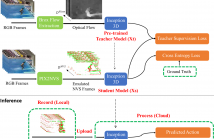
- Read more about NEUROMORPHIC VISION SENSING FOR CNN-BASED ACTION RECOGNITION
- Log in to post comments
Neuromorphic vision sensing (NVS) hardware is now gaining traction as a low-power/high-speed visual sensing technology that circumvents the limitations of conventional active pixel sensing (APS) cameras. While object detection and tracking models have been investigated in conjunction with NVS, there is currently little work on NVS for higher-level semantic tasks, such as action recognition.
- Categories:
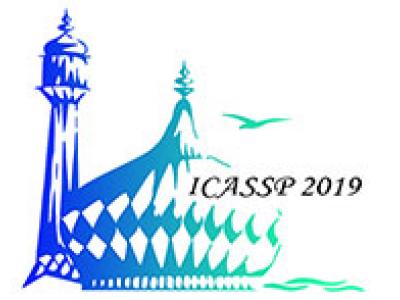
- Read more about Feature Selection for Multi-labeled Variables via Dependency Maximization
- Log in to post comments
Feature selection and reducing the dimensionality of data is an essential step in data analysis. In this work, we propose a new criterion for feature selection that is formulated as conditional information between features given the labeled variable. Instead of using the standard mutual information measure based on Kullback-Leibler divergence, we use our proposed criterion to filter out redundant features for the purpose of multiclass classification.
- Categories:
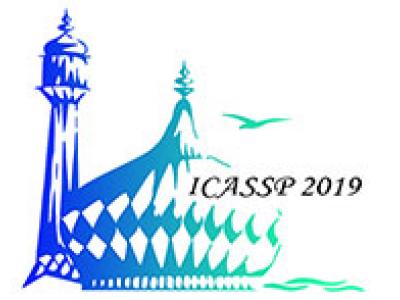
- Read more about AUTOMATIC RADAR-BASED GESTURE DETECTION VIA REGION-BASED DCNN
- Log in to post comments
In this paper, a region-based deep convolutional neural network
(R-DCNN) is proposed to detect and classify gestures
measured by a frequency-modulated continuous wave radar
system. Micro-Doppler (μD) signatures of gestures are exploited,
and the resulting spectrograms are fed into a neural
network. We are the first to use the R-DCNN for radar-based
gesture recognition, such that multiple gestures could be automatically
detected and classified without manually clipping
the data streams according to each hand movement in advance.
- Categories:
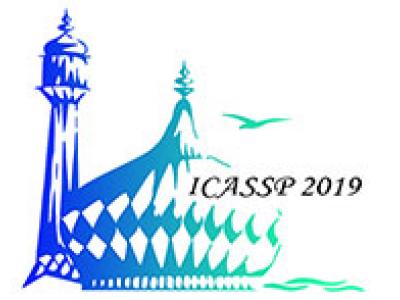
- Read more about Recurrent Neural Networks with Stochastic Layers for Acoustic Novelty Detection
- Log in to post comments
In this paper, we adapt Recurrent Neural Networks with Stochastic Layers, which are the state-of-the-art for generating text, music and speech, to the problem of acoustic novelty detection. By integrating uncertainty into the hidden states, this type of network is able to learn the distribution of complex sequences. Because the learned distribution can be calculated explicitly in terms of probability, we can evaluate how likely an observation is then detect low-probability events as novel.
- Categories:
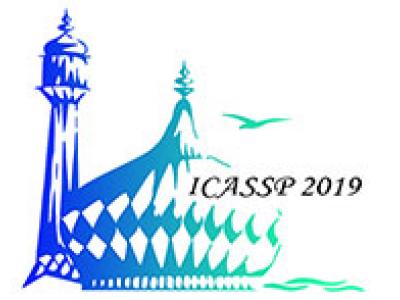
- Read more about Learning Motion Disfluencies for Automatic Sign Language Segmentation
- Log in to post comments
We introduce a novel technique for the automatic detection of word boundaries within continuous sentence expressions in Japanese Sign Language from three-dimensional body joint positions. First, the flow of signed sentence data within a temporal neighborhood is determined utilizing the spatial correlations between line segments of inter-joint pairs. Next, a frame-wise binary random forest classifier is trained to distinguish word and non-word frame content based on the extracted spatio-temporal features.
Poster.pdf

- Categories:
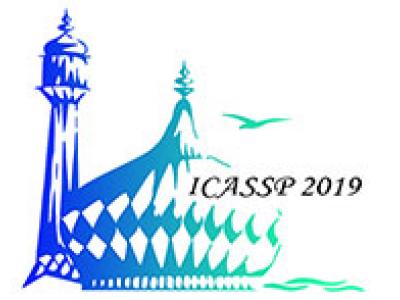
- Read more about A LARGE SCALE ANALYSIS OF LOGISTIC REGRESSION: ASYMPTOTIC PERFORMANCE AND NEW INSIGHTS
- Log in to post comments
- Categories:
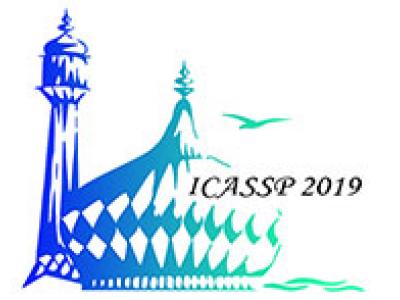
- Read more about Analysis Dictionary Learning: An Efficient and Discriminative Solution
- Log in to post comments
- Categories:
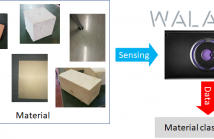
- Read more about MATERIAL IDENTIFICATION USING RF SENSORS AND CONVOLUTIONAL NEURAL NETWORKS
- Log in to post comments
Recent years have assisted a widespreading of Radio-Frequency-based tracking and mapping algorithms for a wide range of applications, ranging from environment surveillance to human-computer interface.
poster.pdf

- Categories:
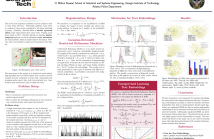
We present a novel event embedding algorithm for crime data that can jointly capture time, location, and the complex free-text component of each event. The embedding is achieved by regularized Restricted Boltzmann Machines (RBMs), and we introduce a new way to regularize by imposing a ℓ1 penalty on the conditional distributions of the observed variables of RBMs. This choice of regularization performs feature selection and it also leads to efficient computation since the gradient can be computed in a closed form.
- Categories:
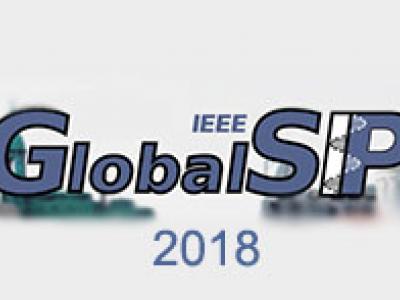
- Read more about Reconstruction-free deep convolutional neural networks for partially observed images
- Log in to post comments
Conventional image discrimination tasks are performed on fully observed images. In challenging real imaging scenarios, where sensing systems are energy demanding or need to operate with limited bandwidth and exposure-time budgets, or defective pixels, where the data collected often suffers from missing information, and this makes the task extremely hard. In this paper, we leverage Convolutional Neural Networks (CNNs) to extract information from partially observed images.
- Categories: