- Read more about DA-VLAD: DISCRIMINATIVE ACTION VECTOR OF LOCALLY AGGREGATED DESCRIPTORS FOR ACTION RECOGNITION
- Log in to post comments
In this paper, we propose a novel encoding method for the representation of human action videos, that we call Discriminative Action Vector of Locally Aggregated Descriptors (DA-VLAD). DA-VLAD is motivated by the fact that there are many unnecessary and overlapping frames that cause non-discriminative codewords during the training process. DA-VLAD deals with this issue by extracting class-specific clusters and learning the discriminative power of these codewords in the form of informative weights.
- Categories:
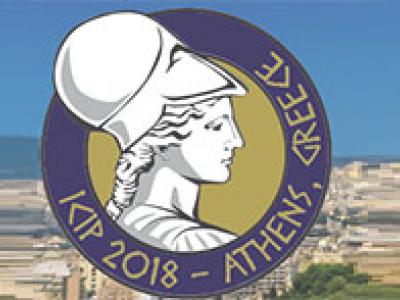
- Read more about GRANULOMETRY-BASED DESCRIPTOR FOR PATHOLOGICAL TISSUE DISCRIMINATION IN HISTOPATHOLOGICAL IMAGES
- Log in to post comments
Prostate cancer is one of the types of cancer with the highest incidence in humans. In particular, prostate cancer is the main cause of death from cancer in men over 70 years of age. The automatic analysis of histological images is nowadays a key factor for helping doctors in the diagnosis task. In this paper, we present granulometries as a novel image descriptor to identify abnormal patterns in the prostatic tissue. The morphological alteration suffered by the main structures of pathological glands are registered by the proposed descriptor and achieved in a feature vector.
- Categories:
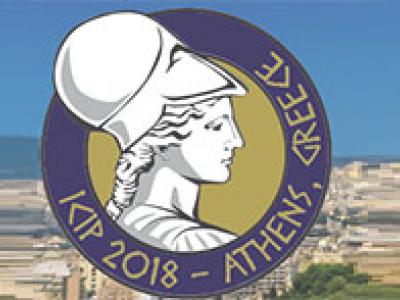
- Read more about KEY JOINTS SELECTION AND SPATIOTEMPORAL MINING FOR SKELETON-BASEDACTION RECOGNITION
- Log in to post comments
- Categories:
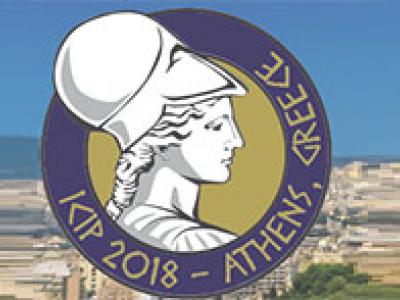
- Read more about GRAPH-BASED EARLY-FUSION FOR FLOOD DETECTION
- Log in to post comments
Flooding is one of the most harmful natural disasters, as it poses danger to both buildings and human lives. Therefore, it is fundamental to monitor these disasters to define prevention strategies and help authorities in damage control. With the wide use of portable devices (e.g., smartphones), there is an increase of the documentation and communication of flood events in social media. However, the use of these data in monitoring systems is not straightforward and depends on the creation of effective recognition strategies.
- Categories:
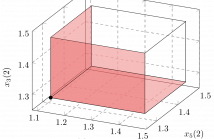
- Read more about Analysis of the Viterbi Algorithm Using Tropical Algebra and Geometry
- Log in to post comments
The Viterbi algorithm and its pruning variant, are some of the most frequently used algorithms in communications and speech recognition. There has been extended research on improving the algorithms’ computational complexity, however work trying to interpret their nonlinear structure and geometry has been limited. In this work we analyse the Viterbi algorithm in the field of tropical (min-plus) algebra, and we utilize its pruning variant in order to define a polytope. Then, we interpret certain faces of the polytope as the most probable states of the algorithm.
- Categories:
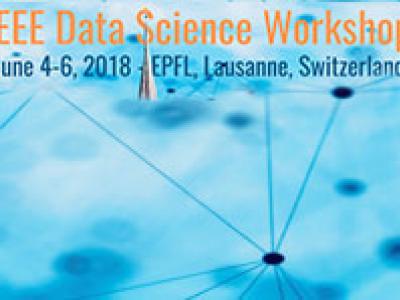
We consider the problem of predicting power outages in an electrical power grid due to hazards produced by convective storms. These storms produce extreme weather phenomena such as intense wind, tornadoes and lightning over a small area. In this paper, we discuss the application of state-of-the-art machine learning techniques, such as random forest classifiers and deep neural networks, to predict the amount of damage caused by storms.
- Categories:
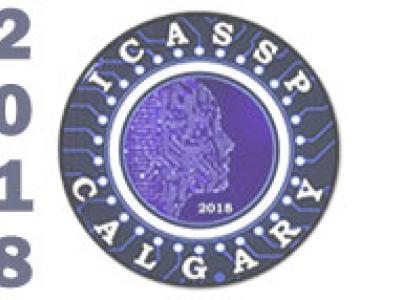
- Categories:
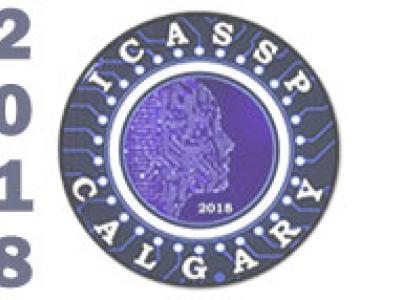
- Categories:
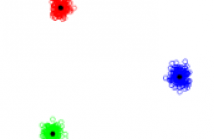
- Read more about Clustering of data with missing entries
- Log in to post comments
The analysis of large datasets is often complicated by the presence of missing entries, mainly because most of the current machine learning algorithms are designed to work with full data. The main focus of this work is to introduce a clustering
- Categories:
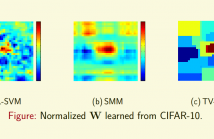
- Read more about TV-SVM: Support Vector Machine with Total Variational Regularization
- Log in to post comments
To leverage the spatial relationship of lattice data, such as images, we introduce total variational (TV) regularization into support vector machines (SVM), called TV-SVM. TV-SVM encourages local smoothness and sparsity in gradient domain of the learned parameters. TV-SVM is optimized via the alternating direction method of multipliers (ADMM) algorithm and is significantly better than (Linear) SVM for image classifications.
- Categories: