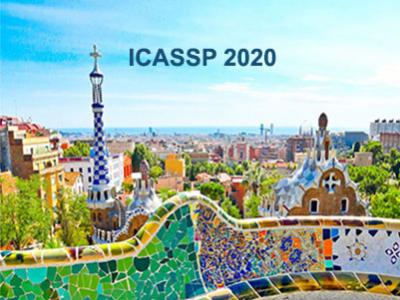
- Read more about TEMPORAL CODING IN SPIKING NEURAL NETWORKS WITH ALPHA SYNAPTIC FUNCTION
- Log in to post comments
- Categories:
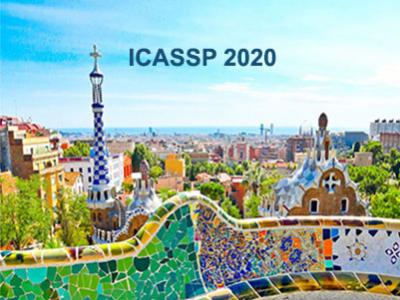
- Read more about An ensemble Based Approach for Generalized Detection of Spoofing Attacks to Automatic Speaker Recognizers
- Log in to post comments
As automatic speaker recognizer systems become mainstream, voice spoofing attacks are on the rise. Common attack strategies include replay, the use of text-to-speech synthesis, and voice conversion systems. While previously-proposed end-to-end detection frameworks have shown to be effective in spotting attacks for one particular spoofing strategy, they have relied on different models, architectures, and speech representations, depending on the spoofing strategy.
- Categories:
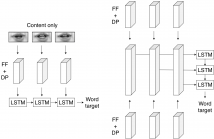
We present a novel lipreading system that improves on the task of speaker-independent word recognition by decoupling motion and content dynamics. We achieve this by implementing a deep learning architecture that uses two distinct pipelines to process motion and content and subsequently merges them, implementing an end-to-end trainable system that performs fusion of independently learned representations. We obtain a average relative word accuracy improvement of ≈6.8% on unseen speakers and of ≈3.3% on known speakers, with respect to a baseline which uses a standard architecture.
- Categories:
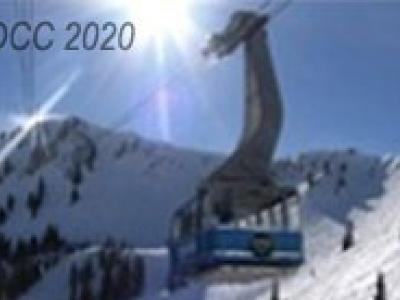
Motivated by the ever-increasing demands for limited communication bandwidth and low-power consumption, we propose a new methodology, named joint Variational Autoencoders with Bernoulli mixture models (VAB), for performing clustering in the compressed data domain. The idea is to reduce the data dimension by Variational Autoencoders (VAEs) and group data representations by Bernoulli mixture models (BMMs).
- Categories:
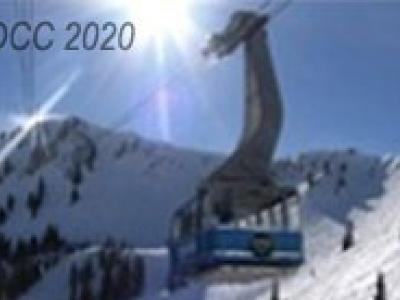
- Read more about Light Field Image Compression Using Multi-Branch Spatial Transformer Networks Based View Synthesis
- Log in to post comments
The recent years have witnessed the widespread of light field imaging in interactive and immersive visual applications. To record the directional information of the light rays, larger storage space is required by light field images compared with conventional 2D images. Hence, the efficient compression of light field image is highly desired for further applications. In this paper, we propose a novel light field image compression scheme using multi- branch spatial transformer networks based view synthesis.
- Categories:
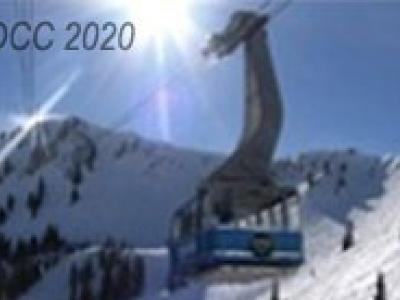
- Read more about Flow-Guided Temporal-Spatial Network for HEVC Compressed Video Quality Enhancement
- Log in to post comments
In this work, a flow-guided temporal-spatial network (FGTSN) is proposed to enhance the quality of HEVC compressed video. Specially, we first employ a motion estimation subnet via trainable optical flow module to estimate the motion flow between current frame and its adjacent frames. Guiding by the predicted motion flow, the adjacent frames are aligned to current frame. Then, a temporal encoder is designed to discover the variations between current frame and its warped frames. Finally, the reconstruction frame is generated by training the model in a multi-supervised fashion.
- Categories:
- Read more about HIGH PERFORMANCE SUPERVISED TIME-DELAY ESTIMATION USING NEURAL NETWORKS
- Log in to post comments
Time-delay estimation is an essential building block of many signal processing applications. This paper follows up on earlier work for acoustic source localization and time delay estimation using pattern recognition techniques; it presents high performance results obtained with supervised training of neural networks which challenge the state of the art and compares its performance to that of well-known methods such as the Generalized Cross-Correlation or Adaptive Eigenvalue Decomposition.
- Categories:
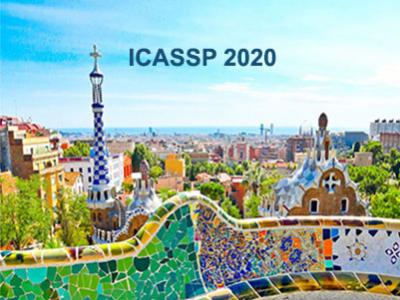
- Read more about Counting dense objects in remote sensing images
- Log in to post comments
Estimating accurate number of interested objects from a given image is a challenging yet important task. Significant efforts have been made to address this problem and achieve great progress, yet counting number of ground objects from remote sensing images is barely studied. In this paper, we are interested in counting dense objects from remote sensing images. Compared with object counting in natural scene, this task is challenging in following factors: large scale variation, complex cluttered background and orientation arbitrariness.
- Categories:
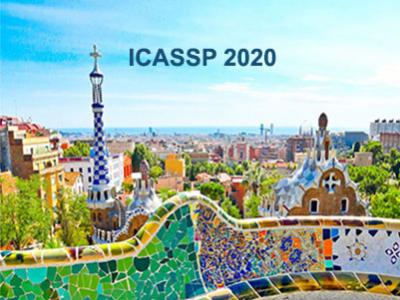
- Read more about Counting dense objects in remote sensing images
- Log in to post comments
Estimating accurate number of interested objects from a given image is a challenging yet important task. Significant efforts have been made to address this problem and achieve great progress, yet counting number of ground objects from remote sensing images is barely studied. In this paper, we are interested in counting dense objects from remote sensing images. Compared with object counting in natural scene, this task is challenging in following factors: large scale variation, complex cluttered background and orientation arbitrariness.
- Categories:
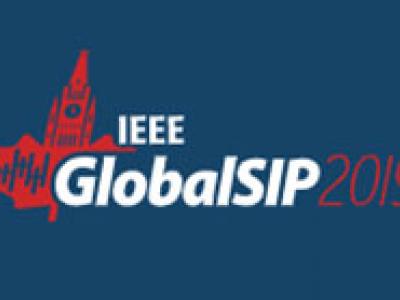
- Read more about Serious Games and ML for Detecting MCI
- Log in to post comments
Our work has focused on detecting Mild Cognitive Impairment (MCI) by developing Serious Games (SG) on mobile devices, distinct from games marketed as 'brain training' which claim to maintain mental acuity. One game, WarCAT, captures players' moves during the game to infer processes of strategy recognition, learning, and memory. The purpose of our game is to use the generated game-play data combined with machine learning (ML) to help detect MCI. MCI is difficult to detect for several reasons.
- Categories: