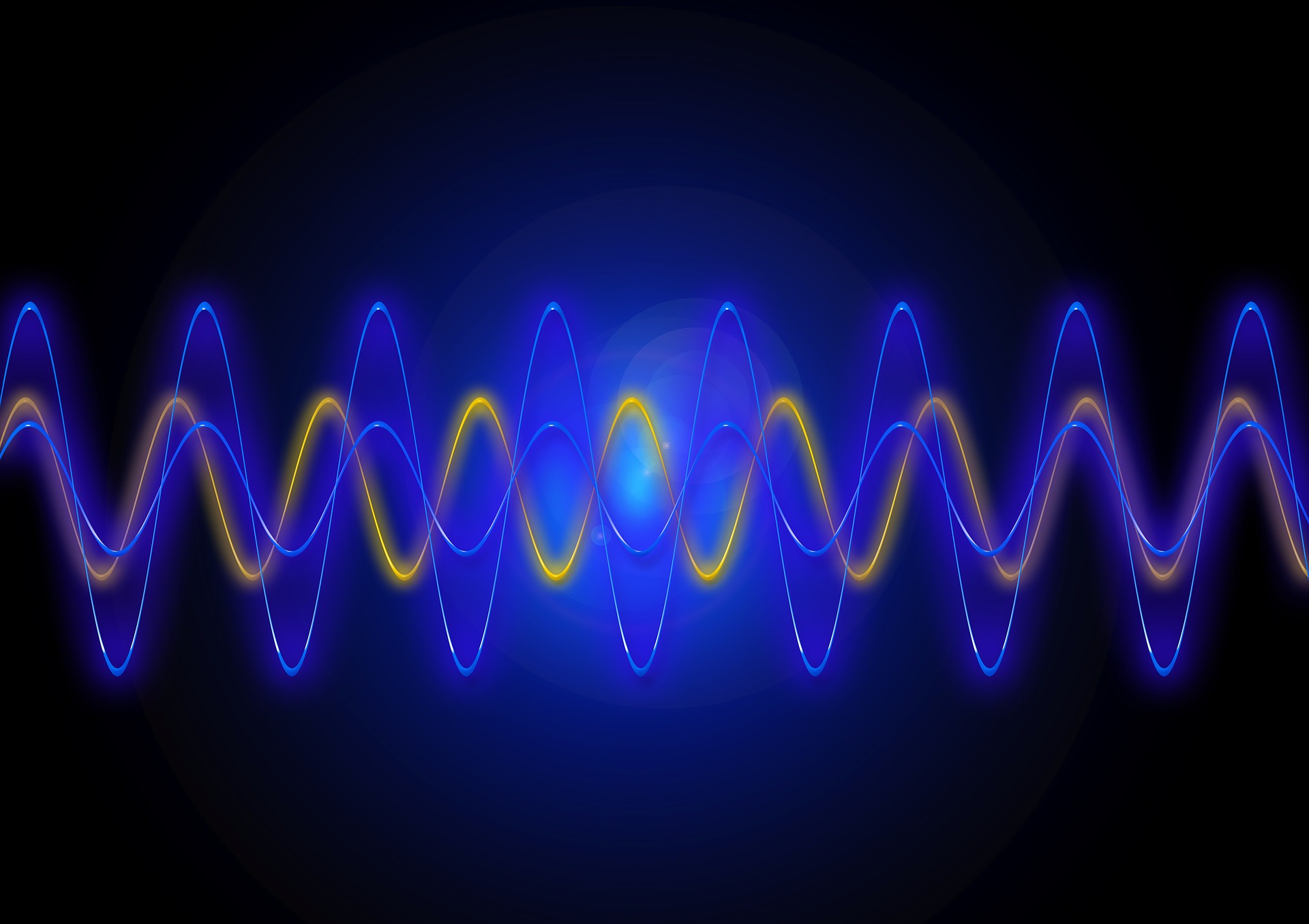
- Read more about Assisted Learning: Cooperative AI with Autonomy
- Log in to post comments
- Categories:
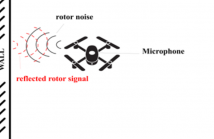
In this paper, we propose a method to estimate the proximity of an acoustic reflector, e.g., a wall, using ego-noise, i.e., the noise produced by the moving parts of a listening robot. This is achieved by estimating the times of arrival of acoustic echoes reflected from the surface. Simulated experiments show that the proposed non-intrusive approach is capable of accurately estimating the distance of a reflector up to 1 meter and outperforms a previously proposed intrusive approach under loud ego-noise conditions.
- Categories:
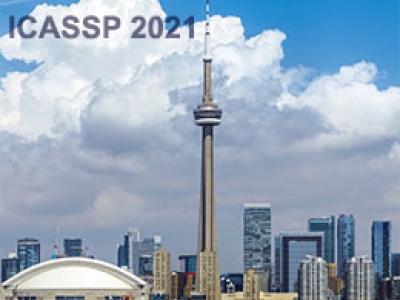
- Read more about A PARALLELIZABLE LATTICE RESCORING STRATEGY WITH NEURAL LANGUAGE MODELS
- Log in to post comments
This paper proposes a parallel computation strategy and a posterior-based lattice expansion algorithm for efficient lattice rescoring with neural language models (LMs) for automatic speech recognition. First, lattices from first-pass decoding are expanded by the proposed posterior-based lattice expansion algorithm. Second, each expanded lattice is converted into a minimal list of hypotheses that covers every arc. Each hypothesis is constrained to be the best path for at least one arc it includes.
- Categories:
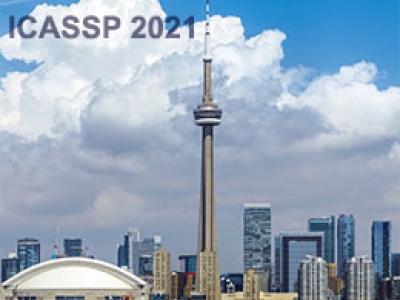
- Read more about Federated Learning With Local Differential Privacy: Trade-Offs Between Privacy, Utility, and Communication
- Log in to post comments
Federated learning (FL) allows to train a massive amount of data privately due to its decentralized structure. Stochastic gradient descent (SGD) is commonly used for FL due to its good empirical performance, but sensitive user information can still be inferred from weight updates shared during FL iterations. We consider Gaussian mechanisms to preserve local differential privacy (LDP) of user data in the FL model with SGD. The trade-offs between user privacy, global utility, and transmission rate are proved by defining appropriate metrics for FL with LDP.
- Categories:
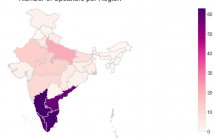
- Read more about NISP: A Multi-lingual Multi-accent Dataset for Speaker Profiling
- Log in to post comments
Many commercial and forensic applications of speech demand the extraction of information about the speaker characteristics, which falls into the broad category of speaker profiling. The speaker characteristics needed for profiling include physical traits of the speaker like height, age, and gender of the speaker along with the native language of the speaker. Many of the datasets available have only partial information for speaker profiling.
- Categories:
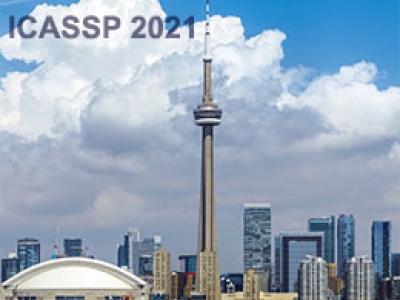
- Read more about MULTIVIEW SENSING WITH UNKNOWN PERMUTATIONS: AN OPTIMAL TRANSPORT APPROACH
- Log in to post comments
In several applications, including imaging of deformable objects while in motion, simultaneous localization and mapping, and unlabeled sensing, we encounter the problem of recovering a signal that is measured subject to unknown permutations. In this paper we take a fresh look at this problem through the lens of optimal transport (OT). In particular, we recognize that in most practical applications the unknown permutations are not arbitrary but some are more likely to occur than others.
- Categories:
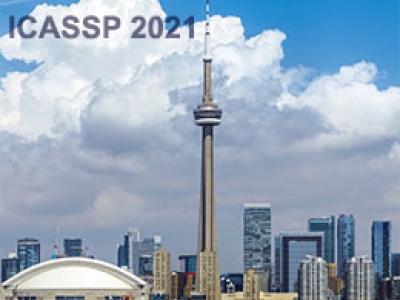
- Read more about Moving object classification with a sub-6 GHz massive MIMO array using real data
- Log in to post comments
- Categories:
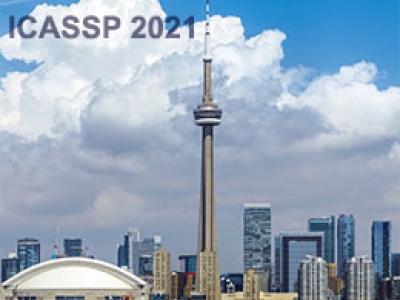
- Read more about MORE:A Metric-learning based Framework for Open-domain Relation Extraction
- Log in to post comments
Open relation extraction (OpenRE) is the task of extracting relation schemes from open-domain corpora. Most existing OpenRE methods either do not fully benefit from high-quality labeled corpora or can not learn semantic representation directly, affecting downstream clustering efficiency. To address these problems, in this work, we propose a novel learning framework named MORE (Metric learning-based Open Relation Extraction.
- Categories:
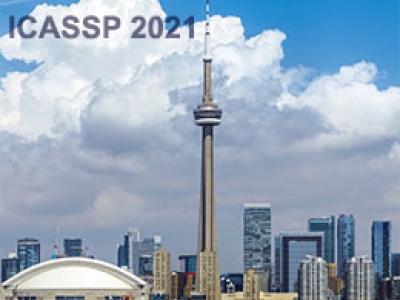
During the COVID-19 pandemic the health authorities at airports and train stations try to screen and identify the travellers possibly exposed to the virus. However, many individuals avoid getting tested and hence may misreport their travel history. This is a challenge for the health authorities who wish to ascertain the truly susceptible cases in spite of this strategic misreporting. We investigate the problem of questioning travellers to classify them for further testing when the travellers are strategic or are unwilling to reveal their travel histories.
- Categories:
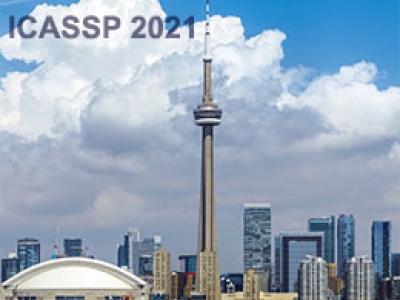
During the COVID-19 pandemic the health authorities at airports and train stations try to screen and identify the travellers possibly exposed to the virus. However, many individuals avoid getting tested and hence may misreport their travel history. This is a challenge for the health authorities who wish to ascertain the truly susceptible cases in spite of this strategic misreporting. We investigate the problem of questioning travellers to classify them for further testing when the travellers are strategic or are unwilling to reveal their travel histories.
- Categories: