- Image/Video Storage, Retrieval
- Image/Video Processing
- Image/Video Coding
- Image Scanning, Display, and Printing
- Image Formation
- Read more about News Story Clustering with Fisher Embedding
- Log in to post comments
An automatic news story clustering system is presented to facilitate efficient news browsing and summarization. We describe news content by considering both what objects appear and how these objects move in news stories. With Fisher embedding, we respectively encode local features, semantics features, and dense trajectories as Fisher vectors, based on which similarity between news stories can be well evaluated and thus better clustering performance can be obtained.
- Categories:
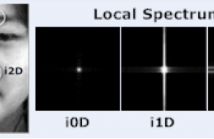
- Read more about Intrinsic Two-Dimensional Local Structures for Micro-Expression Recognition
- Log in to post comments
An elapsed facial emotion involves changes of facial contour due to the motions (such as contraction or stretch) of facial muscles located at the eyes, nose, lips and etc. Thus, the important information such as corners of facial contours that are located in various regions of the face are crucial to the recognition of facial expressions, and even more apparent for micro-expressions. In this paper, we propose the first known notion of employing intrinsic two-dimensional (i2D) local structures to represent these features for micro-expression recognition.
- Categories:
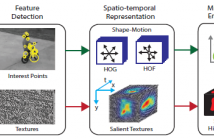
- Read more about Spatio-Temporal Mid-Level Feature Bank for Action Recognition in Low Quality Video
- Log in to post comments
It is a great challenge to perform high level recognition tasks on videos that are poor in quality. In this paper, we propose a new spatio-temporal mid-level (STEM) feature bank for recognizing human actions in low quality videos. The feature bank comprises of a trio of local spatio-temporal features, i.e. shape, motion and textures, which respectively encode structural, dynamic and statistical information in video. These features are encoded into mid-level representations and aggregated to construct STEM.
- Categories:
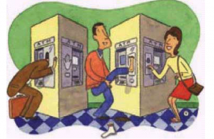
- Read more about Discriminant Correlation Analysis for Feature Level Fusion with Application to Multimodal Biometrics
- Log in to post comments
In this paper, we present Discriminant Correlation Analysis (DCA), a feature level fusion technique that incorporates the class associations in correlation analysis of the feature sets. DCA performs an effective feature fusion by maximizing the pair-wise correlations across the two feature sets, and at the same time, eliminating the between-class correlations and restricting the correlations to be within classes.
- Categories:
- Read more about Dcitionary Learning for Poisson Compressed Sensing
- Log in to post comments
Imaging techniques involve counting of photons striking a detector. Due to fluctuations in the counting process, the measured photon counts are known to be corrupted by Poisson noise. In this paper, we propose a blind dictionary learning framework for the reconstruction of photographic image data from Poisson corrupted measurements acquired by a \emph{compressive} camera.
- Categories:
- Categories:
- Read more about DICTIONARY LEARNING FOR POISSON COMPRESSED SENSING
- Log in to post comments
Imaging techniques involve counting of photons striking a detector.
Due to fluctuations in the counting process, the measured
photon counts are known to be corrupted by Poisson
noise. In this paper, we propose a blind dictionary learning
framework for the reconstruction of photographic image data
from Poisson corrupted measurements acquired by a compressive
camera. We exploit the inherent non-negativity of the
data by modeling the dictionary as well as the sparse dictionary
coefficients as non-negative entities, and infer these directly
- Categories:
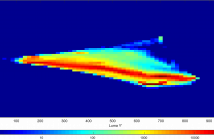
Color pixel encoding optimizes the conversion of linear physical values of light into integer values. The efficiency of such encoding methods depends on a trade-off between the bit-depth used and the visible distortion introduced by quantization. This efficiency for different color pixel encoding approaches has been evaluated in literature, without considering the fact that before transmission to the end-user, color encoded content needs to be compressed using a video codec.
- Categories:
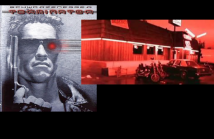
- Categories:
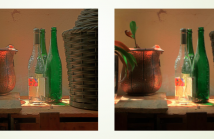
- Read more about Subjective and Objective Quality Assessment of Tone- Mapped Images
- Log in to post comments
1570165825.pdf

- Categories: