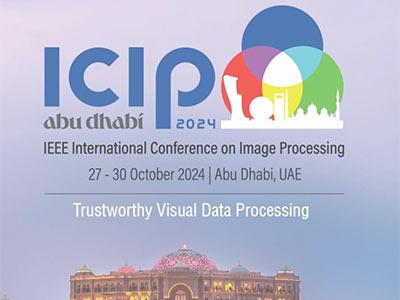
- Read more about Redefining Visual Quality: The Impact of Loss Functions on INR-Based Image Compression
- Log in to post comments
Implicit Neural Representations (INR) are a novel data representation technique which is gaining ground in the image compression field due to its simplicity and interesting results in terms of rate/distortion ratio.
- Categories: