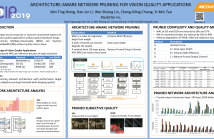
- Read more about ARCHITECTURE-AWARE NETWORK PRUNING FOR VISION QUALITY APPLICATIONS
- Log in to post comments
Convolutional neural network (CNN) delivers impressive achievements in computer vision and machine learning field. However, CNN incurs high computational complexity, especially for vision quality applications because of large image resolution. In this paper, we propose an iterative architecture-aware pruning algorithm with adaptive magnitude threshold while cooperating with quality-metric measurement simultaneously. We show the performance improvement applied on vision quality applications and provide comprehensive analysis with flexible pruning configuration.
- Categories:
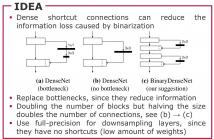
Binary neural networks are a promising approach to execute convolutional neural networks on devices with low computational power. Previous work on this subject often quantizes pretrained full-precision models and uses complex training strategies. In our work, we focus on increasing the performance of binary neural networks by training from scratch with a simple training strategy. In our experiments we show that we are able to achieve state-of-the-art results on standard benchmark datasets.
poster.pdf

- Categories:
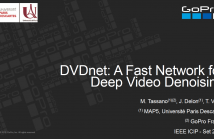
This document includes the slides of the ICIP2019 presentation of the publication "DVDnet: A Fast Network for Deep Video Denoising".
- Categories:
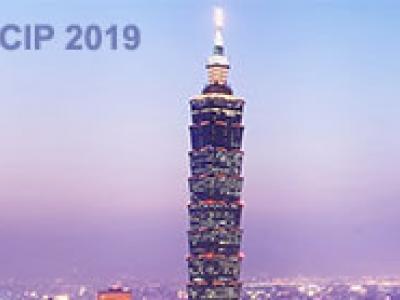
- Read more about EFFICIENT FINE-TUNING OF NEURAL NETWORKS FOR ARTIFACT REMOVAL IN DEEP LEARNING FOR INVERSE IMAGING PROBLEMS
- Log in to post comments
While Deep Neural Networks trained for solving inverse imaging problems (such as super-resolution, denoising, or inpainting tasks) regularly achieve new state-of-the-art restoration performance, this increase in performance is often accompanied with undesired artifacts generated in their solution. These artifacts are usually specific to the type of neural network architecture, training, or test input image used for the inverse imaging problem at hand. In this paper, we propose a fast, efficient post-processing method for reducing these artifacts.
- Categories:
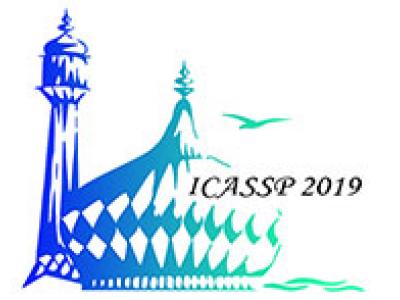
- Read more about Speech Emotion Recognition Using Multi-hop Attention Mechanism
- Log in to post comments
In this paper, we are interested in exploiting textual and acoustic data of an utterance for the speech emotion classification task. The baseline approach models the information from audio and text independently using two deep neural networks (DNNs). The outputs from both the DNNs are then fused for classification. As opposed to using knowledge from both the modalities separately, we propose a framework to exploit acoustic information in tandem with lexical data.
- Categories:
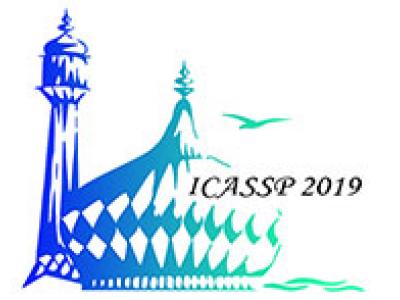
- Read more about Aggregation Graph Neural Networks
- Log in to post comments
Graph neural networks (GNNs) regularize classical neural networks by exploiting the underlying irregular structure supporting graph data, extending its application to broader data domains. The aggregation GNN presented here is a novel GNN that exploits the fact that the data collected at a single node by means of successive local exchanges with neighbors exhibits a regular structure. Thus, regular convolution and regular pooling yield an appropriately regularized GNN.
- Categories:
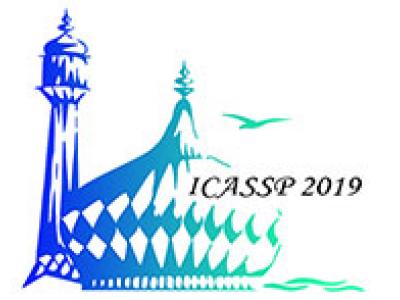
- Read more about Network Adaptation Strategies for Learning New Classes without Forgetting the Original Ones
- Log in to post comments
We address the problem of adding new classes to an existing classifier without hurting the original classes, when no access is allowed to any sample from the original classes. This problem arises frequently since models are often shared without their training data, due to privacy and data ownership concerns. We propose an easy-to-use approach that modifies the original classifier by retraining a suitable subset of layers using a linearly-tuned, knowledge-distillation regularization.
- Categories:
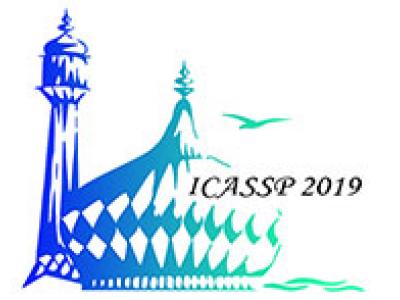
- Read more about Peak Detection and Baseline Correction using a Convolution Neural Network
- Log in to post comments
- Categories:
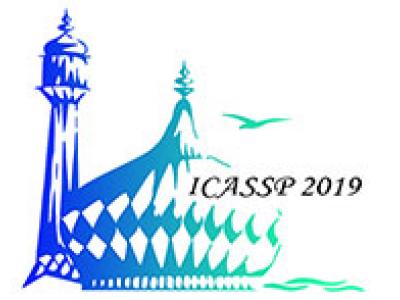
- Read more about Divergence Based Weighting for Information Channels in Deep Convolutional Neural Networks for Bird Audio Detection
- Log in to post comments
In this paper, we address the problem of bird audio detec-
tion and propose a new convolutional neural network archi-
tecture together with a divergence based information channel
weighing strategy in order to achieve improved state-of-the-
art performance and faster convergence. The effectiveness of
the methodology is shown on the Bird Audio Detection Chal-
lenge 2018 (Detection and Classification of Acoustic Scenes
and Events Challenge, Task 3) development data set.
- Categories:
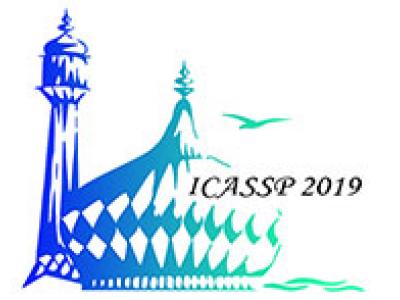
- Read more about SPATIALLY ADAPTIVE LOSSES FOR VIDEO SUPER-RESOLUTION WITH GANS
- Log in to post comments
ICASSP_PPT.pdf

- Categories: