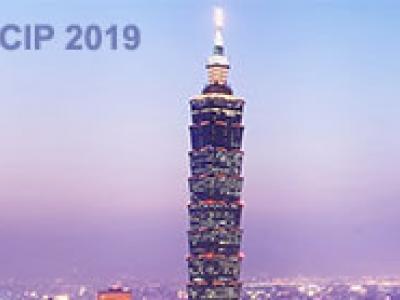
- Read more about Partition Tree Guided Progressive Rethinking Network for in-Loop Filtering of HEVC
- Log in to post comments
In-Loop filter is a key part in High Efficiency Video Coding(HEVC) which effectively removes the compression artifacts.Recently, many newly proposed methods combine residual learning and dense connection to construct a deeper network for better in-loop filtering performance. However,the long-term dependency between blocks is neglected, and information usually passes between blocks only after dimension compression.
- Categories:
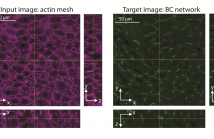
- Read more about PREDICTION OF MULTIPLE 3D TISSUE STRUCTURES BASED ON SINGLE-MARKER IMAGES USING CONVOLUTIONAL NEURAL NETWORKS
- Log in to post comments
A quantitative understanding of complex biological systems such as tissues requires reconstructing the structure of the different components of the system. Fluorescence microscopy provides the means to visualize simultaneously several tissue components. However, it can be time consuming and is limited by the number of fluorescent markers that can be used. In this study, we describe a toolbox of algorithms based on convolutional neural networks for the prediction of 3D tissue structures by learning features embedded within single-marker images.
- Categories:
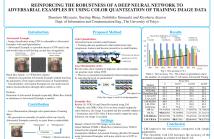
- Read more about REINFORCING THE ROBUSTNESS OF A DEEP NEURAL NETWORK TO ADVERSARIAL EXAMPLES BY USING COLOR QUANTIZATION OF TRAINING IMAGE DATA
- Log in to post comments
Recent works have shown the vulnerability of deep convolu-tional neural network (DCNN) to adversarial examples withmalicious perturbations. In particular, Black-Box attackswithout information of parameter and architectures of thetarget models are feared as realistic threats. To address thisproblem, we propose a method using an ensemble of mod-els trained by color-quantized data with loss maximization.Color-quantization can allow the trained models to focuson learning conspicuous spatial features to enhance the ro-bustness of DCNNs to adversarial examples.
- Categories:
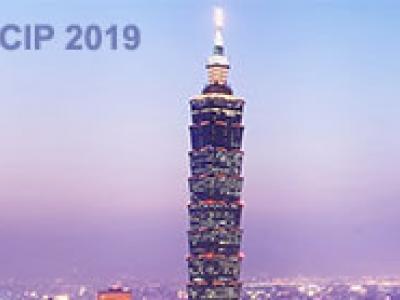
- Read more about Image Pre-Transformation for Recognition-Aware Image Compression
- Log in to post comments
- Categories:
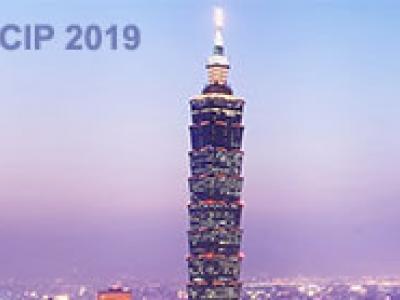
- Read more about Presentation Slides REVE: Regularizing Deep Learning using Variational Entropy Bound
- Log in to post comments
Studies on generalization performance of machine learning algorithms under the scope of information theory suggest that compressed representations can guarantee good generalization, inspiring many compression-based regularization methods. In this paper, we introduce REVE, a new regularization scheme. Noting that compressing the representation can be sub-optimal, our first contribution is to identify a variable that is directly responsible for the final prediction. Our method aims at compressing the class conditioned entropy of this latter variable.
- Categories:
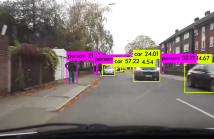
This paper proposed a modified YOLOv3 which has an extra object depth prediction module for obstacle detection and avoidance. We use a pre-processed KITTI dataset to train the proposed, unified model for (i) object detection and (ii) depth prediction and use the AirSim flight simulator to generate synthetic aerial images to verify that our model can be applied in different data domains.
- Categories:
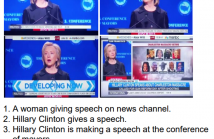
- Categories:
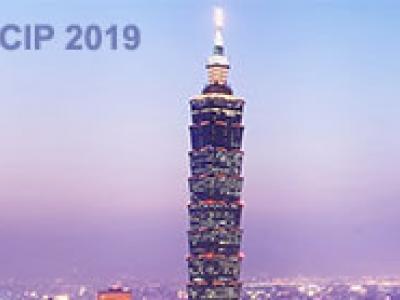
- Read more about A Multi-Task Bayesian Deep Neural Net for Detecting Life-Threatening Infant Incidents From Head Images
- Log in to post comments
The notorious incident of sudden infant death syndrome (SIDS) can easily happen to a newborn due to many environmental factors. To prevent such tragic incidents from happening, we propose a multi-task deep learning framework that detects different facial traits and two life-threatening indicators, i.e. which facial parts are occluded or covered, by analyzing the infant head image. Furthermore, we extend and adapt the recently developed models that capture data-dependent uncertainty from noisy observations for our application.
- Categories:
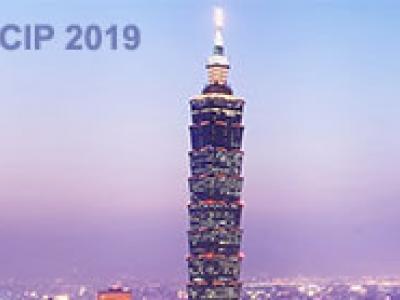
- Read more about Hyperspectral Image Classification with Tensor-Based Rank-R Learning Models
- Log in to post comments
- Categories: