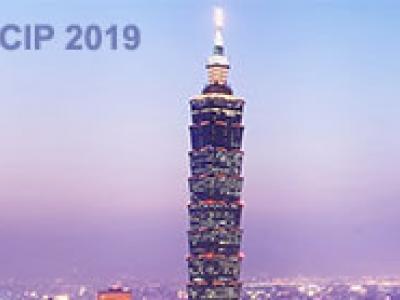
- Read more about Efficient Codebook and Factorization for Second-Order Representation Learning
- Log in to post comments
- Categories:
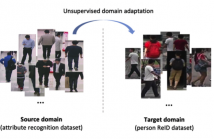
- Read more about UNSUPERVISED DOMAIN-ADAPTIVE PERSON RE-IDENTIFICATION BASED ON ATTRIBUTES
- Log in to post comments
Pedestrian attributes, e.g., hair length, clothes type and color, locally describe the semantic appearance of a person. Training person re-identification (ReID) algorithms under the supervision of such attributes have proven to be effective in extracting local features. Different from person identity, at- tributes are consistent across different domains (or datasets). However, most of ReID datasets lack attribute annotations. On the other hand, there are several datasets labeled with sufficient attributes for the case of pedestrian attribute recognition.
- Categories:
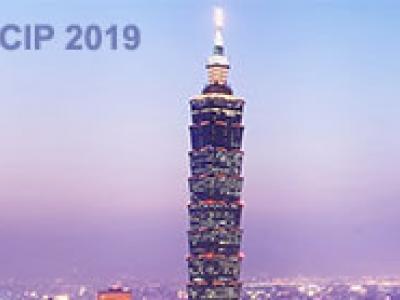
- Read more about Augmented Visual-semantic Embeddings for Image and Sentence Matching
- Log in to post comments
- Categories:
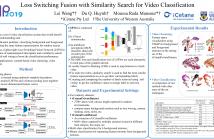
- Read more about Loss Switching Fusion with Similarity Search for Video Classification
- Log in to post comments
From video streaming to security and surveillance applications , video data play an important role in our daily living today. However, managing a large amount of video data and retrieving the most useful information for the user remain a challenging task. In this paper, we propose a novel video classification system that would benefit the scene understanding task. We define our classification problem as classifying background and foreground motions using the same feature representation for outdoor scenes.
- Categories:
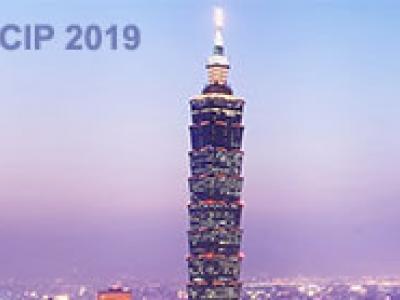
- Read more about poster of "joint image restoration and matching based on hierarchical sparse representation"
- Log in to post comments
- Categories:
- Categories:
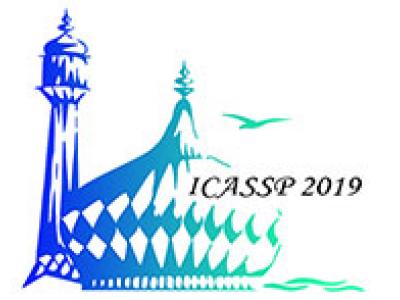
- Categories:
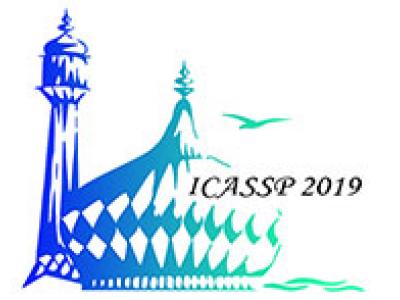
- Read more about Gradient Image Super-Resolution for Low-Resolution Image Recognition
- Log in to post comments
In visual object recognition problems essential to surveillance and navigation problems in a variety of military and civilian use cases,low-resolution and low-quality images present great challenges to this problem. Recent advancements in deep learning based methods like EDSR/VDSR have boosted pixel domain image super-resolution(SR) performances significantly in terms of signal to noise ratio(SNR)/mean square error(MSE) metrics of the super-resolved image.
- Categories:
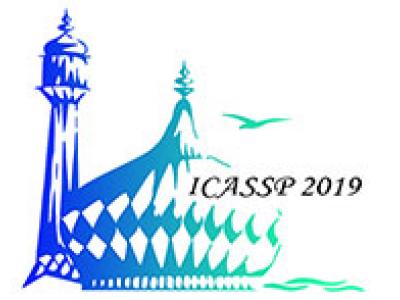
- Read more about CONVOLUTIONAL-SPARSE-CODED DYNAMIC MODE DECOMPOSITION AND ITS APPLICATION TO RIVER STATE ESTIMATION
- Log in to post comments
This work proposes convolutional-sparse-coded dynamic mode decomposition (CSC-DMD) by unifying extended dynamic mode de-
- Categories:
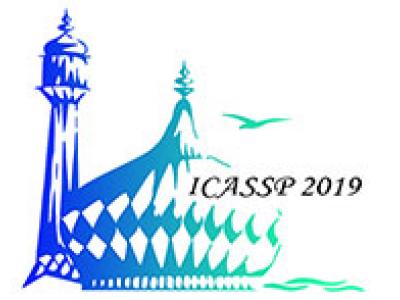
- Read more about Bilinear Representation for Language-based Image Editing Using Conditional Generative Adversarial Networks
- Log in to post comments
The task of Language-Based Image Editing (LBIE) aims at generating a target image by editing the source image based on the given language description. The main challenge of LBIE is to disentangle the semantics in image and text and then combine them to generate realistic images. Therefore, the editing performance is heavily dependent on the learned representation.
- Categories: