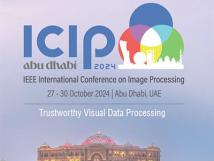
IEEE ICIP 2024 - The International Conference on Image Processing (ICIP), sponsored by the IEEE Signal Processing Society, is the premier forum for the presentation of technological advances and research results in the fields of theoretical, experimental, and applied image and video processing. ICIP has been held annually since 1994, brings together leading engineers and scientists in image and video processing from around the world. Visit website.

- Read more about Adversarial Robustness for Deep Metric Learning
- Log in to post comments
Deep Metric Learning (DML) based on Convolutional Neural Networks (CNNs) is vulnerable to adversarial attacks. Adversarial training, where adversarial samples are generated at each iteration, is one of the prominent defense techniques for robust DML. However, adversarial training increases computational complexity and causes a trade-off between robustness and generalization. This study proposes a lightweight, robust DML framework that learns a non-linear projection to map the embeddings of a CNN into an adversarially robust space.
- Categories:
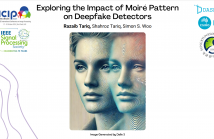
Deepfake detection is critical in mitigating the societal threats posed by manipulated videos. While various algorithms have been developed for this purpose, challenges arise when detectors operate externally, such as on smartphones, when users take a photo of deepfake images and upload on the Internet. One significant challenge in such scenarios is the presence of Moire patterns, which degrade image quality and confound conventional classification algorithms, including deep neural networks (DNNs). The impact of Moire patterns remains largely unexplored for deepfake detectors.
- Categories:
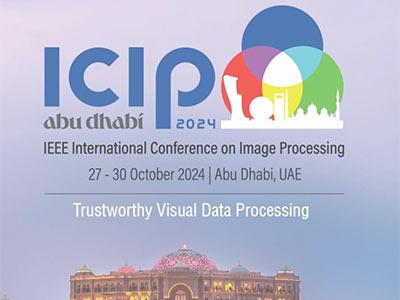
- Read more about Giraffe: A Genetic Programming Algorithm To Build Deep Learning Ensembles For Ecg Arrhythmia Classification
- Log in to post comments
Cardiovascular diseases remain one of the leading causes of death worldwide. Therefore, developing and validating automated tools to help identify high-risk patients are of paramount clinical utility. In this article, we tackle this task and introduce a genetic programming algorithm (called GIRAFFE) to build (deep) machine learning classification ensembles for arrhythmia classification from two-dimensional images of 12-lead electrocardiogram (ECG) tracings.
- Categories:
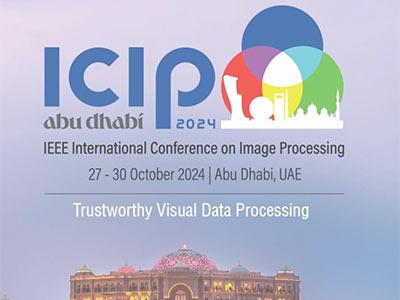
- Read more about FUSION OF INDEPENDENT AND INTERACTIVE FEATURES FOR HUMAN-OBJECT INTERACTION DETECTION
- Log in to post comments
Human-Object Interaction (HOI) detection, which aims to identify humans and objects with interactive behaviors in images and predict the behaviors between them, is of great significance for semantic understanding. The existing works primarily focus on exploring the fine-grained semantic features of humans and objects, as well as the spatial relationships between them. However, these methods do not leverage the contextual information within the interaction area, which could potentially be valuable for predicting interaction behavior.
- Categories:

- Read more about Unrolled Projected Gradient Algorithm for Stain Separation in Digital Histopathological Images
- Log in to post comments
This paper introduces a novel optimization approach for stain separation in digital histopathological images. Our stain separation cost function incorporates a smooth total variation regularization and is minimized by using a projected gradient algorithm. To enhance computational efficiency and enable supervised learning of the hyperparameters, we further unroll our algorithm into a neural network. The unrolled architecture is not only more efficient for solving the stain separation problem, but also allows to design a highly interpretable and flexible method.
- Categories:
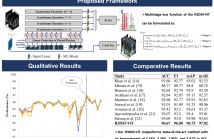
- Read more about Recurrent 3-D Multi-level Visual Transformer for Joint Classification of Heterogeneous 2-D and 3-D Radiographic Data
- 1 comment
- Log in to post comments
Recent advancements in artificial intelligence algorithms for medical imaging show significant potential in automating the detection of lung infections from chest radiograph scans. However, current approaches often focus solely on either 2-D or 3-D scans, failing to leverage the combined advantages of both modalities. Moreover, conventional slice-based methods place a manual burden on radiologists for slice selection.
- Categories:
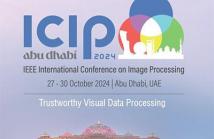
- Read more about City Traffic Aware Multi-Target Tracking Prediction With Multi-Camera
- Log in to post comments
In recent years, Multi-Camera Multiple Object Tracking (MCMT) has gained significant attention as a crucial computer vision application. Research focuses on data association and track detection. However, accurately selecting datasets from raw vision data remains challenging due to real-world complexities like object types, varying speeds, and unknown directions. To address these problems, this paper proposes the Object Tracking Model (OTM) to capture the feature of target area with the Camera Monitoring Network (CMN) based on Graph Convolutional Network (GCN).
- Categories:
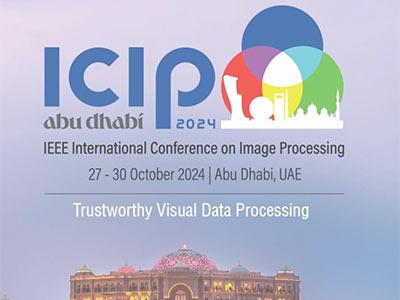
- Read more about Gumbel-NeRF: Representing Unseen Objects as Part-Compositional Neural Radiance Fields
- Log in to post comments
We propose Gumbel-NeRF, a mixture-of-expert (MoE) neural radiance fields (NeRF) model with a hindsight expert selection mechanism for synthesizing novel views of unseen objects. Previous studies have shown that the MoE structure provides high-quality representations of a given large-scale scene consisting of many objects. However, we observe that such a MoE NeRF model often produces low-quality representations in the vicinity of experts’ boundaries when applied to the task of novel view synthesis of an unseen object from one/few-shot input.
- Categories:
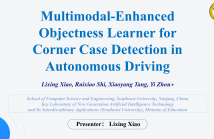
- Read more about Multimodal-Enhanced Objectness Learner for Corner Case Detection in Autonomous Driving
- 1 comment
- Log in to post comments
Previous works on object detection have achieved high accuracy in closed-set scenarios, but their performance in open-world scenarios is not satisfactory. One of the challenging open-world problems is corner case detection in autonomous driving. Existing detectors struggle with these cases, relying heavily on visual appearance and exhibiting poor generalization ability. In this paper, we propose a solution by reducing the discrepancy between known and unknown classes and introduce a multimodal-enhanced objectness notion learner.
- Categories:
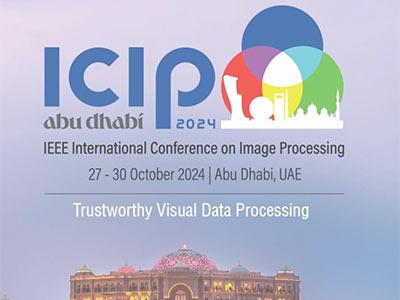
- Read more about ON THE DETECTION OF IMAGES GENERATED FROM TEXT
- Log in to post comments
The introduction of diverse text-to-image generation models has sparked significant interest across various sectors. While these models provide the groundbreaking capability to convert textual descriptions into visual data, their widespread usage has ignited concerns over misusing realistic synthesized images. Despite the pressing need, research on detecting such synthetic images remains limited. This paper aims to bridge this gap by evaluating the ability of several existing detectors to detect synthesized images produced by text-to-image generation models.
- Categories: