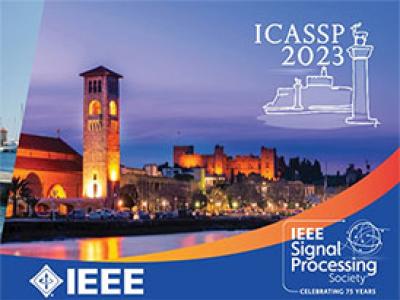
- Read more about RECOGNIZING HIGHLY VARIABLE AMERICAN SIGN LANGUAGE IN VIRTUAL REALITY
- Log in to post comments
Recognizing signs in virtual reality (VR) is challenging; here, we developed an American Sign Language (ASL) recognition system in a VR environment. We collected a dataset of 2,500 ASL numerical digits (0-10) and 500 instances of the ASL sign for TEA from 10 participants using an Oculus Quest 2. Participants produced ASL signs naturally, resulting in significant variability in location, orientation, duration, and motion trajectory. Additionally, the ten signers in this initial study were diverse in age, sex, ASL proficiency, and hearing status, with most being deaf lifelong ASL users.
- Categories:
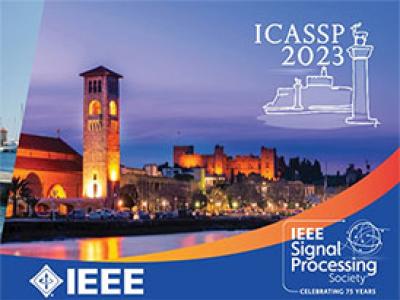
- Read more about F0 ESTIMATION FROM TELEPHONE SPEECH USING DEEP FEATURE LOSS
- 1 comment
- Log in to post comments
Accurate pitch estimation in speech signal plays a vital role in several applications. Robust pitch estimation in telephone speech is still a challenge due to the narrow bandwidth of the signal. Electroglottograph (EGG) signal is a reliable means for pitch estimation, however, it’s not practically possible to
- Categories:
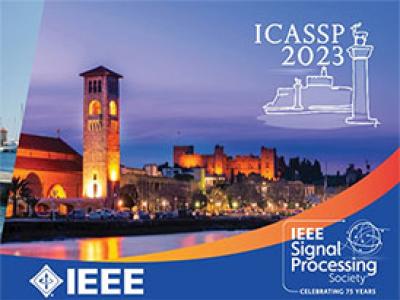
- Categories:
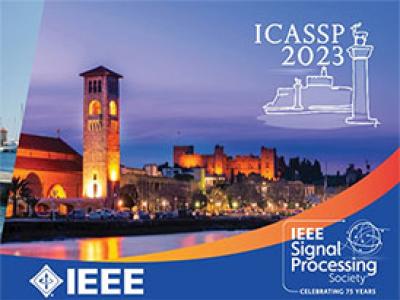
- Read more about Residual Squeeze-and-Excitation U-shaped Network for Minutia Extraction in Contactless Fingerprint Images
- Log in to post comments
Slides.pdf

- Categories:
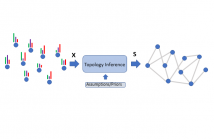
- Read more about Network Topology Inference from Gaussian and Stationary Graph Signals
- Log in to post comments
Graphs have become pervasive tools to represent information and datasets with irregular support. However, in many cases, the underlying graph is either unavailable or naively obtained, calling for more advanced methods for its estimation. Indeed, graph topology inference methods that estimate the network structure from a set of signal observations have a long and well-established history. By assuming that the observations are both Gaussian and stationary in the sought graph, this paper proposes a new scheme to learn the network from nodal observations.
- Categories:
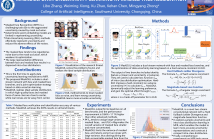
- Read more about MaskDUL: Data Uncertainty Learning in Masked Face Recognition
- Log in to post comments
Since mask occlusion causes plentiful loss of facial feature, Masked Face Recognition (MFR) is a challenging image processing task, and the recognition results are susceptible to noise. However, existing MFR methods are mostly deterministic point embedding models, which are limited in representing noise images. Moreover, Data Uncertainty Learning (DUL) fails to achieve reasonable performance in MFR.
Poster.pdf

- Categories:
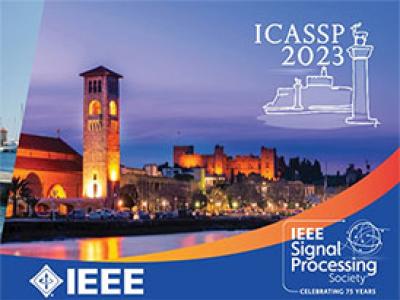
- Read more about Delay-aware Backpressure Routing Using Graph Neural Networks
- Log in to post comments
We propose a throughput-optimal biased backpressure (BP) algorithm for routing, where the bias is learned through a graph neural network that seeks to minimize end-to-end delay. Classical BP routing provides a simple yet powerful distributed solution for resource allocation in wireless multi-hop networks but has poor delay performance. A low-cost approach to improve this delay performance is to favor shorter paths by incorporating pre-defined biases in the BP computation, such as a bias based on the shortest path (hop) distance to the destination.
- Categories:
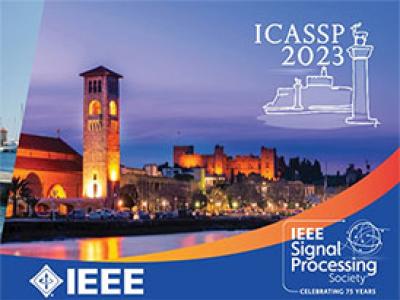
This paper investigates negative sampling for contrastive learning in the context of audio-text retrieval. The strategy for negative sampling refers to selecting negatives (either audio clips or textual descriptions) from a pool of candidates for a positive audio-text pair. We explore sampling strategies via model-estimated within-modality and cross-modality relevance scores for audio and text samples. With a constant training setting on the retrieval system from [1], we study eight sampling strategies, including hard and semi-hard negative sampling.
- Categories:
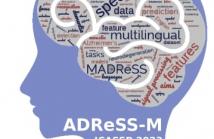
- Read more about Cross-Lingual Transfer Learning for Alzheimer’s Detection from Spontaneous Speech
- Log in to post comments
Alzheimer’s disease (AD) is a progressive neurodegenerative disease most often associated with memory deficits and cognitive decline. With the aging population, there has been much interest in automated methods for cognitive impairment detection. One approach that has attracted attention in recent years is AD detection through spontaneous speech. While the results are promising, it is not certain whether the learned speech features can be generalized across languages. To fill this gap, the ADReSS-M challenge was organized.
- Categories: