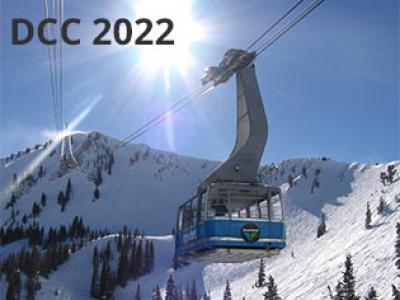
- Read more about Selective Weighted Adaptive Coding
- 1 comment
- Log in to post comments
- Categories:
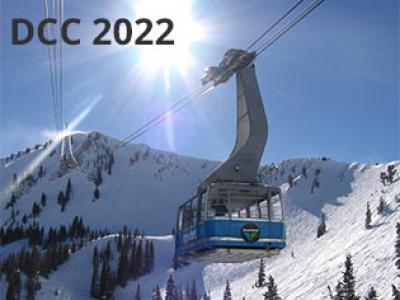
- Read more about A Huffman Code Based Crypto-System
- 1 comment
- Log in to post comments
- Categories:
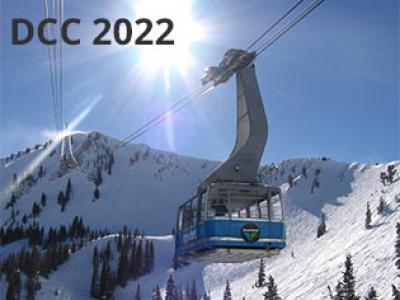
- Read more about FM-Indexing Grammars Induced by Suffix Sorting for Long Patterns
- Log in to post comments
- Categories:
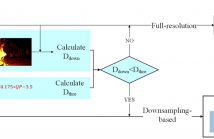
Resampling-based coding, i.e. down-sampling before encoding and up-sampling after decoding, has been recognized to be an effective tool for compressing high-resolution videos at low bitrates. The newest video coding standard, Versatile Video Coding (VVC), supports resampling-based coding via a mechanism named Reference Picture Resampling (RPR), where the spatial resolution can be changed without inserting an intra frame. Intuitively, it is not wise to utilize a single resolution throughout the whole video, because frames with different contents may prefer different coding resolutions.
- Categories:
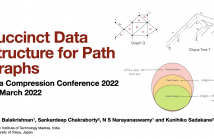
- Read more about Succinct Data Structure for Path Graphs
- Log in to post comments
We consider the problem of designing space-efficient data structures for unlabelled path graphs with n vertices while supporting basic navigational queries such as degree, adjacency, and neighborhood queries efficiently. We provide two solutions for this problem. Our first data structure is succinct and occupies n log n+o(n log n) bits while answering adjacency query in O(log n) time, and neighborhood and degree queries in O(d log^2 n) time where d is the degree of the queried vertex. Our second data structure answers all these queries faster at the expense of slightly more space.
- Categories:
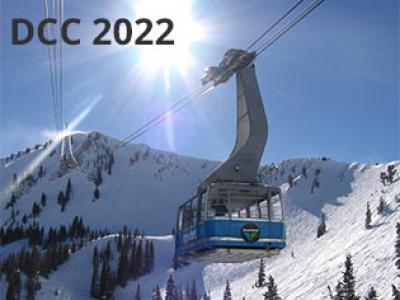
- Read more about Converting RLBWT to LZ77 in smaller space
- 1 comment
- Log in to post comments
RLBWT2LZ77.pdf

- Categories:
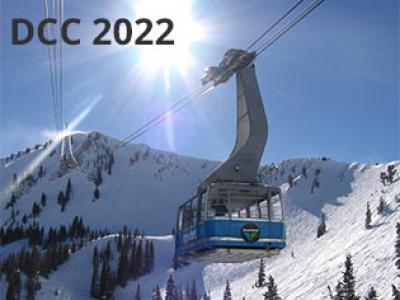
- Read more about Learning Tucker Compression for Deep CNN
- 1 comment
- Log in to post comments
Recently, tensor decomposition approaches are used to compress deep convolutional neural networks (CNN) for getting a faster CNN with fewer parameters. However, there are two problems of tensor decomposition based CNN compression approaches, one is that they usually decompose CNN layer by layer, ignoring the correlation between layers, the other is that training and compressing a CNN is separated, easily leading to local optimum of ranks. In this paper, Learning Tucker Compression (LTC) is proposed.
164-Paper.pdf

- Categories:
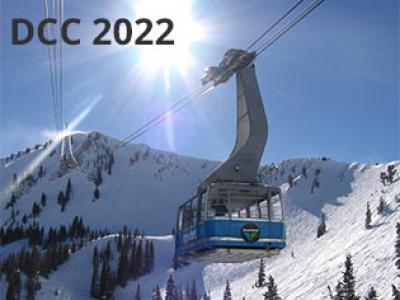
- Read more about Datastructure Lower Bounds for Depth First Search
- Log in to post comments
- Categories:
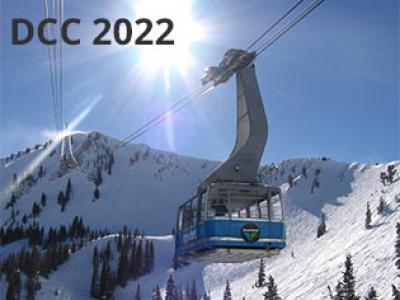
- Categories:
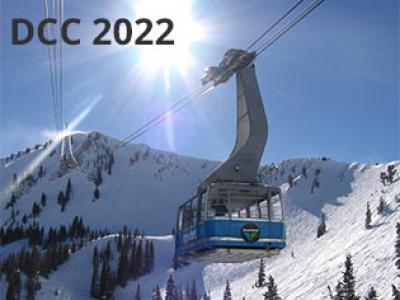
- Read more about Binary-Coded Ternary Number Representation in Natural Language Text Compression
- 3 comments
- Log in to post comments
A lossless data compression code based on a binary-coded ternary number representation is investigated. The code is synchronizable and supports a direct search in a compressed file. A simple monotonous encoding and very fast decoding algorithms are constructed owing to code properties. Experiments show that in natural language text compression the new code outperforms byte-aligned codes SCDC and RPBC either in compression ratio or in decoding speed.
- Categories: